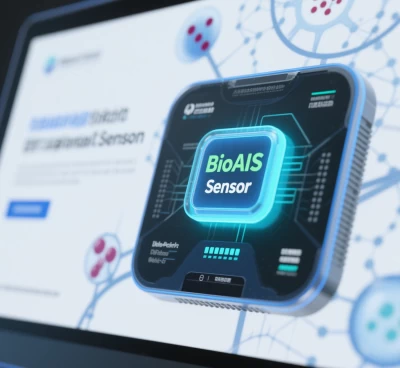
BioAISensor: An In-Depth Analysis
I. Core Definition and Origins
BioAISensor represents an advanced interdisciplinary technology integrating biosensing and artificial intelligence (AI). It focuses on real-time processing and intelligent decision-making for physiological, chemical, or environmental data collected by biosensors, enabling a closed-loop transition from “signal detection” to “smart diagnostics.”
Technical Essence:
- Biosensors: Composed of biorecognition elements (enzymes, antibodies, DNA) and physicochemical transducers (electrochemical/optical/piezoelectric), converting biological responses into quantifiable signals.
- AI Empowerment: Machine learning (ML) and deep learning (DL) optimize sensor performance, including noise reduction, pattern recognition, and predictive modeling.
II. Technical Architecture and Innovations
- System Architecture Layers:
Layer Functional Modules Key Technologies Sensing Layer Biorecognition & Signal Conversion Nanomaterials / Microfluidics / Flexible Electronics Computing Layer Edge AI Chip Processing TensorFlow Lite / Edge Computing Frameworks Decision Layer Multimodal Data Fusion & Inference Knowledge Graphs / Reinforcement Learning - Key Technological Breakthroughs:
- Signal Enhancement: AI algorithms (e.g., CNNs) improve signal-to-noise ratios, boosting glucose sensor precision to 0.1mmol/L.
- Dynamic Calibration: RNNs enable real-time environmental interference compensation (temperature, humidity), reducing wearable heart rate monitoring errors to <2%.
- Label-Free Detection: GANs create virtual biorecognition elements, eliminating antibody dependency (e.g., Etongue sensors for heavy metal detection).
III. Applications and Case Studies
- Healthcare Monitoring:
- Mental Health: Flexible epidermal electrodes + AI emotion models detect depression relapse via sweat cortisol (89% accuracy).
- Early Cancer Screening: Nanopore sensors + AI identify circulating tumor DNA (ctDNA), achieving 92% detection rate for Stage I breast cancer.
- Chronic Disease Management: Smart contact lenses (Google Verily) monitor tear glucose, using LSTM networks to predict diabetic complications.
- Environmental and Food Safety:
Scenario Technology Solution Performance Metrics Water Quality Monitoring Microbial fuel cells + Random Forest BOD detection in 15 minutes Pesticide Residue Detection Graphene quantum dot sensors + SVM Carbendazim detection limit: 0.01ppm Pathogen Identification Impedance biosensors + CNNs 99.7% specificity for Salmonella - Industrial and Military Innovations:
- Biomanufacturing: Fermentation pH/O₂ sensors + reinforcement learning boost antibiotic yields by 23%.
- Military Health Systems: The U.S. Tactical Biosensor system uses sweat metabolites to predict heatstroke (<30-second response).
IV. Challenges and Ethical Considerations
- Technical Bottlenecks:
- Data Heterogeneity: Spatiotemporal alignment challenges for multimodal biosignals (electrochemical/optical/mechanical).
- Miniaturization Paradox: Power constraints (<1mW) in flexible electronics conflict with AI computational demands.
- Long-Term Stability: Enzyme degradation limits biosensor lifespan (<30 days).
- Ethical and Safety Issues:
- Privacy Risks: Brain-computer interface sensors vulnerable to neural data hacking.
- Algorithmic Bias: AI models trained on Western populations show 18% higher misjudgment rates for Asian physiological signals.
- Ecological Impact: Nanopollution risks from widespread environmental nanosensors.
V. Future Directions
- Converging Technologies:
- Quantum Biosensing: Diamond NV center sensors + quantum ML enable single-molecule detection.
- Organ-on-a-Chip Integration: Liver/kidney chips + AI simulate drug metabolism, cutting preclinical trial timelines by 60%.
- Neuromorphic Computing: Memristor arrays mimic biological synapses for adaptive learning.
- Standardization and Ecosystem:
- Certification Frameworks: ISO/IEC JTC 1 working groups to establish AI-biosensor performance standards.
- Open-Source Platforms: OpenAI’s Biosensor Toolkit offers 200+ pre-trained models (e.g., ECG-Arrhythmia v4).
Conclusion
BioAISensor marks a paradigm shift from passive detection to proactive intervention in biosensing. Its breakthroughs in precision medicine, environmental monitoring, and industrial applications are redefining human interaction with biological data. Despite ethical and technical hurdles, advancements in federated learning and neuromorphic chips position BioAISensor for widespread industrial adoption by 2030.
If you are interested in purchasing this domain, please contact: chuanchuan810@gmail.com
BioAI Sensor(生物人工智能传感器) 是一种结合 生物传感技术 与 人工智能(AI) 的智能系统,用于实时监测、分析生物信号或环境中的生物分子,并通过AI算法实现数据解读、预测或决策。以下是详细解析:
1. 核心组成
生物传感部分:
利用生物分子(如酶、抗体、DNA)或生物材料(如细胞、组织)作为识别元件,特异性检测目标物(如葡萄糖、病原体、蛋白质)。
常见技术:电化学传感器、光学传感器(荧光/比色)、纳米孔测序等。
AI集成部分:
通过机器学习(如深度学习、强化学习)处理复杂生物信号,提高检测精度或预测能力。
典型应用:噪声过滤、模式识别、实时决策。
2. 工作原理
信号采集:生物传感器捕获目标物(如血液中的血糖),转化为电/光信号。
数据预处理:AI去除基线漂移、环境干扰(如卷积神经网络去噪)。
分析预测:AI模型(如LSTM、随机森林)分类或回归,输出结果(如疾病风险评分)。
3. 典型应用场景
领域 应用案例 技术结合
医疗诊断 无创血糖监测(如Google智能隐形眼镜) 电化学传感器 + 时间序列预测模型
环境监测 水中重金属离子检测(如CRISPR-Cas12a荧光传感器 + AI图像分析) 生物分子开关 + 计算机视觉
食品安全 肉类新鲜度检测(如微生物传感器阵列 + 机器学习分类) 代谢物传感 + 支持向量机(SVM)
合成生物学 细胞工厂代谢物动态调控(如荧光报告基因 + 强化学习优化) 基因电路 + DDPG算法
4. 技术优势
高灵敏度:AI可识别传统方法无法检测的微弱信号(如单分子水平)。
实时性:边缘计算(如TensorFlow Lite)支持现场快速分析。
自适应学习:持续优化模型以适应个体差异(如个性化健康监测)。
5. 挑战与解决方案
数据噪声:
使用生成对抗网络(GAN)合成训练数据,增强模型鲁棒性。
生物传感器漂移:
AI动态校准(如递归神经网络RNN修正基线)。
伦理与隐私:
联邦学习(Federated Learning)实现分布式数据训练,保护用户隐私。
6. 代表研究/产品
DeepMind Health:AI分析视网膜图像预测糖尿病视网膜病变。
SARS-CoV-2 AI传感器:结合CRISPR与智能手机摄像头,实现COVID-19快速诊断。
Neuralink:脑机接口传感器 + AI解码神经信号。
7. 未来方向
闭环系统:BioAI传感器与执行器联动(如胰岛素泵自动调节)。
多模态融合:整合基因测序、蛋白质组学等数据,构建全息健康模型。
如需具体技术实现(如用Python处理生物传感器数据),可进一步探讨!