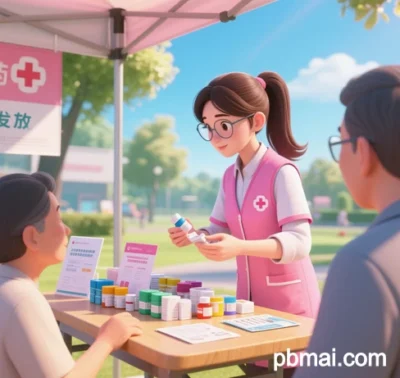
AI-Driven Pharmacy Benefit Management (PBM): Trends and Integration (2025 Perspective)
Pharmacy Benefit Management (PBM), a critical component of healthcare cost control and medication optimization, is undergoing a transformative shift from passive management to predictive intervention through AI integration. Below are key technological trends and implementation pathways:
I. Industry Challenges and AI Solutions
1. Addressing Cost Escalation
- Drug Price Inflation: AI-powered dynamic pricing systems recommend generic alternatives and optimize pharmacy network selection (e.g., CVS vs. Walmart cost analysis) to reduce out-of-pocket expenses.
- Waste Reduction: AI analyzes medication adherence patterns to identify unused prescriptions, curbing annual drug waste.
2. Enhancing Efficiency
- Automated Prior Authorization: AI reduces approval times by matching clinical guidelines with insurance policies in real time.
- Data Integration: Federated learning frameworks unify electronic health records (EHRs), genetic data, and pharmacy inventories to create comprehensive patient profiles.
II. Core AI Applications in PBM
- Automated Prescription Review:
NLP and knowledge graphs flag contraindications, reducing prescription errors. - Dynamic Formulary Optimization:
Reinforcement learning evaluates drug efficacy and cost-effectiveness to lower annual per-patient spending. - Adherence Prediction:
Time-series models analyze wearable data to predict chronic disease medication discontinuation risks. - Supply Chain Forecasting:
Graph neural networks predict regional medication demand, improving inventory turnover.
Case Studies:
- CVS Health’s AI Audit Engine: Leverages historical prescription data to detect dosing errors and drug interactions, reducing hospital readmissions.
- OptumRx Cost Predictor: Quantum computing optimizes Medicare drug cost-sharing models, lowering high out-of-pocket burdens.
III. Technology Integration Pathways
1. Data Infrastructure
- Privacy-Preserving Computation: Homomorphic encryption enables secure cross-institutional data sharing.
- Multimodal Knowledge Bases: CLIP models align drug labels, clinical research, and real-world evidence for dynamic rule updates.
2. Decision-Making Systems
- Hybrid Reasoning: Combines FDA-encoded rules with deep learning for personalized treatment predictions.
- Counterfactual Analysis: GANs simulate medication substitution outcomes to support payer-provider negotiations.
3. User Interfaces
- Generative AI Assistants: GPT-4-driven virtual pharmacists resolve insurance queries, reducing call center load.
- AR Medication Guides: HoloLens 2 displays 3D molecular structures and dosing schedules to improve patient understanding.
IV. Challenges and Ethical Considerations
1. Technical Limitations
- Algorithmic Bias: Overreliance on commercial insurance data skews recommendations for Medicaid populations.
- Regulatory Gaps: FDA transparency requirements conflict with opaque AI models.
2. Ethical Dilemmas
- Conflict of Interest: AI-driven preferential drug recommendations may violate antitrust laws.
- Liability Allocation: Ambiguity in assigning blame for AI-caused authorization denials.
V. Industry Evolution
1. Emerging Services
- AI Auditing: Third-party fairness assessments of PBM algorithms.
- Collaborative Learning: Federated platforms enable rural hospitals to co-develop AI models.
2. Innovative Insurance Models
- Outcome-Based Contracts: Drug manufacturers and PBMs share risks/rewards based on AI-predicted efficacy.
- Dynamic Pricing: Digital twins simulate medication trajectories to refine premium calculations.
Conclusion: From Cost Management to Health Partnership
AI-PBM integration is redefining healthcare by:
- Personalized Care: Tailoring treatments (e.g., metformin response prediction via gut microbiome analysis).
- Value Demonstration: Helping pharma prove real-world drug efficacy beyond pricing wars.
- Preventive Resource Allocation: Predicting regional disease burdens for proactive interventions (e.g., AI-driven vaccine distribution).
The ultimate challenge lies in building trustworthy AI-PBM ecosystems, balancing algorithmic performance, third-party oversight, and equity. By 2030, advances in quantum computing and causal inference may position PBMs as fully autonomous healthcare optimizers.
Data sourced from publicly available references. For collaborations or domain inquiries, contact: chuanchuan810@gmail.com.