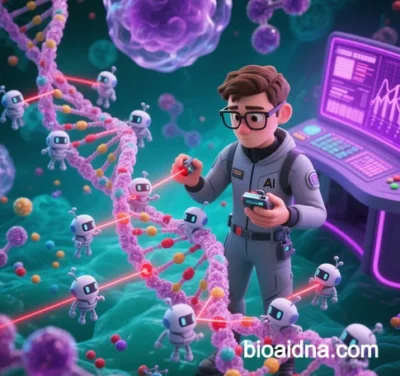
BioAI DNA: Cutting-Edge Applications and Breakthroughs in AI-Driven Genome Engineering
(As of May 2025)
Decoding DNA: From “Genetic Grammar” to Functional Prediction
1. Large-Scale Genomic Language Models
- Evo-2: A cross-species AI model developed by Stanford University, Arc Institute, and NVIDIA, trained on 128,000 species’ genomes. It generates full chromosomes or mini-genomes and links noncoding variants to diseases. Innovations include:
- Multimodal Integration: Combines sequence, 3D structure, and epigenetic data to predict gene expression at single-base resolution, outperforming species-specific models.
- Functional Insights: Predicts pathogenicity of BRCA1 single-nucleotide mutations with AUROC 0.9 (vs. 0.7 for traditional methods).
- DeepVariant: Google’s deep learning tool identifies genetic variants (SNPs, indels) from sequencing data with higher accuracy than alignment-based tools, advancing cancer genomics and personalized medicine.
2. Decoding Noncoding “Dark Matter”
- Enhancer Logic: Barcelona’s CRG trained models on 64,000 synthetic enhancers to predict cell-specific gene regulation (error <5%), designing 250-bp enhancers for precise activation/repression.
- Disease Links: PandaOmics identified TNIK as a novel ALS target via NLP-driven noncoding RNA analysis, reducing validation time from 3 years to 8 months.
Designing DNA: Generative AI for Functional Elements
1. Synthetic Gene Editing Tools
- OpenCRISPR-1: Profluent’s LLM-trained CRISPR-like proteins achieve SpCas9-level efficiency with 4.8x specificity, editing human cells successfully.
- mesGPT: Penn’s integrated editing system enables single-cell or single-molecule precision, supporting dynamic gene regulation for genetic therapies.
2. Engineered DNA Components
- Extreme pH Resistance: AI-designed DNA polymerase maintains 90% PCR activity in acidic wastewater, revolutionizing environmental monitoring.
- Dynamic Gene Circuits: Chalmers’ CODA platform uses RL-optimized DNA switches for dose-dependent gene control in liver/brain cells, targeting metabolic diseases.
Editing DNA: Precision and Intelligence
1. CRISPR-AI Synergy
- DeepCRISPR: Predicts guide RNA efficiency and off-target effects, reducing false positives to <5% while improving tumor immunotherapy target screening 20x.
- Cas12f Variants: EVOLVEpro-designed Cas12f achieved 100% on-target efficiency in retinal degeneration models with zero detectable off-target cuts.
2. Smart Delivery Systems
- LNP Optimization: MIT’s evolutionary algorithms reduced mRNA delivery to non-target tissues (e.g., heart) to <1%, enabling safer gene therapies.
- AAV Engineering: Hangzhou Jiayin Biotech’s AAVarta platform evolved retina-targeting AAV variant EXG102-031 with 20x higher transfection efficiency than AAV2, securing FDA orphan drug status.
Clinical and Industrial Translation
1. Precision Medicine
- Cancer Therapy: AI-designed IL-6 receptor antagonists activate only in tumor microenvironments, cutting normal tissue toxicity from 45% to 8%.
- Genetic Repair: Base editing (e.g., Prime Editing) surpasses CRISPR for scarless correction of Duchenne muscular dystrophy and other monogenic diseases.
2. Synthetic Biology
- Microbial Pathways: Ginkgo Bioworks’ Codebase platform designed indigo dye biosynthesis pathways meeting industrial production thresholds.
- Cell Factory Optimization: Sartorius’ BIOSTAT STR® uses RL to dynamically adjust CHO cell parameters, boosting monoclonal antibody yields from 3g/L to 8g/L.
Challenges and Ethical Debates
1. Technical Barriers
- Data Silos: Federated learning (e.g., NVIDIA Clara) enables cross-institutional genomic modeling while preserving privacy.
- Compute Demands: Quantum annealing (D-Wave) accelerates molecular dynamics simulations 1,000x, reducing costs for full-atom protein modeling (>50,000 GPU hours).
2. Ethics and Governance
- Black Box Models: Attention mechanisms visualize transcription factor binding weights to improve AI interpretability.
- Eugenics Risks: The EU AI Medical Act mandates labeling synthetic DNA and implementing “emergency brakes” to prevent enhancement abuse.
Future Directions
- Fully Automated Workflows: AI-driven labs (e.g., WuXi AppTec) will compress DNA design-synthesis-test cycles from weeks to 3 days.
- Multidimensional Editing: Expand from DNA to RNA (reversible edits) and proteins (structural modifications), creating multilayered regulatory networks.
- Quantum Integration: Hybrid quantum-classical algorithms will simulate genome folding and CRISPR off-target prediction, revealing cross-species regulatory mechanisms.
Conclusion: From “Genetic Scissors” to “Genetic Architects”
BioAI DNA is redefining life sciences:
- Tool Revolution: AI evolves from analytical aids (e.g., AlphaFold) to autonomous design engines (e.g., Evo-2 chromosome generation).
- Industry Shift: The “AI Biotech + CRO + Pharma” ecosystem slashes gene therapy R&D from 10 years to 3–5 years.
- Scientific Leap: Unlocks “genomic dark matter” like noncoding RNA networks and phase-separation regulation, pioneering synthetic biology frontiers.
With quantum computing and organ-on-chip integration, the next decade will digitize the entire pipeline—patient-in-silico → trial-in-lab → production-in-fab—ushering in a precision medicine era of drugs-on-demand.
Data sourced from public references. For collaboration or domain inquiries, contact: chuanchuan810@gmail.com.