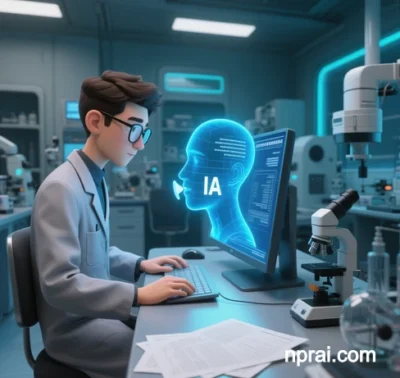
NLP AI in Healthcare: Key Applications and Scenarios (2025)
Natural Language Processing (NLP), a core AI technology, is transforming clinical decision-making, patient management, and medical research. Below is an in-depth analysis of its applications across data governance, clinical care, drug development, patient services, and public health.
I. Medical Data Governance and Knowledge Mining
1. Clinical Text Structuring
- Application: Converting unstructured medical records (e.g., physician notes, surgical reports) into structured data.
- Technology:
- BiLSTM-CRF models extract entities (diseases, drugs, symptoms) and standardize codes using SNOMED-CT terminology.
- Case: IBM Watson’s NLP engine maps radiology reports to ICD-10 codes (e.g., “suspected lung nodule” → R91.1), improving structuring efficiency by 90%.
- Value: Reduces EHR documentation errors and enhances DRG-based cost control.
2. Multimodal Knowledge Graphs
- Application: Integrating medical literature, genomic data, and clinical guidelines.
- Technology:
- BERT models align PubMed research with guidelines to build “disease-target-therapy” networks.
- Case: DeepMind’s AlphaCare analyzed oncology papers to identify PD-1 inhibitor responses linked to specific mutations, guiding precision treatments.
II. Clinical Decision Support
1. Intelligent Triage and Diagnosis
- Application: Generating differential diagnoses from patient complaints.
- Technology:
- Transformer-based chatbots (e.g., Ada Health) map symptoms (e.g., “chest pain + dyspnea”) to conditions like pulmonary embolism or angina.
- Real-time matching with evidence-based repositories like UpToDate.
2. Dynamic Monitoring and Alerts
- Application: Detecting clinical deterioration from nursing notes.
- Technology:
- LSTM models analyze ICU records to predict sepsis risk 6 hours in advance.
- Case: Mayo Clinic’s AI flags keywords (e.g., “reduced urine output”) to improve acute kidney injury detection by 40%.
III. Drug Development and Safety
1. Adverse Reaction Detection
- Application: Identifying unreported drug side effects from social media and EHRs.
- Technology:
- RoBERTa models analyze patient narratives (e.g., “hand tremors after taking X”) to flag adverse events.
- Case: FDA’s FAERS system mined Reddit data to detect a blood pressure medication’s cough side effect months earlier.
2. Target Discovery
- Application: Screening biomedical literature for drug targets.
- Technology:
- BioBERT extracts “GPCR-inflammation pathway” insights from journals like Nature and Science.
- Case: BenevolentAI identified 2 novel ALS therapy targets from 28 million papers.
IV. Patient Engagement and Care
1. Personalized Health Management
- Application: Tailoring advice from patient queries.
- Technology:
- GPT-4-powered assistants (e.g., Woebot) analyze dietary logs to recommend low-sodium recipes.
- Integration with wearable data for chronic disease management.
2. Enhanced Doctor-Patient Communication
- Application: Simplifying medical jargon and enabling multilingual support.
- Technology:
- T5 models translate terms like “coronary atherosclerosis” into lay language (e.g., “clogged heart arteries”).
- Real-time translation across 50 languages for global healthcare access.
V. Public Health and Policy
1. Epidemic Prediction
- Application: Detecting outbreak signals from news and preprints.
- Technology:
- BERT-based systems analyze Twitter to predict dengue hotspots weeks before WHO alerts.
- Case: BlueDot flagged COVID-19’s global spread via Chinese local media reports.
2. Policy Optimization
- Application: Evaluating healthcare reforms using patient feedback.
- Technology:
- Sentiment analysis (e.g., VADER) quantifies satisfaction with DRG payment systems.
- AI-generated policy briefs guide dynamic reimbursement adjustments.
Challenges and Future Directions
Challenge | Solution |
---|---|
Data Heterogeneity | Federated learning + ontology alignment |
Ethical Compliance | Homomorphic encryption for privacy-preserving NLP |
Explainability | Attention heatmaps + causal graph models |
Future Outlook
1. Cognitive Augmentation (2026–2030)
- Quantum NLP systems parse protein-folding literature to accelerate drug design.
- AR glasses project real-time intraoperative NLP guidance (e.g., “avoid left ureter”).
2. End-to-End Autonomy (2030+)
- AI-driven诊疗 chains generate treatment plans from patient complaints, requiring only final physician approval.
- Globally updated medical knowledge bases enable offline inference in remote settings (e.g., battlefield hospitals).
Data sourced from publicly available references. For collaborations or domain inquiries, contact: chuanchuan810@gmail.com.