BioAIPharma: In-Depth Analysis
BioAIPharma is a portmanteau of Bio (biology), AI (artificial intelligence), and Pharma (pharmaceuticals), referring to AI-driven biopharmaceutical technologies that accelerate drug discovery and development through machine learning, big data analytics, and computational biology. Below is a systematic breakdown of its technical scope, applications, and industry impact:
I. Core Definition and Technical Scope
- Literal Meaning:
- BioAIPharma = Biology + AI + Pharmaceuticals, emphasizing AI’s role in drug discovery, preclinical research, clinical trials, and manufacturing.
- Technical Essence:
- Data-Driven Drug Design: AI analyzes genomic, proteomic, and compound data to predict drug targets and candidate molecules.
- Virtual Screening & Optimization: Generative AI (e.g., AlphaFold, GNN) designs novel molecules or optimizes drug properties (e.g., solubility, toxicity).
- Intelligent Clinical Trials: AI models predict patient stratification, dosing, and adverse effects to shorten trial timelines.
II. Technical Pathways and Innovations
- AI in Drug Development Workflow:
Stage AI Applications Target Discovery NLP mines literature/databases for novel targets Molecular Design GANs generate novel compound structures Preclinical Testing Deep learning predicts ADMET properties Patient Recruitment Computer vision matches trial criteria - Key Technologies:
- AlphaFold (DeepMind): Predicts protein 3D structures for target validation.
- Insilico Medicine: Designed antifibrotic drug ISM001-055 via generative AI in 11 months.
- BenevolentAI: Repurposed baricitinib for COVID-19 using knowledge graphs.
III. Applications and Case Studies
- Novel Drug Development:
- Case 1: Insilico’s AI-discovered TNIK inhibitor for pulmonary fibrosis (Phase II).
- Case 2: Recursion Pharmaceuticals identified angiogenesis inhibitors for rare brain tumors via AI screening.
- Drug Repurposing:
- Case: BenevolentAI repurposed baricitinib for COVID-19 inflammation 3x faster than traditional methods.
- Personalized Medicine:
- Case: Tempus’s AI platform recommends cancer therapies based on genomic and clinical data.
IV. Challenges and Future Directions
- Key Challenges:
- Data Quality: Fragmented biomedical data hinders model generalization.
- Interdisciplinary Barriers: Collaboration gaps between biologists and AI engineers.
- Regulatory Hurdles: Lack of standards for AI-generated drug validation.
- Future Innovations:
- Multimodal AI: Integrate genomics, imaging, and EHR data for predictive models.
- Automated Labs: AI-driven robots for closed-loop “design-synthesize-test” (e.g., Emerald Cloud Lab).
- Quantum Computing: Simulate molecular dynamics with quantum algorithms (e.g., IBM Quantum).
V. Industry Impact
- Efficiency Revolution:
- Cuts traditional R&D timelines (10-15 years) by 30-50%, saving billions in costs.
- Rare Disease Focus:
- Makes orphan drug development economically viable through data modeling.
- Ecosystem Shift:
- AI-powered Biotechs (e.g., Relay Therapeutics) challenge Big Pharma dominance.
Conclusion
BioAIPharma signifies a paradigm shift from trial-and-error experimentation to predictive drug design. By unlocking the value of biological data through AI, it transforms drug development from labor-intensive to computation-driven. Despite data and regulatory challenges, its potential in target discovery and molecular engineering positions it as a cornerstone of next-gen medical innovation.
If you are interested in purchasing this domain, please contact: chuanchuan810@gmail.com
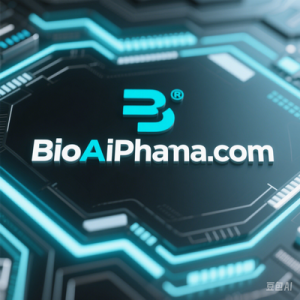