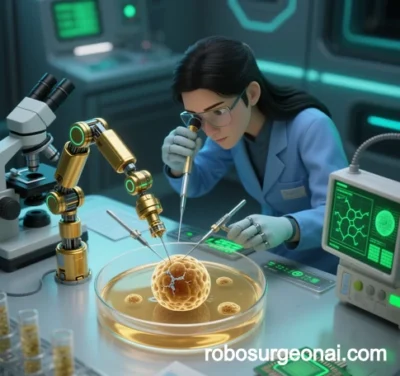
RoboSurgeon AI: Frontiers of Surgical Robotics Technology
1. Technical Definition
RoboSurgeon AI represents a transformative surgical system driven by the integration of artificial intelligence and robotics, advancing surgery toward intelligence, precision, and autonomy. This paradigm shift is structured across four dimensions: technical architecture, core innovations, application scenarios, and ethical challenges.
2. Core Innovations
2.1 Intelligent Perception and Decision-Making
- Multimodal Sensory Fusion:
- Haptic Feedback: Magnetorheological fluids or electroactive polymers (EAP) enable dynamic resistance modulation (0–5 N) to differentiate tissue hardness (e.g., tumors vs. healthy tissue).
- 3D Vision Enhancement: Combines deep learning-based real-time image segmentation (e.g., U-Net++) with 4K fluorescence imaging and optical coherence tomography (OCT) for submillimeter anatomical recognition.
- Biosignal Monitoring: Embedded infrared thermopile sensors track tissue temperature (±0.5°C) and predict intraoperative bleeding risks via conductivity changes.
- AI-Driven Real-Time Decision Systems:
- Surgical Scene Understanding: Visual Question Answering (VQA) models analyze intraoperative images to generate structured procedural guidance (e.g., “Prioritize ligation of the left hepatic artery”).
- Risk Prediction: Transformer-based time-series analysis predicts major vessel injury risks 20 seconds in advance, triggering robotic arm retraction.
- Adaptive Strategy Optimization: Reinforcement learning (RL) extracts optimal surgical patterns from million-scale video datasets to adjust instrument trajectories (e.g., avoiding nerve bundle overstretching).
2.2 Precision Execution and Autonomy
- Nanoscale Manipulation:
- Magnetic Micro-Robots: Sub-500 μm magnetic forceps navigate via external fields for targeted drug delivery or tissue sampling, bypassing traditional instrument limitations.
- Laser Ablation: Femtosecond laser pulses guided by AI path planning achieve subcellular tumor margin ablation while preserving healthy tissue.
- Autonomous Surgical Modules:
- Structured Task Automation: RL-trained AI completes standardized steps (e.g., intestinal anastomosis) with <0.3% error under human supervision.
- Dynamic Environment Adaptation: SLAM (Simultaneous Localization and Mapping) generates real-time 3D maps to address organ displacement or bleeding-induced visual obstructions.
- Human-Robot Collaboration:
- Intent Recognition: Eye-tracking and electromyography (EMG) convert surgeons’ gaze and micro-movements into robotic arm displacements.
- Bidirectional Force Feedback: Surgeons perceive robotic end-effector forces while their input is constrained within safety thresholds.
3. Cross-Disciplinary Integration
- Digital Twins and Virtual Training:
- Patient-Specific Modeling: Preoperative CT/MRI data create organ digital twins, simulating tool-tissue interactions via finite-element analysis.
- VR Skill Assessment: AI simulators evaluate trainees’ motion trajectories, force profiles, and decision latency (e.g., “22% excess force during gallbladder dissection”).
- Genomics-Driven Precision Surgery:
- In Situ Molecular Diagnostics: Intraoperative Raman spectroscopy and AI identify tumor mutations (e.g., KRAS variants) to dynamically adjust resection margins.
- Immunomodulation: Robotic arms deploy PD-1 inhibitor nanoparticles post-resection to suppress recurrence.
- Edge Computing and 5G Remote Collaboration:
- Low-Latency Communication: 5G networks enable transcontinental surgeries with <50 ms command latency (e.g., remote pancreatectomy).
- Federated Learning: Hospitals train encrypted local models, sharing global surgical strategies to resolve data silos and privacy conflicts.
4. Ethical Challenges and Standardization
- Accountability Challenges:
- Decision Traceability: “Black box” systems log AI decision logic for post-incident audits (e.g., misjudged vessel properties causing hemorrhage).
- Legal Liability: Debates center on accountability for fully autonomous procedures—algorithm developers, hospitals, or regulators.
- Risk Mitigation:
- Anti-Tampering Protocols: Blockchain validation and quantum encryption prevent malicious intraoperative image manipulation.
- Fail-Safe Mechanisms: Dual-redundant control systems switch robotic arms to passive mode during power/network failures.
- Ethical Frameworks:
- Informed Consent: Patients must be explicitly informed of AI involvement (e.g., “30% of steps autonomously executed”).
- Equity Assurance: Government subsidies reduce costs of robotic surgeries to prevent healthcare disparities.
5. Future Technological Roadmap
- Brain-Computer Interfaces (BCI): Cortical electrodes capture surgeons’ motor intent for “intent-driven” robotic control.
- Self-Evolving Systems: Lifelong learning (LL) architectures enable AI models to autonomously update post-surgery without human intervention.
- Biohybrid Robotics: Integration of living cells (e.g., cardiomyocyte-driven micro-actuators) for biocompatible tissue interactions.
6. Technological Evolution
RoboSurgeon AI embodies three paradigm shifts:
- Perceptual Expansion: Transition from manual observation to multimodal biosignal fusion (“hyper-sensory surgery”).
- Decision-Making Restructuring: Human intuition augmented by “human-in-the-loop” hybrid intelligence.
- Precision Revolution: Nanoscale manipulation and autonomous control transcend physical limits (e.g., single-cell-level operations).
This evolution redefines surgeons as system supervisors and strategists, realizing the ultimate vision of precision medicine.
Data sourced from public references. For collaborations or domain inquiries, contact: chuanchuan810@gmail.com.