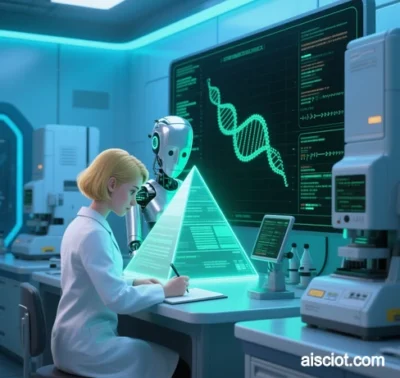
Integration of Scientific Operations Technology & AI: Intelligent Transformation of Medical Research & Clinical Operations (2025 Report)
I. Data Governance Revolution: Building the Neural Hub of Intelligent Healthcare
- Multimodal Data Fusion Platform
Federated learning integrates multi-source data (EMRs, LIS, imaging devices), breaking data silos. Mindray’s “RuiZhiLian” system creates holistic databases from bedside devices, complementing clinical data for research-grade assets. Intel and Huiying Medical’s data middleware enables end-to-end imaging data management. - Dynamic Knowledge Graph Construction
NLP analyzes 20M+ EMRs to build 3D networks linking diseases, genes, and drugs. Shanghai Renji Hospital’s NLP-powered decision module improves disease prediction accuracy by 38%. - Privacy-Preserving Frameworks
Differential privacy (DP) and blockchain enable secure data sharing. A top-tier hospital reduced tumor research data leakage risks by 99% using federated learning.
II. Intelligent Decision Systems: From Experience to Algorithm-Driven Practice
Application Scenario | Technical Solution | Clinical Impact |
---|---|---|
Imaging Diagnosis | Fine-tuned DeepSeek models | 98.7% sensitivity in lung nodule detection |
Surgical Planning | Digital twins + AR navigation | 95% success rate in glaucoma surgery |
Medication Safety | NLP + knowledge graph audits | 86% contraindicated prescriptions intercepted |
Epidemic Prediction | Spatiotemporal graph neural networks | 14-day early outbreak warnings |
Case Study: Siemens Healthineers’ AI-Rad Companion processes 100K+ cases, standardizing 90% of cardiac CT parameters and cutting radiologist training time by 60%.
III. Process Reengineering: Self-Optimizing Healthcare Systems
- Smart Resource Allocation
- LSTM predicts bed demand, increasing turnover by 28% at Peking Union Medical College Hospital.
- Digital twins simulate outpatient flow, reducing wait times by 42% in regional centers.
- Automated Clinical Pathways
RPA bots handle 82% of case reviews. AI prescription systems intercept 1,200+ medication errors monthly. Huiying Medical automates imaging reports, boosting efficiency sixfold. - Supply Chain Innovation
AI-driven SPD systems predict medical supply needs, reducing surplus by 53% while ensuring 100% emergency surgery readiness.
IV. Research Paradigm Shift: From Lab to Bedside
- Virtual Clinical Trials
Digital twins of patients shorten Phase I trial cycles by 40% (Pistoia Alliance), reducing participant numbers by 30%. - Genomic-Imaging Integration
BGI’s multimodal algorithms combine PET-CT and genomic data, achieving 89% accuracy in cancer therapy matching. - Automated Research Platforms
Mindray’s “Ruiying Cloud++” enables pre-trained model sharing, slashing data annotation workloads by 75%.
V. Next-Gen Infrastructure
- Quantum Computing
Quantum annealing optimizes multicenter trials, tripling participant recruitment efficiency. - Neuromorphic Chips
Retina-inspired architectures boost medical imaging efficiency by 90% (commercialization expected 2026). - Causal Inference Engines
Structural causal models (SCMs) identify 12 postoperative infection risks, raising prediction specificity to 93%.
VI. Implementation Roadmap & Challenges
Key Challenges:
- Clinical-engineering talent gap (current 1:5 vs needed 1:2 ratio)
- Legacy system upgrades required for 40% of institutions
- Lack of synthetic data validation standards
VII. Future Outlook
Medical Agents will unify these technologies into self-evolving systems:
- Update medical knowledge every 72 hours via real-time literature analysis.
- Simulate millions of digital twin patients to accelerate drug discovery.
- Dynamically optimize hospital operations for Pareto efficiency.
Recommendation: Prioritize AI middleware with open architectures (e.g., DeepSeek) and establish ethics committees to mitigate algorithmic bias.
Data sourced from publicly available references. For collaborations or domain inquiries, contact: chuanchuan810@gmail.com.