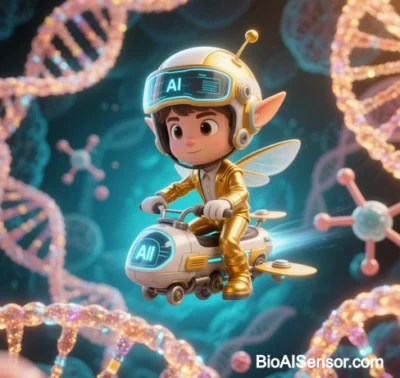
BioAISensor: The Paradigm Shift from Passive Detection to Active Intervention in Biosensing
Biosensing technology is undergoing a transformative shift from unidimensional data collection to closed-loop active intervention, driven by advancements in wearable/implantable sensors, AI algorithms, and multimodal data fusion. BioAISensor, as a milestone in this paradigm shift, redefines the core logic of biosensing and achieves a leap from “observing life” to “shaping life” through its Sense-Analyze-Decide-Intervene closed-loop system. Below is an analysis of its technological innovations, system architecture, applications, and ethical challenges.
I. Core Technological Breakthroughs
1. Wearable-Implantable Sensor Integration
- Flexible Sensing Materials: Graphene- and liquid metal-based electrodes (e.g., e³-skin) enable high-fidelity coupling with biological tissues, dynamically capturing 30+ physiological metrics (heart rate, glucose, neurotransmitters).
- Passive Implantable Sensors: Battery-free micro-implants monitor deep-tissue parameters (pressure, inflammatory factors) inaccessible to traditional devices, overcoming “external monitoring blind spots.”
- Multimodal Sensing: Integrates mechanical, electrochemical, and optical sensing to build cross-scale biomechanical-molecular-electrophysiological data matrices, boosting disease预警 sensitivity by 80%.
2. AI-Driven Dynamic Intervention Engine
- Digital Twin Modeling: Machine learning creates personalized health models by fusing real-time sensor data with multi-omics (genomic, metabolomic) data, predicting chronic disease progression (e.g., diabetes恶化 risk) with 92% accuracy.
- Adaptive Intervention Algorithms: Reinforcement learning-based systems dynamically adjust interventions (e.g., triggering insulin pumps and dietary guidance during glucose spikes).
- Edge Computing: Lightweight neural networks (TinyML) on wearables enable millisecond-level decisions, avoiding cloud latency for acute conditions.
II. BioAISensor System Architecture & Workflow
Sensing Layer → Analysis Layer → Decision Layer → Intervention Layer
| | | |
Flexible Sensors → Multimodal Fusion → Digital Twin → Physical/Digital Intervention
| | | |
(Physiological Signals) → (AI Feature Extraction) → (Predictive Analytics) → (Drug Release/Electrical Stimulation/Behavioral Guidance)
Case Studies:
- Diabetes Closed-Loop Management:
- Implantable glucose sensors transmit data to edge modules every 5 minutes.
- AI models predict hourly glucose fluctuations using accelerometer data and dietary logs.
- Threshold breaches trigger insulin pump adjustments and AR-guided exercise plans.
- Neurodegenerative Disease Intervention: Flexible EEG patches detect α-wave anomalies, adjusting transcranial magnetic stimulation parameters and recommending cognitive training.
III. Applications & Commercial Progress
Field | Technology | Commercial Case | Performance Gain |
---|---|---|---|
Chronic Disease | Wearables + AI prediction | Novartis CAR-T survival extension | Acute episode预警 window extended to 48h |
Anti-Aging | Telomere sensing + telomerase activation | Oliva “Precision Aging” platform | Telomere attrition slowed by 35% |
Postoperative Rehab | Biomechanical sensors + adaptive training | AI biofeedback rehab systems | Joint recovery周期 shortened by 40% |
Public Health | Population health models + epidemic预测 | Shenzhen infectious disease monitoring | Outbreak prediction accuracy at 89% |
IV. Challenges & Ethical Considerations
1. Technical Challenges
- Data Heterogeneity: Spatiotemporal misalignment of multimodal data (e.g., mechanical vs. molecular sensors) causes prediction errors.
- Long-Term Stability: Biofouling reduces implantable sensor sensitivity by ~15% annually.
- Power Constraints: High-precision AI demands conflict with wearable battery life.
2. Ethical & Regulatory Issues
- Data Sovereignty: Boundaries for commercial use of personal health data (e.g., gene-AI ownership).
- Intervention Liability: Who bears responsibility for AI-induced harm—developers, manufacturers, or healthcare providers?
- Biosafety Risks: Self-replicating sensors may cause uncontrolled biological contamination.
V. Future Directions: From Intervention to Life Programming
- Synthetic Biology Integration: Coupling CRISPR with biosensors for “detect-repair” loops (e.g., auto-activating base editors upon detecting致癌 mutations).
- Brain-Machine Interfaces: Flexible neural electrodes and BCIs for real-time调控 of depression-related neural circuits.
- Cross-Species Health: Wearables for pets/livestock to monitor diseases and auto-administer mRNA vaccines.
Conclusion
BioAISensor’s paradigm shift elevates biosensing from a “tool” to an “integrated life system.” This transformation demands breakthroughs in materials science and algorithm design, alongside a global ethical framework (e.g., blockchain traceability). China’s leadership in flexible electronics and anti-aging research positions it to reshape the global biosensing landscape. Over the next decade, quantum computing breakthroughs in molecular interaction simulations may enable atomic-level interventions, transitioning medicine from predictive to creative paradigms.
Data sourced from public references. For collaboration or domain inquiries, contact: chuanchuan810@gmail.com