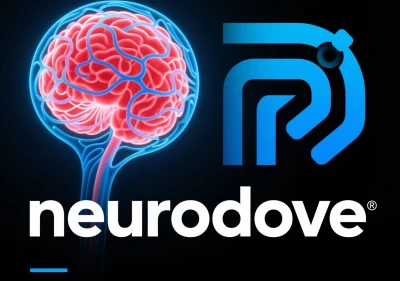
1. Terminology and Core Concepts
Neurodecode is a compound term with two primary meanings:
- Neural Decoding (General Concept):
The process of extracting information related to external stimuli, behaviors, or cognitive states from neural activity signals (e.g., EEG, fMRI, single-neuron recordings). It involves mapping neural responses (e.g., action potentials, hemodynamic signals) to behavioral covariates (e.g., visual stimuli, movement intent), forming a core technique in brain-computer interfaces (BCIs) and computational neuroscience. - Software Tools/Frameworks:
- Neurodecode Framework: An open-source platform for building real-time BCI systems, supporting EEG signal processing, online decoding, and feedback control.
- NeuroDecodeR: An R-based toolkit for neural decoding analysis, offering modular machine learning interfaces for model training and validation.
2. Technical Principles and Workflow
Neurodecode implementation typically follows these steps:
2.1 Data Acquisition and Preprocessing
- Signal Types: EEG, ECoG, fMRI, single-unit recordings.
- Preprocessing: Noise reduction (e.g., ICA for ocular artifact removal), time-frequency analysis (wavelet transforms), feature extraction (e.g., P300 amplitude/latency).
2.2 Decoding Model Development
- Machine Learning Methods:
- Supervised Learning: Algorithms like LDA, SVM, and LSTMs for classifier/regressor training.
- Unsupervised Learning: Clustering (K-means) or dimensionality reduction (PCA, t-SNE) to explore neural patterns.
- Real-Time Decoding: Low-latency requirements (e.g., <62ms feedback loops in EEG-BCI systems).
2.3 Validation and Optimization
- Cross-Validation: Leave-One-Out or time-series splits to assess model generalization.
- Metrics: Accuracy, F1-score, mutual information, or reconstruction error (e.g., SSIM for fMRI).
3. Functional Features of Neurodecode Tools
3.1 Neurodecode Framework
- Modular Design:
- Stream Processing: Real-time signal acquisition via Lab Streaming Layer (LSL).
- Online Decoding Pipeline: Integrates filtering, feature extraction, model inference, and feedback generation.
- Applications:
- Speech Imagery BCI: Decoding EEG patterns for communication aids in speech-impaired users.
- Neurofeedback Training: Real-time brain state monitoring for ADHD therapy or cognitive enhancement.
3.2 NeuroDecodeR
- Core Features:
- Data Standardization: Supports formats like NIX and NWB.
- Extensible Algorithm Library: Prebuilt classifiers (LDA, SVM, random forests) and custom model integration.
- Advantages:
- Cross-Platform Compatibility: Seamless integration with R’s statistical tools (e.g., linear mixed models) and visualization (ggplot2).
- Open-Source Reproducibility: Transparent codebase for collaborative research.
4. Applications and Case Studies
4.1 Medical Rehabilitation
- Motor Function Restoration: Decoding motor cortex signals to control exoskeletons or prosthetics (e.g., Össur’s mind-controlled bionic legs).
- Language Assistance: EEG-based speech synthesis for communication disorders.
4.2 Cognitive Science Research
- Neural Representation Analysis: fMRI decoding of brain responses to complex stimuli (e.g., faces, natural scenes).
- Memory and Decision-Making: Hippocampal activity decoding for spatial memory studies.
4.3 Industrial and Human-Machine Interaction
- Neuroergonomics: Monitoring operator attention/fatigue to optimize workflows (e.g., pilot training).
- VR Integration: Enhancing metaverse immersion via haptic feedback and motion intent decoding (e.g., Meta’s haptic gloves).
5. Challenges and Future Directions
5.1 Technical Hurdles
- Signal Noise and Individual Variability: EEG susceptibility to EMG artifacts; user-specific calibration needs.
- Real-Time Computational Demands: High-density neural data (e.g., 256-channel ECoG) strains embedded systems.
5.2 Emerging Innovations
- Multimodal Fusion: Combining EEG, fNIRS, and eye-tracking for robust decoding.
- Adaptive Online Learning: Integrating transfer and incremental learning to reduce BCI training time.
- Brain-Cloud Interfaces: 6G-enabled remote neural decoding for collaborative control.
6. Conclusion
Neurodecode serves as both a foundational neural decoding technique and a critical toolchain for BCI development. Its broad scope spans signal processing to machine learning, while specialized tools like NeuroDecodeR and the Neurodecode Framework democratize access to advanced applications in healthcare, research, and industry. With advancements in neural hardware and AI, Neurodecode is poised to revolutionize personalized medicine, cognitive augmentation, and immersive technologies.
If you are interested in purchasing this domain, please contact us via email: chuanchuan810@gmail.com