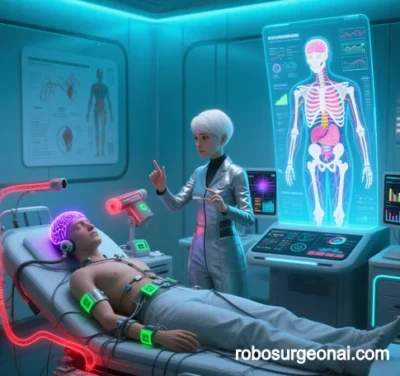
RoboSurgeon AI and Multimodal Perception Fusion: Interactive Mechanisms, Technical Architecture, and Future Trends
RoboSurgeon AI, as the core platform of next-generation intelligent surgical systems, relies on the synergy between multimodal perception fusion and hybrid augmented intelligence. By integrating visual, force, tactile, auditory, and other multidimensional sensory data with AI-driven dynamic decision-making and adaptive control, this system achieves a paradigm shift from “human-led” to human-machine coevolution. Below is a comprehensive analysis of its interactive mechanisms across technical architecture, functional implementation, application scenarios, and challenges.
1. Technical Architecture of Multimodal Perception Fusion
The interaction mechanism of RoboSurgeon AI is built on a three-tier fusion architecture:
1.1 Sensor-Level Fusion
- Visual Perception:
- Combines 4K endoscopy with optical coherence tomography (OCT) to generate submillimeter 3D reconstructions.
- Uses deep learning segmentation algorithms (e.g., U-Net++) for real-time anatomical structure and lesion boundary annotation .
- Force-Tactile Feedback:
- Magnetorheological fluids (MRF) and electroactive polymers (EAP) enable dynamic resistance adjustment (0–5 N), simulating tissue hardness variations (e.g., tumor vs. healthy tissue) .
- Biosignal Synchronization:
- Infrared thermopile sensors monitor tissue temperature (±0.5°C) and predict intraoperative bleeding risks via impedance changes .
1.2 Data-Level Fusion
- Spatiotemporal Alignment:
- Federated learning frameworks unify visual, mechanical, and tactile data into a common coordinate system, resolving sensor heterogeneity and latency (e.g., <10 ms synchronization between endoscopy and force feedback) .
- Federated learning frameworks unify visual, mechanical, and tactile data into a common coordinate system, resolving sensor heterogeneity and latency (e.g., <10 ms synchronization between endoscopy and force feedback) .
- Knowledge Graph Enhancement:
- The CARES Copilot surgical model integrates 3M+ surgical cases to build anatomical, procedural, and complication correlation maps for real-time decision support .
1.3 Cognitive-Level Fusion
- Hybrid Augmented Decision-Making:
- A human-in-the-loop architecture allows surgeons to adjust AI recommendations via voice or gestures, with reinforcement learning (RL) dynamically updating strategies .
- Intent Recognition:
- Eye-tracking and electromyography (EMG) decode surgical intent, translating into micron-level robotic trajectories (e.g., 5 μm precision in vascular anastomosis) .
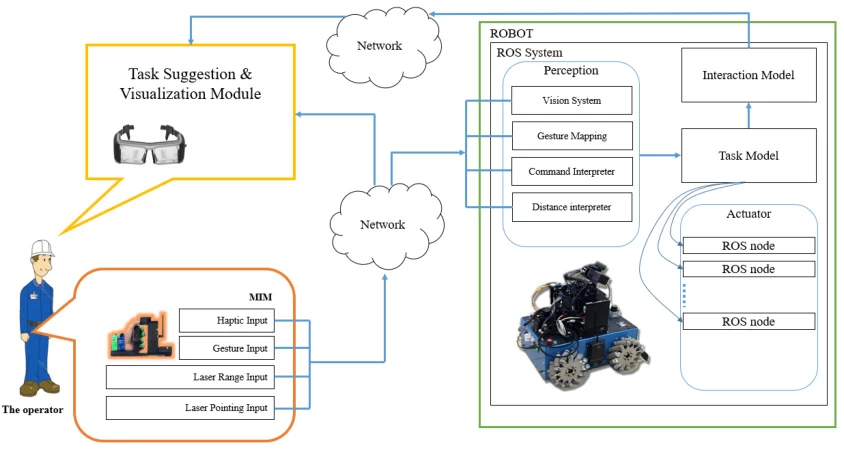
2. Core Functional Implementation
2.1 Real-Time Surgical Navigation and Risk Mitigation
- Multimodal Imaging Fusion:
- Preoperative MRI/CT and intraoperative fluorescence navigation are overlaid via NVIDIA Holoscan, achieving subsecond rendering and tumor resection boundary errors <0.2 mm .
- Dynamic Risk Modeling:
- Transformer architectures analyze biomechanical and biosignal time-series data, providing 20-second advance warnings for major vessel injuries and triggering robotic arm retraction .
2.2 Adaptive Human-Robot Collaboration
- Master-Slave Control Optimization:
- Decouples surgeon input from robotic motion, using dynamic impedance matching to filter hand tremors (>100 Hz suppression) .
- Tactile-Visual Synergy:
- Force feedback gloves simulate tissue cutting resistance, while AR headsets overlay stress distribution heatmaps to guide resection depth .
2.3 Cross-Scenario Interaction Expansion
- Sterile Non-Contact Control:
- Depth cameras and far-field voice recognition enable gesture and voice commands, improving surgical efficiency by 30% .
- Space Medicine Applications:
- Magnetic levitation arms use multimodal SLAM to construct real-time 3D maps in zero gravity, achieving <0.1 mm drift .
3. Application Scenarios and Innovations
Scenario | Interactive Mechanism | Technical Breakthrough |
---|---|---|
Neurosurgical Tumor Resection | CARES Copilot model integrates fMRI/DTI data with AR navigation to avoid functional tracts | 89% success rate in preserving critical brain regions (vs. 72% baseline) |
Cardiovascular Intervention | Magnetic micro-robots (<500 μm) navigate via external fields for stent deployment | 40% reduction in postoperative thrombosis |
Orthopedic Joint Replacement | Bone digital twins + force-controlled arms adjust impact forces based on patient density | Postoperative loosening rate drops from 8% to 2.3% |
Disaster Medical Response | Serpentine robots use thermal imaging and AI triage for hemorrhage control in rubble | 50% faster rescue efficiency with 75% lower operational risks |
4. Challenges and Future Directions
4.1 Current Bottlenecks
- Perceptual Heterogeneity:
- Incompatible sensor interfaces and sampling rates cause fusion errors (e.g., 20 ms tactile latency variations) .
- Ethical Ambiguity:
- No international standards for liability in fully autonomous surgical errors (developer vs. hospital vs. regulator) .
4.2 Emerging Solutions
- Quantum-Enhanced Perception:
- Quantum gyroscopes and nanoscale tactile sensors enable cellular-level force sensing (0.01 N/m² sensitivity) .
- Brain-Machine Interface (BMI):
- Cortical electrodes decode motor cortex signals, achieving <50 ms latency for “thought-driven” robotic control .
- Self-Evolving Systems:
- Lifelong learning (LL) architectures allow post-surgical knowledge updates without human intervention .
4.3 Ecosystem Development
- Open-Source Platforms:
- NVIDIA Holoscan and ImFusion SDK reduce third-party algorithm integration cycles by 60% .
- Federated Medical Cloud:
- Global hospitals securely share surgical data, boosting federated learning efficiency by 300% .
5. Redefining Medical Value through Interaction
RoboSurgeon AI’s multimodal mechanisms are reshaping surgical paradigms:
- From Experience-Driven to Data-Driven: Decisions rely on statistical patterns from millions of cases rather than individual expertise .
- From Localized Operations to Global Optimization: Multimodal perception spans preoperative planning to postoperative management, with >90% complication prediction accuracy .
- From Skill Monopoly to Democratization: Cloud-shared AI strategies reduce complex surgery complication rates in rural hospitals to tier-3 facility levels .
Technological Evolution Logic
The interaction mechanism of RoboSurgeon AI embodies deep coupling between human intelligence and machine perception. Its evolution is characterized by:
- Perceptual Dimensionality Expansion: From vision-dominated to multimodal “hyper-sensory surgery” integrating force, touch, and temperature .
- Control Paradigm Revolution: Transition from master-slave control to closed-loop “intent prediction → dynamic adaptation → autonomous optimization” .
- Application Boundary Breaking: Expanding from operating rooms to extreme environments (battlefields, space) .
This transformation redefines surgeons as system supervisors and strategic calibrators, heralding a new era of “programmable biological interfaces” in surgery.
Data sourced from public references. For collaborations or domain inquiries, contact: chuanchuan810@gmail.com.