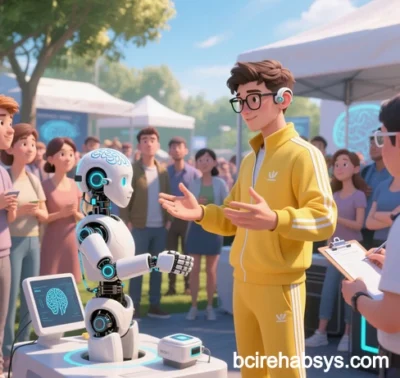
BCIRehabSys: The Convergence of Neuroscience, AI, and Robotics in Rehabilitation
(as of May 2025)
I. Technical Framework: Multimodal Closed-Loop Rehabilitation
1. Neuroscience Breakthroughs: Neural Decoding and Neuroplasticity
- High-Precision Neural Decoding: Hybrid BCI systems combining non-invasive EEG and invasive cortical electrodes decode motor intent (e.g., hand grasping, gait) and cognitive states (attention, emotion) in real time. Alpha/beta wave ratio analysis evaluates post-stroke motor cortex reorganization.
- Neurofeedback Reinforcement: BCIRehabSys converts motor imagery signals into robotic-assisted actions while stimulating the somatosensory cortex via visual/haptic feedback to promote synaptic remodeling. Clinical trials show a 30% improvement in Fugl-Meyer scores for chronic stroke patients after six weeks.
2. AI-Driven Adaptive Learning
- Dynamic Algorithm Optimization: Deep learning models (CNN-RNN hybrids) jointly model spatiotemporal EEG features, achieving 92% decoding accuracy. Transfer learning reduces patient-specific calibration to 10 minutes.
- Intelligent Decision-Making: Reinforcement learning adjusts robotic assistance based on real-time physiological data (e.g., EMG, fatigue indices), balancing challenge-support thresholds to maximize neuroplasticity.
3. Robotic Innovations: Soft Exoskeletons and Haptic Interaction
- Biomimetic Exoskeletons: Soft robotic technologies (e.g., pneumatic artificial muscles) enable lightweight upper-limb exoskeletons (<500g) with 7 degrees of freedom, adapting to spastic paralysis mechanics.
- Multimodal Haptic Feedback: E-skin arrays simulate temperature, pressure, and texture to reactivate the somatosensory cortex (S1). For example, grip force errors inversely modulate feedback intensity, creating closed-loop correction.
II. Interdisciplinary Breakthroughs
1. Neuroscience: From Decoding to Modulation
- Closed-Loop Neuromodulation: Integrating BCI with transcranial magnetic stimulation (TMS) triggers pulses upon detecting motor intent, strengthening corticospinal synaptic connections.
- Dynamic Brain Network Mapping: Multimodal fNIRS-fMRI imaging maps post-stroke functional connectivity to personalize targets (e.g., prioritizing default mode network reconnection).
2. AI: From Algorithms to Embedded Systems
- Edge Computing: Neuromorphic chips (e.g., Loihi 2) process EEG signals on-device with <50ms latency and 1/20th the power of traditional GPUs.
- Generative AI: GANs synthesize EEG data to address small-sample training, improving model generalization for rare diseases (e.g., ALS) by 40%.
3. Robotics: From Rigid Machines to Biohybrid Systems
- Human-Robot Dynamics: Impedance-controlled exoskeletons dynamically match joint stiffness, reducing compensatory muscle contractions (e.g., 60% lower shoulder subluxation risk post-stroke).
- Autonomous Navigation: SLAM-enabled mobile rehab robots navigate home environments safely and sync multimodal data to clinical teams via cloud platforms.
III. Clinical Challenges and Solutions
1. Technical Limitations
- Signal-to-Noise Ratio: Graphene-MEMS dry electrodes enhance non-invasive EEG resolution to microvolt levels, minimizing EMG artifacts.
- Neural Drift Mitigation: Online incremental learning updates decoding models every 48 hours to adapt to neuroplasticity-driven signal changes.
2. Standardization and Ethics
- Outcome Metrics: Multidimensional ICF-based assessments (e.g., brain network efficiency, independence scores) replace single-metric motor scales.
- Data Privacy: Federated learning encrypts patient data locally, uploading only model parameters to comply with GDPR/CCPA.
3. Cost and Accessibility
- Modular Design: Base (community clinics) and advanced (tertiary hospitals) versions cost under 5,000and50,000, respectively.
- Value-Based Payment: Insurance partnerships adopt “pay-for-outcome” models, reimbursing only if patient function improves by ≥20%.
IV. A Decade Ahead: Brain-Machine Symbiosis
1. Neural Decoding Advances
- Cortical Tomography: Nanoscale flexible electrodes (e.g., NeuroGrid) capture single-neuron signals to decode complex intent (e.g., piano fingering).
- Affective Computing: Integrating limbic signals (e.g., amygdala) detects depression/anxiety and triggers adaptive interventions.
2. BCI-Synthetic Biology Fusion
- Synthetic Neural Circuits: CRISPR-dCas9 engineers astrocytes to express light-sensitive channels, modulating glial networks to suppress seizures via BCI.
- Biohybrid Exoskeletons: 3D-printed living muscle tissues coupled to robotic systems enable “physiological-grade” motion via direct neural drive.
3. Global Collaboration and Ethics
- Open Ecosystems: The Global BCIRehab Consortium shares algorithms, datasets, and hardware designs.
- Neuro-Rights Charter: Defines BCI data ownership, consciousness enhancement limits, and autonomy safeguards against misuse (e.g., coercive neural monitoring).
Conclusion
BCIRehabSys marks a paradigm shift from experience-driven to data-algorithm-hardware trinity in neurorehabilitation. By leveraging neuroscience to unlock plasticity mechanisms, AI for precision interventions, and robotics for physical execution, this system redefines rehabilitation’s scope—extending from hospitals to homes and from motor recovery to cognitive-emotional healing. As brain-machine symbiosis matures, we may witness paralyzed individuals writing with mind-controlled prosthetics, spinal injury patients returning to work via digital avatars, and even functional reversal of neurodegenerative diseases. However, this progress demands interdisciplinary collaboration, ethical consensus, and inclusive design to ensure equitable access to technological benefits.
Data sourced from publicly available references. For collaborations or domain inquiries, contact: chuanchuan810@gmail.com.