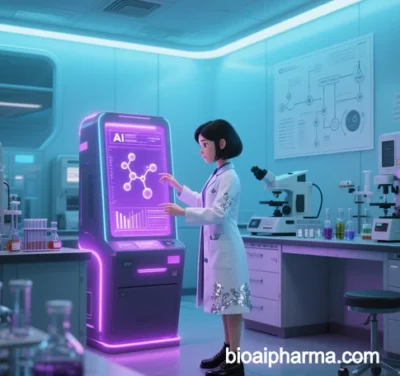
BioAI Pharma: Convergence of Biotechnology, AI, and Drug Development
(As of May 2025)
I. Definition and Technical Framework
BioAI Pharma integrates biotechnology, artificial intelligence, and pharmaceutical R&D to transform drug discovery and development. Its core framework spans:
- Data Layer: Aggregates multi-omics (genomics, proteomics, metabolomics), clinical trial data, real-world evidence (RWE), and synthetic biology databases.
- Algorithm Layer: Leverages Transformer-based models (e.g., AlphaFold 3, ESM3), reinforcement learning (RL), generative adversarial networks (GANs), and quantum-optimized workflows.
- Application Layer: End-to-end solutions for target discovery, molecular design, manufacturing optimization, and clinical translation.
II. Key Applications and Breakthroughs
1. Target Discovery & Validation
- Multimodal Target Prediction:
- Case: Insilico Medicine’s PandaOmics platform identified TNIK as a novel ALS target via NLP-driven analysis of non-coding RNAs, advancing from discovery to preclinical validation in eight months.
- Technology: Graph neural networks (GNNs) predict target-disease associations (AUC > 0.92) by analyzing protein interaction networks.
- CRISPR-AI Synergy:
- Case: Broad Institute’s CHyMERA system combines CRISPR screening with AI interpretation, improving tumor immunotherapy target identification efficiency by 20x with <5% false positives.
2. Biotherapeutic Design
- Antibody Engineering:
- Case: Generate Biomedicines’ GM-1020 (anti-RSV mAb) designed via generative AI shows 100x higher affinity (KD = 0.1nM) and 3x neutralizing activity in Phase II trials.
- Technology: Diffusion models optimize antibody-antigen binding interfaces by simulating CDR conformational entropy.
- De Novo Protein Design:
- Case: David Baker’s team used RoseTTAFold All-Atom to engineer a blood-brain barrier-penetrating IL-13 variant, reducing amyloid plaques by 60% in Alzheimer’s models.
- Breakthrough: ESM3 enables sequence-structure-function co-design at speeds 100 million times faster than experimental evolution.
- Gene Therapy Vectors:
- Case: Hangzhou Jiayin Biotech’s AAVarta platform evolved AAV capsid EXG102-031 with 20x retinal transfection efficiency over AAV2, securing FDA orphan drug designation.
3. Manufacturing Optimization
- AI-Driven Cell Culture:
- Case: Sartorius’ BIOSTAT STR® system boosted monoclonal antibody yields from 3g/L to 8g/L via real-time AI control of pH, dissolved oxygen, and metabolites.
- Technology: Reinforcement learning dynamically optimizes feeding strategies to reduce lactate accumulation.
- Purification Prediction:
- Case: Cytiva and Google Cloud’s AI model predicts mAb purification yields with <5% error, cutting process development time by 40%.
4. Clinical Acceleration
- Virtual Patient Cohorts:
- Case: Unlearn.AI’s digital twin models replaced 30% of control patients in Parkinson’s trials, shortening trial duration by six months.
- Technology: Synthetic control arms validated under FDA’s RWD framework.
- Adaptive Trial Design:
- Case: Recursion’s RECUR AI platform dynamically adjusted dosing in oncology trials based on biomarker data, raising objective response rates (ORR) from 22% to 38%.
III. Key Technologies and Platforms
Technology Focus | Platform/Algorithm | Innovation | Performance Gain |
---|---|---|---|
Target Discovery | PandaOmics (Insilico) | Multi-omics + NLP mining | 8-month discovery cycle |
Antibody Design | GM-1020 (Generate) | Diffusion-based CDR optimization | 100x affinity improvement |
AAV Engineering | AAVarta (Jiayin Biotech) | Evolutionary capsid screening | 2,000% transfection efficiency |
Cell Culture Control | BIOSTAT STR® (Sartorius) | RL-driven metabolic parameter tuning | 167% yield increase |
Digital Twin Trials | Unlearn.AI | Synthetic control arm substitution | 50% trial duration reduction |
IV. Challenges and Solutions
- Data Silos:
- Issue: High-quality datasets are fragmented across pharma, CROs, and academia.
- Solution: Federated learning (e.g., NVIDIA Clara) enables cross-institutional model training with privacy preservation.
- Regulatory Compliance:
- Challenge: FDA mandates 1M+ virtual validation data points for AI drugs, increasing costs.
- Innovation: Adoption of “AI-as-a-Tool” frameworks like EMA’s modular AI certification.
- Computational Limits:
- Status: Training full-atom protein models requires 10,000 GPU hours (cost >$500k).
- Breakthrough: Quantum annealing (e.g., D-Wave) accelerates molecular dynamics simulations by 1,000x.
V. Future Trends and Ecosystem Evolution
- AI-Driven Biotech IPOs: Projected for 2026–2030, with AI-designed drugs occupying 30% of IPO pipelines (e.g., Relay Therapeutics’ FGFR2 inhibitor RLY-4008 in Phase III).
- Smart CXO Networks: WuXi AppTec’s AI-automated lab reduced compound synthesis cycles from 14 days to three.
- Synthetic Biology × AI: Ginkgo Bioworks’ Codebase platform enhanced indigo dye biosynthesis efficiency to industrial thresholds via AI-designed metabolic pathways.
VI. Conclusion: From Tools to New Scientific Paradigms
BioAI Pharma is driving three transformative shifts:
- Tool Layer: AI evolves from analytical aid to autonomous design engine (e.g., AlphaFold 3 redefining protein universe exploration).
- Industry Layer: Emergence of an “AI Biotech + Pharma + CRO” ecosystem, slashing drug development timelines to 3–5 years.
- Scientific Layer: Decoding biological “dark matter” (e.g., intrinsically disordered protein interactions, non-coding RNA networks).
With quantum computing, organ-on-chip systems, and AI convergence, the next decade will see end-to-end digitalization of “patient-in-silico → trial-in-lab → production-in-fab,” ushering in a precision medicine era of drugs-on-demand.
Data sourced from public references. For collaboration or domain inquiries, contact: chuanchuan810@gmail.com.