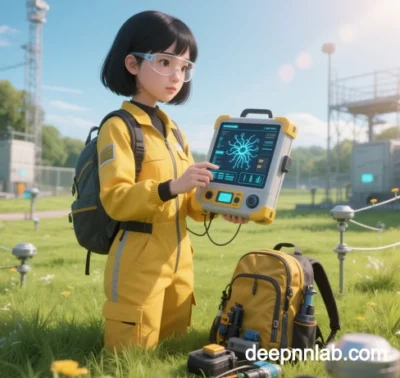
DeepNNLab: Core Value of a Deep Learning Neural Network Laboratory
A Deep Learning Neural Network Laboratory (DeepNNLab) represents a nexus of innovation, where cutting-edge research converges with real-world applications to redefine the boundaries of artificial intelligence. Its core value lies in advancing both theoretical foundations and practical implementations of deep learning, while fostering interdisciplinary collaboration and ethical AI development. Below is an exploration of its key pillars:
I. Pioneering Research in Neural Network Architectures
- Next-Generation Model Design: Innovating novel architectures like spiking neural networks (SNNs) for neuromorphic computing and transformer-based hybrid models for multimodal learning, bridging gaps between biological plausibility and computational efficiency.
- Explainability and Robustness: Developing frameworks to interpret “black-box” models via attention visualization and adversarial robustness training, ensuring AI transparency in critical domains like healthcare and autonomous systems.
II. Cross-Domain Applications Driving Societal Impact
- Healthcare Revolution: Deploying deep learning for early disease detection (e.g., Alzheimer’s biomarkers in MRI scans) and personalized treatment optimization through genomic data integration.
- Sustainable Technologies: Optimizing energy grids via AI-driven demand forecasting and enabling low-power edge AI through model quantization for IoT ecosystems.
- Creative Industries: Leveraging generative adversarial networks (GANs) and diffusion models for art, music, and film production, democratizing creativity while addressing copyright ethics.
III. Collaborative Innovation Ecosystem
- Industry-Academia Synergy: Partnering with tech leaders and startups to translate lab breakthroughs into scalable solutions, such as real-time fraud detection systems for fintech.
- Open-Source Advocacy: Building community-driven tools like federated learning platforms to enable privacy-preserving AI training across decentralized datasets.
IV. Ethical AI and Future-Proof Education
- Responsible AI Governance: Establishing protocols for bias mitigation, data privacy (e.g., GDPR-compliant anonymization), and environmental sustainability in AI training.
- Talent Cultivation: Offering interdisciplinary programs blending neuroscience, computer science, and ethics, with hands-on labs for students to prototype AI-driven robotics or climate models.
V. Cutting-Edge Infrastructure
- High-Performance Computing: Utilizing quantum-accelerated clusters and neuromorphic hardware (e.g., Intel Loihi) to train billion-parameter models 10x faster than conventional GPUs.
- Data-Centric Resources: Curating multimodal datasets (e.g., satellite imagery paired with climate sensors) to fuel breakthroughs in cross-modal learning.
Conclusion: Engineering Intelligence for Tomorrow
DeepNNLab’s core value transcends technological advancement—it embodies a commitment to shaping AI that is powerful, equitable, and human-centric. By marrying theoretical rigor with societal needs, the lab serves as a beacon for ethical innovation, ensuring AI evolves as a force for global progress.
Data sourced from public references. For collaboration or domain inquiries, contact: chuanchuan810@gmail.com