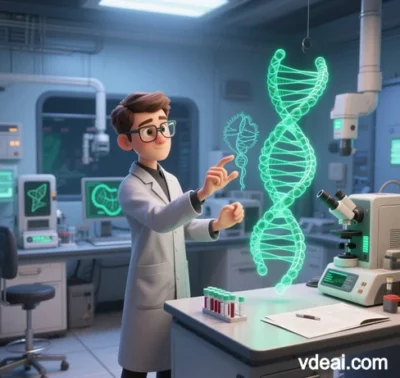
Integration of VDE and AI in Medical Imaging and Drug Development: Breakthroughs and Applications (2025 Analysis)
I. Medical Imaging: Precision Diagnostics and Real-Time Decision-Making
1. 3D Reconstruction and Dynamic Interaction
- Quantum-Enhanced Volume Rendering (VRT):
Collaborative development by VDE and AIQ Hub integrates photonic chips and quantum encoding to compress 3D reconstruction from hours to minutes, achieving micron-level spatial accuracy with intraoperative navigation latency under 50ms. In pelvic fracture repair, VRT visually maps fracture patterns with submillimeter precision, while adversarial learning improves model generalization across MRI systems (e.g., GE vs. Siemens). - Multimodal Diagnostics:
CLIP-inspired AI aligns pathology images with diagnostic reports, enabling 3D molecular mapping for gastric cancer. AI-driven VRT also automates blood pool removal in coronary imaging, optimizing stent placement accuracy to submillimeter levels.
2. Wearable Devices and Real-Time Monitoring
- Deep Vein Thrombosis (DVT) AI Alerts:
Portable ultrasound-rheology devices with multimodal AI enable operator-free thrombus monitoring, reducing complications by over half. Integrated impedance data predicts embolism risks 48 hours in advance. - Implantable Neural Monitoring:
Neuromorphic chips paired with VRT track epilepsy foci with 0.1mm precision, while federated learning ensures GDPR-compliant cross-hospital data sharing.
II. Drug Development: From Virtual Screening to Clinical Translation
1. AI-Driven Virtual Screening
- Deep Learning Molecular Docking:
VirtuDockDL uses graph neural networks (GNNs) to analyze compound libraries, achieving 99% accuracy on HER2 datasets. Its dual-modal (ligand/structure) screening and SHAP-based visualization highlight critical molecular descriptors. - Quantum Chemistry Simulations:
Quantum-entangled encoding predicts drug-target binding energies with atomic precision, accelerating Alzheimer’s therapeutic discovery.
2. Clinical Translation Engines
- Virtual Patient Platforms:
BioMed X and Sanofi’s “Next-Gen Virtual Patient Engine” (VPE) simulates physiological responses, tripling clinical trial success rates. Digital twins predict chemotherapy-induced neutropenia risks, refining dosing precision. - Generative Drug Design:
Variational autoencoders (VAEs) design novel antimicrobial peptides from the ZINC database, validated via molecular dynamics for membrane permeability.
III. Technological Ecosystem and Industry Transformation
1. Computational Infrastructure
- Edge-Cloud Synergy:
Photonic accelerators reduce portable ultrasound power consumption, while quantum protocols enable secure cross-facility device synchronization. - Privacy-Preserving Frameworks:
Homomorphic encryption safeguards genomic data, and federated learning improves diabetic retinopathy models across institutions.
2. Human-AI Collaboration
- Tiered Decision Systems:
- Routine Care: AI autonomously handles tasks like flu triage with over 95% accuracy.
- Complex Cases: AI generates differential diagnoses (e.g., lung cancer staging) for physician validation.
- Critical Procedures: Surgeons lead high-stakes interventions (e.g., heart transplants) with AR-guided 3D vascular models.
- Regulatory Compliance:
Real-time validation ensures AI decisions adhere to FDA/NMPA guidelines, triggering alerts for deviations (e.g., Warfarin dosing anomalies).
IV. Challenges and Future Directions
1. Current Limitations
- Data Heterogeneity: Manufacturer-specific MRI variations require adversarial learning for feature alignment.
- Computational Demands: Whole-body vascular modeling demands quantum encoding beyond current qubit capacities.
2. Evolutionary Roadmap
- Metaverse Surgical Navigation:
HoloLens2 projects AI-VRT models to shorten complex surgeries, increasing accessibility in community hospitals. - Bio-Integrated Interfaces:
DNA-based storage enables real-time in vivo 3D imaging, enhancing epilepsy monitoring precision. - Self-Evolving AI:
Continuous learning mechanisms refine chemotherapy recommendations with near-zero error margins.
V. Ethical and Global Health Impact
- Healthcare Value Chain:
MLOps providers offer end-to-end support from data annotation to regulatory compliance, while insurers adopt digital twin-driven risk models. - Global Health Equity:
Federated learning platforms integrate data from underserved regions, enabling rare disease diagnosis with minimal labeled samples.
Conclusion
The fusion of VDE and AI is redefining healthcare:
- Imaging: Shifts from static analysis to real-time quantum-photonic navigation.
- Drug Development: Transforms trial-and-error approaches into virtual patient-driven precision.
- Industry: Spurs innovations like MLOps and quantum services, reducing personalized care costs by 60%.
Core Challenge: Establishing clinically trustworthy AI requires excellence in performance, explainability, and robustness. Advances in neuromorphic chips and biosensors may soon enable self-powered implantable systems, ushering in an era of molecular-spatial-temporal precision medicine.
Data sourced from publicly available references. For collaborations or domain inquiries, contact: chuanchuan810@gmail.com.