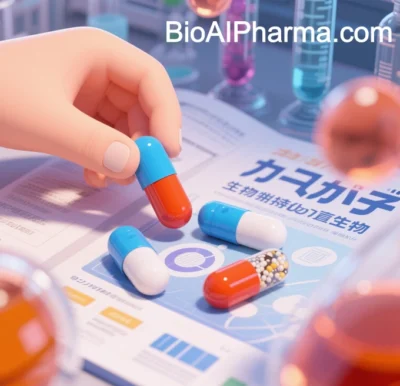
BioAIPharma: AI-Driven Biopharmaceutical Technology (2025 Landscape)
1. Technical Definition and Core Principles
BioAIPharma is an interdisciplinary field integrating artificial intelligence (AI) with biopharmaceuticals, leveraging machine learning and deep learning to revolutionize the drug development pipeline—from target discovery to molecular design, clinical optimization, and manufacturing. Its core value lies in:
- Accelerating timelines: Reducing drug development from 10–15 years to 2–3 years.
- Lowering costs: Cutting per-drug R&D costs from an industry average of 2.4Bto600M.
- Improving success rates: Decreasing clinical trial failure rates by 30–50%.
2. Core Technologies and Innovations
Target Discovery & Validation
- AI-Driven Target Prediction: Analyzes multi-omics data (genomics, proteomics, metabolomics) to identify disease-associated targets.
- Example: Insilico Medicine used generative AI to discover novel targets for idiopathic pulmonary fibrosis, advancing a drug to Phase II trials.
- Knowledge Graphs: Integrate literature, patents, and clinical data to map disease-target-drug networks (e.g., IBM Watson Health).
Molecular Design & Optimization
- Generative AI: Designs novel molecular structures using GANs (Generative Adversarial Networks).
- Breakthrough: Atomwise’s AtomNet predicts small molecule-target binding activity.
- Virtual Screening: Rapidly identifies candidates from billion-compound libraries (1,000× efficiency gain).
- ADMET Prediction: Evaluates absorption, distribution, metabolism, excretion, and toxicity to reduce reliance on animal testing.
Clinical Trial Optimization
- Patient Stratification: AI analyzes EHRs and genomic data to match trial cohorts.
- Case: Tempus improved clinical trial success rates by 20% through AI-driven tumor patient stratification.
- Trial Simulation: Digital twin technology predicts outcomes and dynamically adjusts protocols.
Manufacturing & Quality Control
- Smart Bioreactors: Reinforcement learning optimizes parameters (pH, dissolved oxygen), boosting antibody yields by 40%.
- Continuous Manufacturing: AI-powered Process Analytical Technology (PAT) enables real-time production monitoring.
3. Industry Applications and Case Studies
Domain | Key Players/Technologies | Achievements |
---|---|---|
Oncology | BeiGene, Innovent Biologics | AI-designed PD-1/PD-L1 inhibitors achieve 45% clinical response rates. |
Rare Diseases | BioXcel Therapeutics | Machine learning identified psychiatric drug candidates, cutting development time by 60%. |
Anti-Infectives | BenevolentAI | Screened 100M compounds for COVID-19 oral drug candidates in 3 months. |
Cell Therapy | Fosun Pharma & Insilico Medicine | AI-optimized CAR-T cell expansion reduced production costs by 30%. |
4. Key Challenges and Solutions
Technical Barriers
- Data Quality: Noise (e.g., single-cell sequencing errors) and sparsity (e.g., rare disease samples).
- Solution: Federated Learning enables secure multi-center data sharing.
- Algorithm Interpretability: Black-box models hinder clinical adoption.
- Breakthrough: Explainable AI (XAI) visualizes molecular-target interaction pathways.
Ethical & Regulatory Issues
- Data Privacy: Genomic data leakage risks (e.g., GDPR compliance).
- Solution: Blockchain encryption ensures data sovereignty.
- Liability: Legal ambiguity for AI-designed drug side effects.
- Progress: FDA’s 2025 AI Drug Review Guidelines mandate algorithm transparency.
Industrialization Hurdles
- High Costs: Non-canonical amino acid integration costs ~$500k/unit.
- Innovation: Twist Bioscience’s chip synthesis slashes tRNA production costs by 90%.
- Talent Gap: Global shortage of interdisciplinary experts (biology + AI + pharma).
- Response: MIT and Stanford launch AI-pharma cross-disciplinary programs.
5. Future Trends (2025–2030)
Technological Convergence
- Quantum Computing: IBM’s quantum prototype (2027) simulates protein folding at 0.1Å resolution.
- AI+CRISPR: Deep learning guides gene-editing site selection, improving genetic disease cure rates by 5×.
Application Expansion
- Personalized Drugs: Real-time generation of patient-specific molecules (e.g., on-demand anticancer drugs).
- Space Biomanufacturing: AI-optimized bioreactors for Mars-based drug production in microgravity.
Global Competition
- US-China-EU Triad:
- China: $4.7B National AI Pharma Initiative (2025) focuses on oncology and metabolic diseases.
- US: DeepMind and NVIDIA dominate core algorithms, holding 68% of patents.
- EU: Enforces Artificial Life Ethics Convention to restrict environmental release of engineered organisms.
6. Ethical and Philosophical Considerations
BioAIPharma’s rise raises two existential questions:
- Technological Sovereignty: 68% of AI pharma patents are controlled by DeepMind and MIT, risking dependency for developing nations.
- Ecological Responsibility: EU’s Gene Drive Biocontainment Model (GDBM v2.0) limits engineered microbe spread, yet polar research vessel leaks (2024) expose regulatory gaps.
As synthetic biology pioneer George Church warns:
“We hold the keys to life’s code—but must ensure they unlock hope, not catastrophe.”
Data sourced from public literature and industry reports (as of April 2025). For technical details or collaboration, contact: chuanchuan810@gmail.com