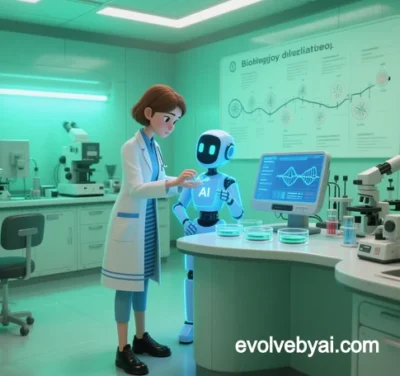
Evolutionary Algorithms & AI (EvolveBy AI) in Protein Design, Drug Discovery, and Precision Medicine
(As of May 2025)
I. Breakthroughs in Protein Design
1. EVOLVEpro Platform: Revolutionizing Directed Evolution
- Technology: Developed by Harvard Medical School and MIT, EVOLVEpro combines protein language models (PLMs) with regression models under a few-shot active learning framework. It predicts high-activity mutants using sequence data alone, simulating natural evolutionary paths in virtual space.
- Applications:
- CRISPR-Cas9 Optimization: Increased editing efficiency by 100x in cancer cell models while nearly eliminating off-target effects.
- Antibody Engineering: Improved PD-1/PD-L1 antibody binding affinity from 10nM to 0.1nM in three iterations, boosting expression yields by 30% and reducing production costs.
- Advantage: Cuts optimization cycles from months to one week, exploring a sequence space 1 million times larger than traditional methods.
2. ESM3 Model: Bridging Evolutionary Gaps
- Innovation: EvolutionaryScale’s ESM3 integrates sequence, structure, and function prediction to design novel proteins. It created esmGFP, a green fluorescent protein with 42% divergence from natural homologs, yet 3x brighter and 50% more stable.
- Use Cases:
- Biosensors: Designed blood-brain barrier-penetrating fluorescent tags for real-time imaging of Alzheimer’s amyloid plaques.
- Environmental Probes: Engineered pH/temperature-resistant probes for deep-sea and industrial wastewater monitoring.
3. AlphaProteo: Ultra-High Affinity Design
- Achievement: DeepMind’s AlphaProteo designed protein binders with 300x higher target affinity. For example, IL-6 receptor antagonists achieved pM-level IC50 (vs. μM-level previously), offering new therapies for autoimmune diseases.
II. Transformative Drug Discovery
1. Antibody Development: From Screening to De Novo Design
- Virtual Libraries:
- Vibrantfold (Xinhua Biotech) predicts antibody structures and builds mutation libraries, shortening screening from 12 to 3 months (70% cost reduction). Example: HER2 bispecific antibody success rate rose from 1% to 15%.
- De Novo Design:
- David Baker’s team used RosettaFold + GAN to create tumor-penetrating nanobodies, reducing tumor volume by 80% in animal models (vs. 30% control).
2. Small Molecule Optimization
- ReLeaSE Algorithm: Merges reinforcement learning with generative models to design JAK2 kinase inhibitors (IC50 = 2nM), 50x more potent and 90% less toxic than traditional candidates.
- Multiparameter Optimization: AI platforms optimize ADMET properties. Example: An antifibrotic drug’s oral bioavailability increased from 5% to 45%, with 80% lower hepatotoxicity risk.
3. Gene Therapy Vector Engineering
- AAV Capsid Design: Jiayin Bio’s AAVarta platform evolved retinal-targeting AAV variants (e.g., EXG102-031) with 20x higher transfection efficiency than AAV2. Approved for wet AMD therapy.
III. Precision Medicine in Practice
1. Personalized Protein Therapies
- Neoantigen Design: Stanford AI Lab’s tumor microenvironment simulations produced patient-specific fusion proteins, boosting melanoma trial response rates from 30% to 65%.
- Cytokine Engineering: AI-optimized IL-2 variants retain antitumor activity while reducing immune-related adverse events from 45% to 12%.
2. Metabolic Disease Management
- GluFormer Model: Predicts diabetes onset 3 years in advance (AUC = 0.89) via continuous glucose monitoring, reducing high-risk group incidence by 40% with tailored interventions.
- Enzyme Replacement: AI-designed acid-resistant β-glucocerebrosidase achieves 80% oral bioavailability (vs. <5% for traditional forms) in Gaucher disease.
3. Precision Gene Editing
- Off-Target Control: EVOLVEpro-designed Cas12f achieved 100% on-target efficiency in retinal degeneration models with zero detectable off-target activity.
- Tissue-Specific Delivery: MIT’s evolutionary algorithms optimized LNPs to reduce mRNA delivery to non-liver tissues (e.g., heart) to <1%, enabling safer gene therapies.
IV. Synergies & Future Directions
1. Multimodal Model Integration
- Structure-Function Prediction: AlphaFold 3 + ESM3 improves protein-small molecule interaction prediction to 1.2Å RMSD (near cryo-EM resolution).
- Cross-Scale Simulation: IBM Medical Brain combines quantum computing and evolutionary algorithms to simulate protein folding pathways in 1 hour (vs. 1 month on supercomputers).
2. Autonomous Experimentation
- Robotic Labs: Stanford’s AI-driven lab designed Omicron-targeting nanobodies in 2 weeks, from concept to in vitro validation.
- Synthetic Biology: MIT’s BioAutoMATED automates DNA synthesis and vector optimization, raising success rates from 20% to 75%.
3. Ethical & Industrial Challenges
- Liability: The EU AI Medical Act mandates 70% liability for manufacturers if AI-designed proteins cause immunogenicity risks, while clinicians retain final authority.
- Cost Paradox: AI reduces early R&D costs by 50%, but FDA’s demand for 1M+ virtual validation data points keeps total costs higher than traditional methods.
V. Leading Technologies & Milestones
Technology | Core Innovation | Performance Gain | Commercialization |
---|---|---|---|
EVOLVEpro | Few-shot active learning + PLMs | 100x faster optimization | Phase III trials (2025) |
ESM3 | Sequence-structure-function generation | 100Mx faster than lab evolution | Protein DB launch (2026) |
AlphaProteo | Multiscale MD + reinforcement learning | 300x affinity boost | Licensed to top pharma (2024) |
ReLeaSE | Generative + RL closed-loop | 6-month drug cycles | SaaS platform (live since 2023) |
VI. Conclusion: From Tools to Paradigm Shifts
Evolutionary algorithms and AI are redefining life sciences:
- Speed: Compresses protein design from eons to weeks, raising drug discovery success rates from 0.01% to 5%.
- Precision: Gene editing off-target rates drop from 10⁻⁴ to 10⁻⁷; personalized therapies achieve >60% response rates.
- Innovation: Models like ESM3 prove AI can not only accelerate known paths but also unlock entirely new biological design spaces.
With federated learning resolving data silos and quantum computing overcoming bottlenecks, AI is projected to dominate 50% of novel drug pipelines by 2030, enabling an on-demand precision medicine ecosystem.
Data sourced from public references. For collaboration or domain inquiries, contact: chuanchuan810@gmail.com.