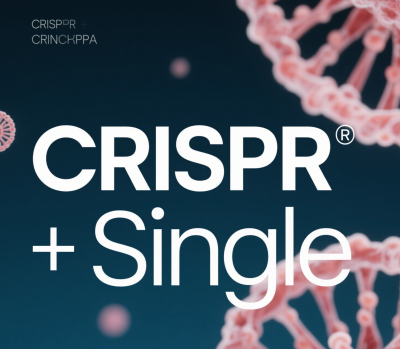
CRISPR + Single-Cell Sequencing: A Deep Dive into Technical Integration
1. Definition and Core Principles
CRISPR + Single-Cell Sequencing is an innovative approach that combines CRISPR gene-editing technology with single-cell resolution omics analysis (e.g., single-cell RNA sequencing). Its goal is to uncover gene functions, cellular heterogeneity, and regulatory networks by enabling high-precision genetic perturbations and multidimensional phenotyping. Key principles include:
- CRISPR: Targets specific genes (e.g., knockout, activation, or inhibition) to induce genetic perturbations.
- Single-Cell Sequencing: Captures genome-wide phenotypic changes (e.g., gene expression, epigenetics, proteomics) at single-cell resolution.
This integration overcomes limitations of traditional CRISPR screens (e.g., analyzing only simple phenotypes like cell proliferation) and enables deep exploration of complex gene regulatory mechanisms.
2. Workflow and Implementation
A typical CRISPR + Single-Cell Sequencing workflow involves:
- sgRNA Library Design: Design CRISPR guide RNA (sgRNA) libraries targeting genes of interest (e.g., genome-wide or pathway-specific).
- Cell Perturbation and Screening:
- Deliver sgRNAs into cells via lentiviral vectors to generate genetically perturbed populations.
- Use droplet microfluidics (e.g., CROP-seq) for high-throughput single-cell capture.
- Single-Cell Sequencing and Data Integration:
- Simultaneously detect sgRNAs (perturbation identifiers) and transcriptomes (phenotypic responses) in single cells.
- Link perturbations to gene expression changes using bioinformatics tools (e.g., Seurat, Monocle).
Technical Variants:
- CROP-seq: Directly associates CRISPR perturbations with single-cell transcriptomes for parallel analysis of thousands of cells.
- Perturb-seq: Integrates single-cell RNA-seq with CRISPR screens to map molecular regulatory networks.
- CRISP-seq: Combines immune cell signaling analysis with single-cell multi-omics.
3. Advantages and Breakthroughs
3.1 Multidimensional Phenotyping
- Cellular Heterogeneity: Reveals divergent responses among cell subpopulations under the same perturbation (e.g., drug-resistant tumor microenvironment subclones).
- Gene Interaction Networks: Identifies synergistic or antagonistic gene relationships via co-expression modules (e.g., schizophrenia risk locus VPS45).
3.2 High-Throughput Functional Screening
- Scalable Perturbations: Analyzes hundreds to thousands of genes in a single experiment (e.g., genome-wide CRISPR screens).
- Dynamic Process Tracking: Maps gene perturbation effects on cell-state transitions (e.g., stem cell differentiation trajectories).
3.3 Precision Target Discovery
- Direct Causality: Matches sgRNAs to phenotypes via single-cell data, avoiding noise in pooled screening.
- Multi-Omics Integration: Platforms like 10x Genomics enable simultaneous detection of transcriptomes, proteomes, and CRISPR perturbations.
4. Key Applications
4.1 Cancer and Immunology
- Drug Resistance: CROP-seq identified MCL1 and BCL2L1 as key resistance genes in NK cell-mediated leukemia targeting.
- Immune Checkpoint Regulation: CRISPR screens uncovered novel PD-1/PD-L1 pathway regulators.
4.2 Neuroscience and Disease Modeling
- Neurodegeneration: Single-cell CRISPR screens in iPSC-derived neurons revealed NQO1’s role in Parkinson’s disease via mitochondrial autophagy defects.
- Psychiatric Disorders: Mapped nonlinear interactions among schizophrenia risk genes.
4.3 Development and Regenerative Medicine
- Cell Fate Decisions: Screened transcription factor libraries to map hematopoietic stem cell differentiation.
- Organoid Optimization: Identified genes maintaining stemness in intestinal organoids.
4.4 Drug Development
- Target Validation: Evaluated cell-specific drug effects using CRISPRa (activation) or CRISPRi (inhibition).
- Combo Therapy Design: Discovered synergistic targets for chemotherapy-immunotherapy combinations.
5. Challenges and Future Directions
5.1 Current Limitations
- Data Noise: Low sensitivity for weakly expressed genes masks subtle perturbation effects.
- sgRNA Misassignment: Droplet sorting or amplification biases cause 5-10% false-positive sgRNA-cell matches.
- Computational Demands: Massive datasets (e.g., million-cell scale) require cloud-based infrastructure.
5.2 Emerging Innovations
- Spatial Multi-Omics Integration: Combines spatial transcriptomics (e.g., DBiT-seq) with CRISPR to study tissue microenvironment dependencies.
- Dynamic Perturbation Tracking: Optogenetic or chemically inducible CRISPR systems for time-resolved analysis.
- AI-Driven Target Prediction: Graph Neural Networks infer gene regulatory networks from single-cell data.
6. Case Studies
Case 1: Leukemia Drug Resistance (Cell Genom, 2023)
- Method: CROP-seq screened 300 genes to analyze NK cell cytotoxicity.
- Discovery: Identified MCL1 and BCL2L1 as resistance genes via mitochondrial apoptosis pathways.
- Application: Guided combination therapy using Venetoclax (BCL2 inhibitor) and NK cell therapy.
Case 2: Neurodegeneration (Nature, 2024)
- Method: Genome-wide CRISPR screens in iPSC-derived neurons with single-cell transcriptomics.
- Discovery: NQO1 loss caused α-synuclein aggregation via defective mitophagy.
- Application: Developed NQO1 activators for preclinical trials.
7. Comparison with Other Technologies
Technology | Strengths | Limitations | Use Cases |
---|---|---|---|
Traditional CRISPR Screens | High-throughput, low cost | Limited to simple phenotypes | Preliminary target discovery |
Single-Cell CRISPR | Resolves heterogeneity and networks | High noise, expensive | Mechanism studies, precision medicine |
Spatial Multi-Omics + CRISPR | Preserves tissue context | Limited resolution/throughput | Tumor microenvironment, development |
8. Summary and Outlook
CRISPR + Single-Cell Sequencing revolutionizes functional genomics by enabling causal inference (linking perturbations to phenotypes) and systems-level integration (multi-omics). As costs decline (projected to $0.01/cell by 2025) and delivery systems improve (e.g., non-viral vectors), this approach will accelerate clinical translation in:
- Personalized Medicine: Patient-derived CRISPR screens for tailored therapies.
- Synthetic Biology: Validating synthetic gene circuits at single-cell resolution.
- Human Cell Atlas: Mapping gene function, cell states, and tissue localization in 3D.
This technology is poised to become a standard toolkit in life sciences, unlocking new dimensions in understanding complex biological systems.
email: chuanchuan810@gmail.com