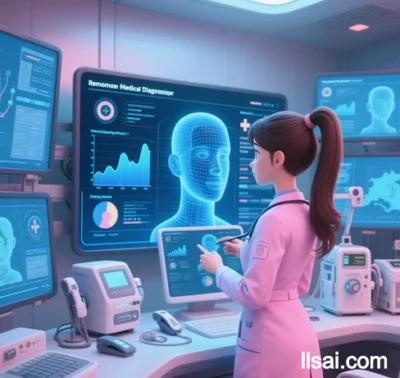
Large Language Model Systems (LLS) in Healthcare: Applications and Technological Advancements (2025 Perspective)
I. Current Applications: Multidisciplinary Integration
1. Clinical Diagnosis & Decision Support
- Intelligent Diagnostic Assistance:
LLS have achieved breakthroughs in medical imaging. Models like Google’s Med-Gemini process extensive contextual data (e.g., lengthy EHRs or surgical videos) to analyze radiology images and interpret complex cases, supporting multimodal reasoning across CT, MRI, and pathology slides.
Specialized applications include neurological disorders, diabetic retinopathy, and lung cancer diagnosis, where LLS integrate patient history, lab results, and imaging features to generate differential diagnoses rivaling specialist accuracy. - Dynamic Decision Optimization:
Reinforcement learning-based systems reduce chemotherapy dosing errors, while federated learning platforms improve diabetic retinopathy models through multicenter data collaboration.
2. Patient Management & Education
- Personalized Interventions:
LLS generate tailored medication guides (e.g., optimizing Warfarin dosing) and enhance chronic disease management (e.g., improving diabetes control metrics). - Clinician-Patient Interaction:
Multimodal GPT models combine imaging analysis, literature review, and natural dialogue, streamlining diagnosis for primary care providers and simplifying device operations via voice interfaces.
3. Drug Development & Research
- Molecular Design & Virtual Trials:
Diffusion models accelerate novel compound discovery (e.g., Alzheimer’s therapeutics), while quantum chemistry simulators predict drug-target interactions with atomic precision.
Digital twins simulate patient physiology to predict chemotherapy-related risks like neutropenia. - Literature Mining:
LLS rapidly parse top medical journals to update treatment protocols and build evidence-based knowledge graphs.
II. Technological Breakthroughs
1. Architectural Innovations
- Hybrid Reasoning Engines:
Integrate symbolic logic (clinical guidelines encoded as decision trees) with deep learning (adaptive node weighting via reinforcement learning) to overcome rigid rule-based systems.
Neuro-symbolic systems (e.g., Transformer + knowledge graphs) enable explainable treatment pathways for conditions like atrial fibrillation. - Multimodal Modeling:
CLIP-like architectures align pathology images with diagnostic reports, while multimodal LLS (e.g., LlaVA-Med) process imaging and text to generate diagnostic insights.
2. Infrastructure Advancements
- Privacy-Preserving Frameworks:
Homomorphic encryption and federated learning enable secure cross-border data sharing, compliant with regulations like GDPR. - Edge-Cloud Synergy:
Photonic accelerators reduce power consumption for portable ultrasound analysis, while quantum entanglement encoding enhances tumor gene detection speed.
3. Reliability & Explainability
- Regulatory Compliance:
Real-time validation ensures AI decisions align with FDA/NMPA guidelines, triggering alerts for deviations. - Interpretability:
Layered explanations using SHAP, LIME, and knowledge graphs address clinical queries, including counterfactual reasoning (e.g., illustrating how D-dimer changes affect embolism risk).
III. Challenges & Future Directions
1. Key Challenges
- Data Quality & Bias:
Manufacturer-specific variations (e.g., GE vs. Siemens MRI data) require adversarial learning for feature alignment. - Clinical Trustworthiness:
Models must achieve high accuracy, explainability, and robustness against adversarial attacks.
2. Emerging Frontiers
- Quantum-Enhanced Systems:
QAOA algorithms optimize radiotherapy dosing, while quantum protocols enable secure cross-facility device synchronization. - Implantable Diagnostics:
Neuromorphic chips and biosensors enable ultra-low-power devices for real-time monitoring of gut microbiota to predict inflammatory bowel disease.
IV. Ethical & Industry Impact
- Human-AI Collaboration:
A tiered autonomy framework balances efficiency and safety: AI handles routine tasks, assists in complex cases, and defers to humans for critical decisions. - Healthcare Ecosystem Transformation:
MLOps providers offer end-to-end solutions from data annotation to regulatory compliance, while insurers adopt digital twin-driven risk models.
Conclusion
LLS have evolved from text-processing tools into intelligent platforms supporting end-to-end healthcare workflows. Despite challenges in data privacy and validation, their integration with quantum computing and edge devices is driving healthcare AI toward hyper-automation and precision. By 2030, LLS are projected to transform advanced medical devices, significantly reducing personalized care costs and redefining the future of medicine.
Data sourced from publicly available references. For collaborations or domain inquiries, contact: chuanchuan810@gmail.com.