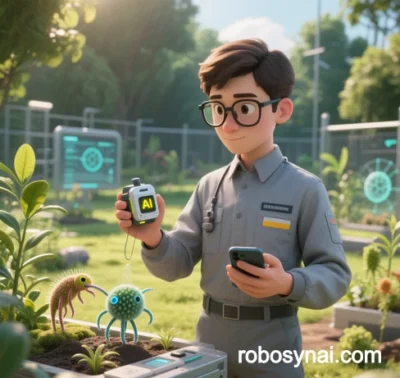
RoboSynAI: The Convergence of AI, Robotics, and Synthetic Biology
RoboSynAI—the deep integration of artificial intelligence (AI), robotics, and synthetic biology—is reshaping industries such as healthcare, manufacturing, energy, and environmental sustainability. This multidisciplinary fusion accelerates innovation through self-evolving systems, intelligent biofactories, and closed-loop sustainable cycles, creating disruptive solutions that transcend the boundaries of living and non-living systems. Below is a systematic exploration of its technical architecture, applications, ethical challenges, and future trajectories.
I. Technical Framework: A Triad of Synergy
RoboSynAI centers on building AI-driven bio-machine hybrid systems, supported by three core pillars:
- AI-Synthetic Biology Engine:
- Protein Design: Tools like AlphaFold 3 predict and optimize interactions between proteins, nucleic acids, and ligands to design novel enzymes or metabolic pathways (e.g., CRISPR-Cas9 variants).
- Cell Factory Programming: Large language models automate microbial pathway optimization via “Design-Build-Test-Learn-Predict” (DBTLP) cycles (e.g., artemisinin biosynthesis).
- Robotics-Biology Interface:
- Nanorobot Delivery: DNA origami robots carry CRISPR components for precision cell repair via magnetic guidance.
- Surgical Robotics Integration: The Da Vinci system integrates AI vision and synthetic biosensors to detect tumor margins and release targeted therapies in real time.
- Sustainable Manufacturing Networks:
- Closed-Loop Biorefining: Engineered cyanobacteria convert CO₂ into bioplastics (PHA), with AI optimizing photobioreactor efficiency.
- Self-Healing Materials: Mycelium composites and 3D-printing robots collaborate to construct structures that dynamically repair environmental damage.
II. Healthcare: From Precision Therapy to Regenerative Medicine
1. Personalized Gene-Cell Therapies
- AI-Optimized Delivery: NVIDIA’s BioNeMo platform trains generative models to predict AAV capsid-HLA affinity, enabling low-immunogenicity vectors (e.g., Spark Therapeutics’ SPK-7001).
- In Vivo CAR-T Factories: Subdermal microrobots deliver CRISPR-Cas12a and mRNA instructions to reprogram T cells into tumor-targeting CAR-T cells.
2. Smart Diagnosis-Treatment Loop
- Liquid Biopsy-AI Decision: Exosome robots capture circulating tumor DNA (ctDNA), with edge computing analyzing mutation profiles to trigger LNP-based siRNA release (e.g., KRAS G12D inhibitors).
- Organ-on-a-Chip Prediction: Microfluidic robots build liver chips to simulate drug metabolism, while AI predicts personalized dosing toxicity (replacing 30% of animal testing).
3. Regenerative Breakthroughs
- Bioprinting Robots: CELLINK’s BIO X6 integrates AI vision and synthetic bioinks to print vascularized cardiac tissue for myocardial infarction repair.
- Mitochondrial Transplants: Magnetically guided nanorobots traverse the blood-brain barrier to deliver engineered mitochondria, reversing Parkinsonian neurodegeneration.
III. Sustainability: From Carbon Neutrality to Circular Economy
1. Carbon-Negative Biomanufacturing
- Synthetic Photosynthesis: CRISPRa-activated Synechococcus fixes CO₂ into biodiesel at gigaton scales, with AI tuning photobioreactors.
- Waste Upcycling: Robots sort municipal waste for synthetic microbial consortia to convert into biodegradable plastics (e.g., PHA), slashing carbon footprints by 70%.
2. Ecological Restoration
- Soil Robots: Autonomous robots deploy synthetic rhizobia to detoxify heavy metals while enhancing nitrogen fixation in contaminated soils.
- Coral Symbionts: Gene-edited zooxanthellae tolerate acidified oceans, with drones reseeding reefs at scale.
3. Energy Revolution
- Microbial Fuel Cells: AI-redesigned Shewanella electron transport chains boost power output fivefold via robotic seabed electrode arrays.
- Hydrogen Production: Cyanobacterial hydrogenases paired with AI-driven photobioreactors enable continuous solar-powered H₂ generation.
IV. Ethical and Governance Challenges
1. Biosafety Risks
- Gene Drive Containment: Suicide gene systems (toxin-antitoxin modules) must be embedded in environmental microbes to prevent horizontal gene transfer.
- AI Model Bias: AlphaFold’s human protein-centric training data may overlook extremophile enzyme diversity.
2. Societal Equity
- Technology Monopolies: Open-source platforms (e.g., Benching Bios’ SynBioCAD) challenge patent barriers by giants like Google DeepMind and Ginkgo Bioworks.
- Global Health Equity: Lyophilized LNPs and solar-powered bioreactors democratize RNA vaccine production for low-income regions.
3. Regulatory Gaps
- Dynamic Adaptation: EU proposals classify AI-synbio products as “emerging risks,” requiring real-time tracking of post-release evolutionary trajectories.
- Cross-Border Governance: WHO and the Convention on Biological Diversity (CBD) jointly draft ethical guidelines for AI-synbio integration.
V. Future Trends: Dawn of Life 3.0
- Self-Evolving Systems:
Phage robots target antibiotic-resistant bacteria, with real-time genomic sequencing informing AI models to adjust lysis strategies. - Human-Machine Symbiosis:
Synthetic bioelectrodes fused with soft robotics restore spinal cord function and augment cognition via neural lace interfaces. - Interstellar Biofactories:
Radiation-resistant synthetic microbes and autonomous robots colonize Mars, producing oxygen and medicines from local resources.
Conclusion
RoboSynAI marks humanity’s transition from modifying life to engineering Life 3.0. Its success hinges on balancing:
- Innovation vs. Biosafety: Embedding “molecular firewalls” in synthetic biology and ensuring AI model transparency.
- Profit vs. Global Good: Patent sharing and decentralized manufacturing to prevent oligopolies.
- Short-Term Gains vs. Long-Term Ethics: Establishing agile global governance coalitions.
As ARK Invest predicts: “By 2030, AI-synbio convergence will redefine over 50% of industrial value chains.” Realizing this vision demands collaboration among scientists, policymakers, philosophers, and the public to ensure technological revolutions serve collective well-being.
Data sourced from publicly available references. For collaborations or domain inquiries, contact: chuanchuan810@gmail.com.
“Robo Syn AI”在医疗领域的潜在含义可能结合了机器人技术(Robo)、协同/合成(Syn)与人工智能(AI),其应用场景和意义可参考以下分析:
1. 核心概念拆解
Robo(机器人):指医疗机器人,如手术机器人、康复机器人或服务机器人15。
Syn(协同/合成):可能指多技术协同(如AI与机器人联合操作)或合成数据(用于AI训练)。
AI(人工智能):涵盖机器学习、深度学习等技术,用于辅助诊断、药物研发等。
2. 医疗领域的可能应用
手术协同系统:AI驱动的机器人辅助外科手术,提升精准度和效率(如达芬奇手术机器人的智能化升级)。
康复与护理:康复机器人通过AI算法个性化调整训练方案,或护理机器人协同医护人员监测患者。
医学影像分析:AI与机器人协作自动化处理影像数据(如CT扫描分析)。
药物研发自动化:机器人实验平台结合AI模型加速化合物筛选。
3. 技术整合的价值
精准医疗:通过机器人执行与AI决策的协同,实现个性化治疗。
效率提升:减少人工操作误差,优化医疗流程(如自动化分诊、药物配送)。
4. 潜在挑战
数据安全与伦理:需确保患者隐私和AI决策的透明度4。
技术成熟度:部分应用仍处于实验阶段,需进一步临床验证。
若该术语指向特定产品或研究,需结合具体上下文进一步确认。