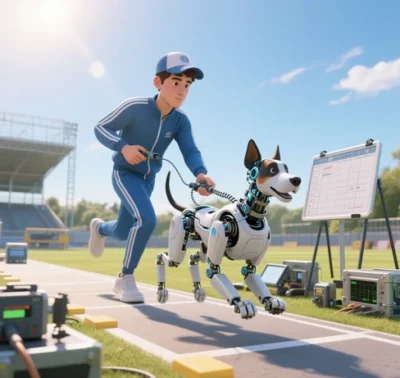
Evobionic: Evolutionary-Driven Bionic Innovation and Case Studies
I. Core Principles of Evobionic Technology
Evobionic, an interdisciplinary frontier integrating synthetic biology, evolutionary computation, and bionic engineering, focuses on optimizing bionic system design by mimicking natural evolutionary mechanisms (e.g., mutation, selection, adaptive radiation) and leveraging functional modules from biological evolutionary history (e.g., quorum sensing, adaptive materials, locomotion patterns). Its framework comprises three pillars:
- Evolutionary Algorithm-Driven Design: Genetic programming and swarm intelligence algorithms simulate natural selection to enhance bionic structures’ mechanical performance and adaptability (e.g., robotic locomotion, material topology).
- Reverse Engineering of Evolutionary History: Genomic/proteomic evolutionary analysis identifies evolutionary pathways of biological modules (e.g., archaeal origins of CRISPR-Cas systems) for bionic applications.
- Dynamic Environmental Adaptation Modeling: Embedding evolutionary pressures (e.g., temperature gradients, mechanical stress) into bionic systems to achieve lifelike self-learning and self-healing capabilities (e.g., self-repairing membranes).
II. Applications and Case Studies
1. Robotics and Motion Control
- Case 1: BionicFlyingFox
A collaborative project by Evonik and Festo, this flying robot mimics bat wing membrane structures:- Evolutionary Design: Evolutionary algorithms simulate millions of years of bat wing evolution, optimizing tear resistance while maintaining aerodynamic efficiency post-damage.
- Dynamic Adaptation: Piezoelectric sensors and reinforcement learning enable real-time wing adjustments for stable flight in turbulent environments.
- Case 2: Octopus-Inspired Soft Robotic Arm
Developed by the Italian Institute of Technology, this soft robot replicates cephalopod adaptability:- Distributed Actuation: Microfluidic units optimized via evolutionary algorithms achieve multi-modal grasping with minimal force error.
- Self-Healing Materials: Silicone-based biomimetic materials, designed using Monte Carlo algorithms, combine high elasticity and autonomous repair.
2. Biomaterials and Medical Engineering
- Case 3: Fermentation-Based Collagen Platform
Evonik’s animal-free collagen technology:- Evolutionary Reverse Engineering: Yeast-edited collagen replicates conserved mammalian triple-helix structures for medical applications.
- Dynamic Self-Assembly: Quorum sensing regulates collagen fiber organization, mimicking extracellular matrix dynamics.
- Case 4: 4D Skin Model for Wound Healing
A collaboration with Revivo BioSystems:- Evolutionary Stress Simulation: Temperature, humidity, and microbial gradients accelerate testing of regenerative hydrogels.
- Cross-Species Regeneration: Salamander-derived genetic modules enhance epidermal-dermal repair in bioengineered hydrogels.
3. Microbiome and Synthetic Ecosystems
- Case 5: Dynamic Avian Intestinal Simulator (DAISy)
Evonik’s gut microbiome model:- Co-Evolution Modeling: Reconstructs microbial networks (e.g., Bacteroidetes-Firmicutes interactions) under antibiotic-free conditions.
- Probiotic Optimization: Evolutionary algorithms enhance butyrate-producing bacteria while suppressing pathogens like Salmonella.
III. Technical Breakthroughs and Methodologies
- Multi-Scale Evolutionary Design: Combines molecular-level evolutionary analysis (e.g., amino acid sequences) with system-level mechanical optimization for functional biomaterials.
- Closed-Loop Experimentation: Platforms like Evonik’s GOBI integrate predictive evolutionary models with lab experiments to refine probiotic therapies.
- Bio-Inspired Autonomy: Evolutionary memory modules enable robots to rapidly generate adaptive strategies in novel environments.
IV. Future Trends and Challenges
- Emerging Synergies
- AI-Evolution Integration: Transformer-based models (e.g., Evo) will accelerate functional module discovery at single-nucleotide resolution.
- Cross-Species Systems: Pollinator-plant coevolution networks inspire next-gen pollination robots and ecological restoration materials.
- Ethical and Technical Hurdles
- Uncontrolled Evolution Risks: Autonomous bionic systems may develop unintended traits (e.g., microbial antibiotic resistance), necessitating real-time monitoring.
- Multi-Physics Modeling: Quantum computing could address current limitations in simulating multi-field interactions (mechanical-electrochemical-thermal).
V. Conclusion
Evobionic is shifting bionics from “form imitation” to “evolutionary empowerment.” By decoding evolutionary history and reconstructing adaptive systems, it has demonstrated transformative impacts in robotics (e.g., BionicFlyingFox), medicine (e.g., DAISy), and ecology. As evolutionary computation merges with synthetic biology, the field aims to realize “design-as-evolution,” ushering in a new era of bio-inspired innovation.
Data sourced from public references. For collaboration or domain inquiries, contact: chuanchuan810@gmail.com