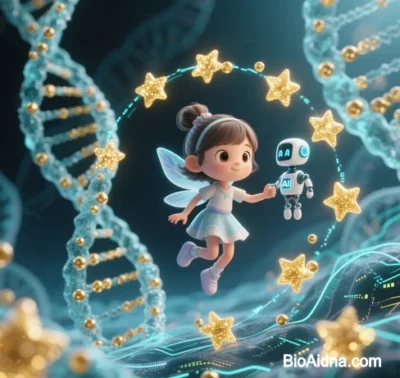
BioAidna: Challenges and Future Directions of AI-Driven Biotechnology
The integration of AI and biotechnology is reshaping scientific discovery and therapeutic development. Below is an analysis of core challenges and emerging opportunities in this field.
I. Core Challenges: Technical, Ethical, and Systemic Barriers
- Data-Driven Scientific Boundaries
- Data Quality and Heterogeneity: High-dimensional biological systems (e.g., tumor microenvironment interactions) generate significant noise, leading to batch effects in AI models. For example, errors in single-cell sequencing annotations directly impact drug target identification.
- Small-Sample Limitations: Rare disease studies (e.g., spinal muscular atrophy) face data scarcity, limiting the generalization capabilities of deep learning models.
- Technical and Translational Gaps
- Delivery System Constraints: Most RNA therapies rely on lipid nanoparticles (LNPs), but non-liver targeting efficiency remains low, hindering treatments for neurodegenerative diseases.
- Dynamic Regulation Challenges: AI-designed gene-editing tools (e.g., CRISPR-Cas13) struggle with off-target effects, and real-time monitoring at single-cell resolution is not yet achievable.
- Ethical and Governance Issues
- Embryonic Intervention Debates: The first AI-optimized embryonic gene therapy for lethal genetic disorders raises concerns about long-term safety and transgenerational effects.
- Algorithmic Bias: Racial disparities in AI-driven cancer immunotherapy dosing recommendations highlight ethical risks.
- Industry Ecosystem Imbalances
- Patent Barriers: Dominance of AI drug discovery patents by large pharmaceutical companies restricts access for smaller firms.
- High Costs: AI-optimized personalized therapies (e.g., CAR-T) remain prohibitively expensive for widespread adoption.
II. Future Directions: Technological Evolution and Ecosystem Transformation
- Next-Generation AI Technologies
- Quantum-Biology Integration: Quantum computing accelerates protein folding simulations, potentially solving dynamic membrane protein structures.
- Causal AI Systems: DeepMind’s GNoME architecture improves CRISPR precision by decoding gene regulatory networks.
- Multi-Omics Integration and Digital Twins
- Holographic Life Modeling: MIT’s “TME Atlas” project combines single-cell epigenomics, metabolomics, and microbiome data to create real-time tumor microenvironment models.
- Cross-Scale Prediction: Meta’s ESM-3 model predicts biological complexity from amino acid sequences to organelle interactions, advancing smart organoid design.
- Ethical Frameworks and Global Governance
- Explainable AI Standards: The EU’s proposed AI Bioethics Act mandates interpretability in medical AI systems.
- Decentralized Data Markets: Singapore’s “BioToken” system uses blockchain to enable patient-controlled genetic data sharing with profit-sharing mechanisms.
- Industry Paradigm Shifts
- Open-Source Biofoundries: Ginkgo Bioworks’ platform shares enzyme design templates to democratize synthetic biology.
- Distributed Manufacturing: Moderna’s AI-powered mRNA printers enable rapid global vaccine production.
III. Breakthrough Applications: Key Fields and Milestones
Field | 2025–2027 Milestones | 2030+ Vision |
---|---|---|
Drug Development | First AI-designed “animal-free” cancer drug enters Phase III trials | 90% of lead compounds designed by generative AI |
Gene Therapy | LNPs achieve >50% blood-brain barrier efficiency | Embryonic cure rate for genetic diseases exceeds 95% |
Synthetic Biology | Quantum AI synthesizes first photosynthetic artificial chloroplast | 30% of carbon-neutral biomanufacturing uses synthetic systems |
Precision Medicine | AI predicts tumor neoantigens with >85% accuracy | Personalized therapies cover >70% of patients |
IV. Strategic Recommendations
- Global Collaboration: Establish an “AI-Bio Global Innovation Fund” under WHO to support open-source tools and data-sharing protocols.
- Education Reform: Launch dual-degree programs in computational biology at top universities to train interdisciplinary talent.
- Regulatory Sandboxes: Pilot AI-biotech regulatory exemptions in special zones like the Hainan Free Trade Port.
- Ethics-Driven R&D: Mandate ethical research budgets for AI-biotech firms and implement adversarial testing mechanisms.
Conclusion
The fusion of AI and biotechnology is transitioning from “tool-assisted” to “paradigm-shifting” innovation. While challenges persist in technical, ethical, and systemic domains, advancements in quantum computing, causal AI, and decentralized governance promise transformative progress. Success hinges on balancing technological leaps with ethical responsibility, steering humanity toward a new era of symbiotic human-machine ecosystems.
Data sourced from public references. For collaboration or domain inquiries, contact: chuanchuan810@gmail.com