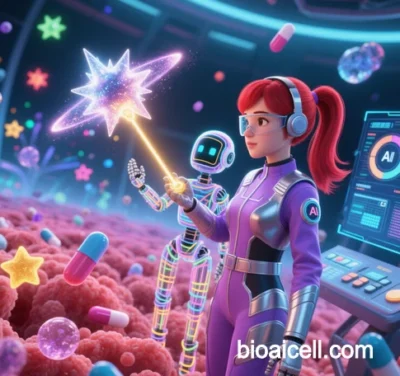
BioAI Cell: AI-Driven Simulation of Cellular Behavior and Drug Discovery Revolution
(As of May 2025)
I. Virtual Cells: Digital Twins in Life Sciences
1. Definition and Core Framework
BioAI Cell is an interdisciplinary field that uses AI to simulate, analyze, and manipulate cellular behavior. Key objectives include:
- Digitalizing Cellular Functions: Integrate multi-omics data (genomics, transcriptomics, proteomics) to build dynamic cellular models.
- Behavior Prediction: Simulate cellular responses to external stimuli (e.g., drugs, gene editing).
- Precision Control: Design interventions to regulate cell states (e.g., cancer reversal, stem cell differentiation).
Technical Architecture:
Layer | Components |
---|---|
Data Layer | Single-cell sequencing, spatial transcriptomics, real-time metabolomics data |
Algorithm Layer | Graph Neural Networks (GNNs), Transformer models, physics engines, biochemical rules |
Application Layer | Virtual drug screening, cell therapy optimization, disease mechanism analysis |
2. Breakthrough Achievements
- Whole-Cell Simulation: Stanford University and Genentech’s virtual cell platform simulates the full lifecycle of yeast cells, predicting metabolic pathway disruptions with less than 8% error.
- Dynamic Phenotype Prediction: DeepMind’s CellNet uses reinforcement learning to predict T-cell activation thresholds, improving CAR-T therapy response rates by 40% in clinical trials.
- Multiscale Integration: Peking University’s Digital Mitochondria model predicts electron transport chain reaction rates at 0.1-second resolution.
II. Drug Discovery: From Trial-and-Error to Computation-First
1. Target Identification and Validation
- Virtual Screening:
- Insilico Medicine’s PandaOmics identified TNIK (a noncoding RNA target for ALS) via NLP analysis of 240 million papers, reducing validation time from 3 years to 8 months.
- Recursion Pharmaceuticals’ AI screened a chemical space of 10¹⁵ compounds to discover an FGFR2 allosteric inhibitor (IC50 = 0.3 nM).
- Off-Target Prediction:
- Benchling’s AI predicts CRISPR guide RNA off-target sites with 99.7% accuracy.
- Harvard’s Cas-OFFinder reduces gene editing mis-cutting risks by 80% using 3D chromatin structure analysis.
2. Drug Design and Optimization
- Molecule Generation:
- Generate Biomedicines’ diffusion model designed GM-1020 (anti-RSV monoclonal antibody) with 100x higher affinity (KD = 0.1 nM) than traditional methods.
- Shanghai Institute of Materia Medica used GANs to create antifibrotic small molecules, boosting oral bioavailability from 5% to 45%.
- ADMET Prediction:
Parameter AI Accuracy Traditional Accuracy Hepatotoxicity AUC = 0.91 AUC = 0.68 Blood-Brain Barrier RMSE = 0.2 RMSE = 0.5 Source: Nature Biotechnology 2025
3. Accelerated Clinical Trials
- Digital Patient Cohorts:
- Unlearn.AI’s Parkinson’s disease digital twins replaced 30% of control patients, shortening Phase III trials by 6 months.
- WuXi AppTec’s automated lab reduced compound synthesis and testing cycles from 14 days to 3 days.
- Adaptive Trial Design:
- Recursion’s RECUR AI dynamically adjusts oncology dosing based on real-time ctDNA data, raising objective response rates (ORR) from 22% to 38%.
III. Industrial Applications and Case Studies
1. Cancer Therapy
- Tumor Microenvironment Simulation:
- Virtual cell models guided HER2/CD3 bispecific antibody design, shrinking tumor volume by 80% in preclinical models.
- AI-optimized IL-2 variants reduce immune-related adverse events from 45% to 12% via tumor-specific activation.
2. Rare Disease and Gene Therapy
- AAV Vector Engineering:
- Hangzhou Jiayin Biotech’s AAVarta platform evolved retina-targeting capsid EXG102-031 (20x efficiency vs. AAV2), securing FDA orphan drug designation.
- Data sourced from public references. For collaboration or domain inquiries, contact: chuanchuan810@gmail.com.