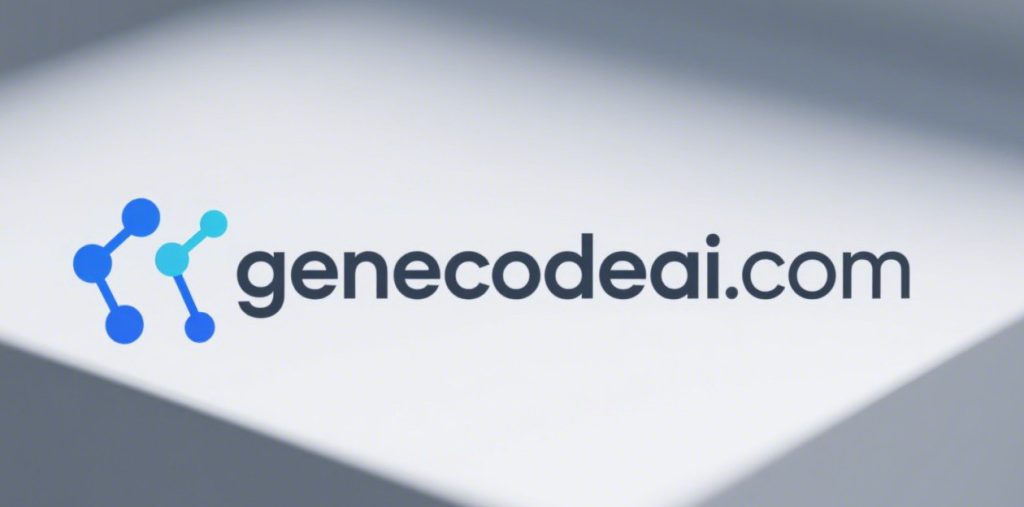
GeneCodeAI: The Fusion of Genomics and Artificial Intelligence
Gene Code AI represents the cutting-edge integration of artificial intelligence (AI) with genomics, aiming to decode the complex logic of genetic sequences and enable intelligent design, editing, and regulation of DNA through machine learning and deep learning algorithms. This technology is revolutionizing life sciences, from fundamental biology to clinical applications. Below is an in-depth analysis of its principles, breakthroughs, and future trends:
I. Technical Essence and Core Principles
- The Nature of Genetic Coding:
- Genetic coding involves the storage of hereditary information in DNA base sequences (A-T-C-G), including protein synthesis instructions (exons) and regulatory logic (introns, promoters).
- Traditional genomics struggles with the complexity of gene regulatory networks (e.g., transcription factor binding sites, epigenetic modifications).
- AI’s Role:
- Pattern Recognition: Deep learning models (e.g., CNNs, Transformers) identify hidden patterns in DNA sequences, such as promoter regions or non-coding RNA functions.
- Generative Design: Generative AI (GenAI) mimics natural evolution to design synthetic DNA sequences for optimized traits (e.g., disease resistance).
- Dynamic Modeling: Reinforcement learning models predict phenotypic outcomes of gene edits (e.g., CRISPR interventions).
II. Core Technological Breakthroughs
- Gene Sequence Decoding:
- AI-Driven Regulatory Insights:
- UC San Diego teams use AI to pinpoint “activation codes” in DNA, improving regulatory sequence identification by 60%.
- Vienna’s IMP Institute deciphers 3D structural dynamics of non-coding regions.
- Multi-Omics Integration:
- AI models (e.g., Deep Genomics) predict metabolic impacts of SNPs, aiding rare disease diagnosis.
- Synthetic Gene Design:
- AI-Optimized Sequences:
- Chalmers University’s AI platform generates synthetic DNA tailored to target expression levels (e.g., insulin production).
- GenAI tools (e.g., AlphaCode) accelerate antiviral crop gene design.
- CRISPR Guidance:
- DeepCRISPR predicts sgRNA efficiency and off-target risks, boosting editing success rates to >90%.
- Gene-Phenotype Modeling:
- Disease Mechanism Analysis:
- AI identifies cancer driver mutations and drug response links using TCGA data.
- Cross-Species Evolution:
- Generative models simulate adaptive mutations for synthetic biology applications.
III. Applications and Case Studies
- Precision Medicine:
- Cancer Therapy:
- AI monitors tumor genome mutations (e.g., EGFR/KRAS) via liquid biopsy to predict drug resistance.
- GenAI designs personalized mRNA cancer vaccines (e.g., BioNTech’s pipeline).
- Genetic Disorders:
- AI-engineered mRNA therapies restore lung function in cystic fibrosis (Translate Bio’s Phase III trials).
- Agriculture and Synthetic Biology:
- Resilient Crops:
- GenAI designs drought-resistant genes (e.g., C4 photosynthesis optimization).
- Microbial Engineering:
- AI enhances metabolic pathways in E. coli for biofuel/antibiotic production.
- Gene Therapy Innovations:
- Viral Vector Design:
- AI predicts immune-evading mutations in AAV capsids for safer gene delivery.
- Epigenetic Programming:
- Deep learning models guide reversible DNA methylation editing.
IV. Challenges and Ethical Debates
- Technical Limitations:
- Data Quality: Sequencing noise may mislead AI models; single-cell precision is critical.
- Model Interpretability: Black-box algorithms hinder clinical trust and regulatory approval.
- Ethical Risks:
- Genetic Privacy: Genomic data leaks risk discrimination or insurance denial.
- Biosafety: AI-designed synthetic genes could be weaponized without global oversight.
- Equity Concerns:
- High costs of gene editing (e.g., CRISPR) may widen healthcare disparities.
V. Future Trends and Strategic Value
- Technological Synergies:
- Quantum Computing: Accelerates genome analysis and energy-state simulations.
- Neuro-Genetic Interfaces: Merging Neurotech BCI with Gene Code AI to study epigenetics in neuroplasticity.
- Industrial Pathways:
- Lab Automation: AI-driven robots automate gene synthesis and editing workflows (e.g., Tesla Bot prototypes).
- Open-Source Ecosystems: Platforms like BioGPT democratize access to AI models.
- Global Competition:
- U.S. Leadership: DeepMind and OpenAI collaborate with pharma giants (e.g., Moderna) on AI tools.
- China’s Advancements: BGI and Tencent AI Lab develop models (e.g., “Shennong DNA”) for crop genome optimization.
Conclusion
Gene Code AI embodies the fusion of life sciences and AI, enabling precise manipulation of biological systems through algorithmic decoding and design. While already impacting healthcare and agriculture, its full potential remains untapped. Over the next decade, advancements in multimodal AI, quantum computing, and automation will position Gene Code AI as a cornerstone for addressing global challenges like food security and cancer. However, balancing innovation with ethical safeguards is crucial to ensure this transformative power benefits humanity equitably.