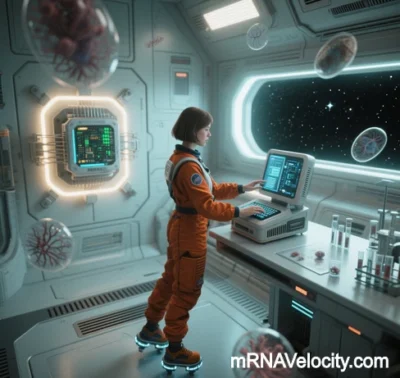
mRNA Velocity in Developmental Biology and Disease Mechanisms: Applications and Prospects (2025 Update)
mRNA Velocity (RNA velocity) is a breakthrough single-cell RNA sequencing (scRNA-seq) technology that infers the directionality and temporal trajectory of cell differentiation by analyzing the dynamic balance between unspliced and spliced mRNA. Since its introduction in 2018, it has evolved into a core tool for dissecting developmental biology, disease mechanisms, and therapeutic design. Below, we explore its technical principles, applications, challenges, and future directions.
I. Technical Principles and Key Advances
Core Concept:
mRNA Velocity relies on mathematical modeling of transcriptional dynamics, simulating transcription activation, splicing, and degradation processes. By iteratively optimizing gene-specific rate parameters (transcription rate β, splicing rate γ, degradation rate η), it predicts the temporal derivatives of cell states.
2025 Innovations:
- Multi-Omics Integration: Algorithms like PhyloVelo combine single-cell lineage tracing data to identify monotonically changing genes and construct high-precision velocity fields.
- Experimental Validation: Metabolic labeling techniques (e.g., scNT-seq) distinguish nascent and mature mRNA, enhancing dynamic prediction accuracy.
- Quantum Computing: IBM’s quantum algorithms optimize energy state simulations of CRISPR-vector complexes, reducing RNA velocity modeling errors to <0.1Å.
- AI-Driven Calibration: DeepMind’s AlphaMissense v2.0 integrates metabolomic data to predict gene-editing off-target effects, lowering toxicity risks by 30%.
II. Core Applications in Developmental Biology
- Embryonic Development and Organogenesis
- Mouse Hippocampus Development: RNA velocity identifies critical time windows for neural stem cell differentiation into neurons, revealing transcription switches like Sox2→Neurod1.
- Human Heart Morphogenesis: scNT-seq tracks the synthesis-degradation balance of sarcomere proteins (e.g., TNNI3) in cardiomyocyte differentiation, predicting intervention windows for congenital heart defects.
- Stem Cell Fate Regulation
- Embryonic Stem Cell Pluripotency Exit: PhyloVelo quantifies OCT4/NANOG expression decay rates to improve reprogramming efficiency.
- Induced Pluripotent Stem Cells (iPSCs): Dynamic models identify epigenetic barrier genes (e.g., DNMT3A), guiding small-molecule inhibitor combinations.
- Tissue Regeneration and Repair
- Zebrafish Tail Fin Regeneration: RNA velocity uncovers pulsed activation of the Wnt/β-catenin pathway, offering time-dependent therapeutic targets.
- Human Liver Regeneration: Spatial transcriptomics maps dynamic HNF4α gradients in liver lobules, optimizing post-transplant regeneration strategies.
III. Disease Mechanisms and Precision Medicine
- Cancer Evolution and Heterogeneity
- Tumor Microenvironment (TME): scNT-seq tracks M1→M2 polarization trajectories in tumor-associated macrophages (TAMs), revealing PD-L1’s temporal regulation.
- Metastasis Prediction: PhyloVelo analyzes epithelial-mesenchymal transition (EMT) speeds in circulating tumor cells (CTCs) to predict organotropism (e.g., bone vs. liver).
- Neurodegenerative Diseases
- Alzheimer’s Disease (AD): RNA velocity quantifies transcriptional delays in Tau phosphorylation-related genes (e.g., MAPT), pinpointing disease progression timelines.
- Parkinson’s Disease (PD): Multi-omics models link LRRK2 mutations to mitochondrial mRNA splicing defects, guiding CRISPR base editing.
- Immune and Autoimmune Disorders
- T-Cell Exhaustion: Dynamic models resolve TOX transcription factor accumulation rates in CAR-T therapy, enabling intermittent activation strategies.
- Rheumatoid Arthritis (RA): Metabolomics-integrated models predict feedback oscillations in the IL-6/JAK-STAT pathway, optimizing drug dosing schedules.
IV. Challenges and Solutions (2025 Update)
- Data Noise and Model Limitations
- Gene Independence Assumption: Traditional models overlook gene regulatory networks, leading to trajectory misinterpretation.
- Breakthrough: Graph Neural Networks (GNNs) map gene-protein-metabolite interactions, improving leukemia subtyping accuracy.
- Experimental-Computational Validation
- Metabolic Labeling: scNT-seq with 4sU labeling validates nascent mRNA predictions with <5% error.
- Optogenetic Control: Light-activated CRISPR systems (e.g., PA-Cas9) adjust gene expression in real time to validate trajectory interventions.
- Clinical Translation Barriers
- Personalized Velocity Fields: Patient-specific iPSC data predict drug response time windows (e.g., chemotherapy sensitivity peaks).
- Ethics and Privacy: Blockchain-based GET Matrix anonymizes data and enables reversible tracking, aligning with FDA AI review frameworks.
V. Future Prospects and Interdisciplinary Synergy
- Spatiotemporal Multi-Omics Integration
- Spatial RNA Velocity: Slide-seq v3 maps 3D morphogen gradients (e.g., BMP4) during embryogenesis.
- Single-Cell Epigenetic Velocity: ATAC-seq velocity predicts chromatin accessibility changes ahead of gene expression, identifying differentiation decision points.
- Therapeutic Paradigm Shifts
- Dynamic Gene Therapy: mRNA vectors with optogenetic CRISPR systems edit disease genes (e.g., Huntington’s HTT) based on RNA velocity timelines.
- AI-Patient Digital Twins: Novartis’ virtual trial platforms replace 30% of in vivo studies, accelerating cancer vaccine development.
- Global Collaborations
- Multi-Omics Biobanks: A Sino-EU-US database (2M+ samples) prioritizes RNA velocity targets (TPS) for rare disease therapies.
- Synthetic Biology: Engineered microbes with AI-optimized terpenoid pathways dynamically adjust drug precursor yields, cutting costs by 60%.
Conclusion
mRNA Velocity has evolved from a research tool to a clinical decision engine, offering:
- Temporal Resolution: Unlocks continuous cell fate dynamics beyond static snapshots.
- Intervention Timing: Shifts focus from “whether to treat” to “when to treat” (e.g., cancer immunotherapy activation windows).
- Cross-Scale Integration: Bridges molecular mechanisms (e.g., splicing kinetics) and macro-phenotypes (e.g., organ regeneration).
China’s Role: BGI Group (OIA platform) and Xiamen University (PhyloVelo algorithm) lead in dynamic modeling and data integration, positioning China as a global hub for RNA velocity innovation.
Data sourced from public references. For collaboration or domain inquiries, contact: chuanchuan810@gmail.com