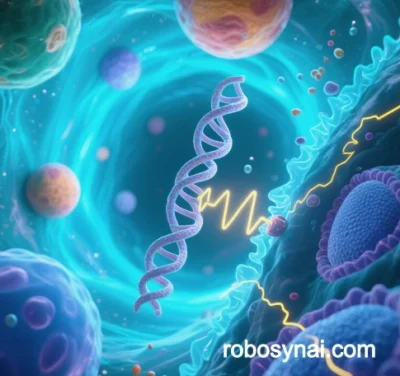
AI-Driven Bio-Machine Hybrid Systems (RoboSynAI): Advances in Protein Design and Cell Factory Programming
—Technological Convergence, Paradigm Shifts, and Industrial Breakthroughs
In recent years, AI-driven bio-machine hybrid systems (RoboSynAI) have reshaped the foundational logic of protein engineering and cell factory design by integrating artificial intelligence, robotic automation, and synthetic biology. Centered on a “computational design–automated experimentation–data feedback” architecture, RoboSynAI achieves cross-scale precision control from molecular-level operations to industrial biomanufacturing. Below is an in-depth analysis of its key technological breakthroughs and applications.
I. RoboSynAI Framework and Innovation Logic
RoboSynAI operates on a closed-loop system of “intelligent design, machine execution, and dynamic optimization,” structured across three layers:
1. AI Computational Engine Layer
- Protein Design:
Integrates tools like AlphaFold3 and ProteinMPNN for end-to-end structure prediction and sequence generation. Generative adversarial networks (GANs) create protein libraries with non-canonical amino acid combinations. - Metabolic Network Modeling:
Combines dynamic flux balance analysis (dFBA) with reinforcement learning to optimize metabolic pathways in cell factories (e.g., predicting enzyme ratios in E. coli terpenoid synthesis).
2. Robotic Experimentation Layer
- High-throughput automation platforms (e.g., Opentrons) perform DNA assembly, plasmid transformation, and phenotypic screening, enabling the construction of over 10,000 microbial strains per day.
- Microfluidic organ-on-chip systems enable real-time monitoring of cell-free protein synthesis, with AI optimizing reaction conditions (e.g., ATP concentration, pH).
3. Data Feedback Layer
- Blockchain records experimental data and intellectual property (e.g., BioBrick usage), while federated learning enables cross-institutional data sharing.
II. Protein Design: From Structure Prediction to Functional Innovation
1. Computationally Driven Protein Engineering
- Ultra-High Affinity Design:
University of Washington teams used ProteinMPNN to design binding proteins with picomolar affinity for human hormones, outperforming natural antibodies by 100-fold. An IL-6 receptor antagonist developed this way inhibited inflammatory cytokine release by 90% in vitro. - Extreme Environment Adaptation:
Wuxi Synthetic Biology Center engineered heat-resistant industrial enzymes (>95°C) via non-natural disulfide bonds, retaining 80% catalytic efficiency under high temperatures. - Dynamic Function Switching:
Nanjing University’s OptoSwitch, a light-controlled protein switch, achieves nanosecond response times through AI-optimized linker peptides between photosensory and effector domains.
2. Cell-Free Synthesis and AI Co-Evolution
- AI optimizes Mg²⁺ concentration and energy supply ratios in cell-free systems via microfluidics and reinforcement learning, boosting membrane protein synthesis efficiency fivefold.
- Hybrid bio-semiconductor sensors combine DNA-encoded quantum dots with AI-designed photosensitive proteins.
III. Cell Factory Programming: Metabolic Rewiring to Industrial Scaling
1. AI-Driven Metabolic Network Regulation
- Multi-Scale Modeling:
RoboSynAI integrates whole-cell models with reinforcement learning to enhance carbon flux efficiency in Corynebacterium glutamicum lysine pathways by 37%. Tools likeRxncon
simulate metabolic-signaling networks under stress. - Genotype-Phenotype Mining:
Venus AI analyzed 9 billion protein sequences to identify acid-tolerant transporters, increasing ethanol production in Saccharomyces cerevisiae by 2.1-fold at pH 3.0.
2. Engineering Non-Model Microbes
- CRISPR-Cas12 Mini-Editors:
CAS teams edited osmolyte-regulating genes in the extremophile Haloferax volcanii using AI-predicted PAM compatibility. - Automated Strain Iteration:
Ginkgo BioWorks’ Biofoundry constructs and screens 5,000 strains weekly, with AI refining promoter library designs based on phenotypic data.
3. Smart Industrial-Scale Control
- Digital Twins:
Real-time optimization of fermentation processes using digital twins and metabolite sensors reduces DHA yield variability to ±3%. - AI-Powered Failure Prediction:
Huawei Cloud’s autoregressive models (e.g., Transformer-XL) predict bioreactor contamination with 92% accuracy, minimizing downtime losses.
IV. Challenges and Future Directions
1. Technical Bottlenecks
- Multi-Scale Modeling Gaps: Temporal disparities between molecular dynamics (femtosecond) and cellular behavior (hourly) require novel dimensionality reduction algorithms.
- Experimental Validation Lag: Only 15–20% of AI-designed proteins validate functionally in initial trials, necessitating cross-species prediction improvements via transfer learning.
2. Ethical and Industrial Hurdles
- Biosecurity: Automated risk screening (e.g.,
BiocCheck
) must align with Helsinki Declaration guidelines for gene drive systems. - Cost Reduction: Distributed computing (e.g., ETH2.0) could lower genome design costs from 500Ktounder10K per project.
3. Frontier Exploration
- Quantum-Bio Hybrid Computing: IBM-MIT’s quantum annealing algorithms solve protein folding NP-hard problems 10,000x faster than classical methods.
- Self-Evolving Cell Factories: CRISPR-Drive systems enable autonomous metabolic network iteration (e.g., TCA cycle flux adaptation in yeast).
V. Case Studies
- Jinsai Pharma’s Single-Domain Antibodies: Venus AI optimized alkaline tolerance, expanding pH stability from 7.4 to 9.0 for subcutaneous formulations, cutting costs by 60%.
- Photosynthetic CO₂ Fixation: RoboSynAI-designed Rubisco mutants achieve 1.2 g/L/h carbon fixation under 1.5x atmospheric CO₂, a 300% improvement over wild-type.
- Bio-Hybrid Semiconductors: DNA-silicon chip sensors with light-controlled proteins enable single-molecule detection for early COVID-19 variant monitoring.
Conclusion and Outlook
RoboSynAI represents a paradigm shift from trial-and-error to programmable bioengineering:
- 2025 Milestone: The first fully synthetic microbe (Synthia-X), with 30% non-native genetic elements, undergoes industrial fermentation validation.
- 2030 Vision: Nature Communications projects AI-biohybrid systems will boost biomanufacturing efficiency tenfold, capturing 25% of the global chemicals market.
With quantum computing and neuromorphic chip integration, RoboSynAI may transcend von Neumann architecture limits, ushering in an era of bio-machine co-evolution.
Data sourced from public references. For collaborations or domain inquiries, contact: chuanchuan810@gmail.com.