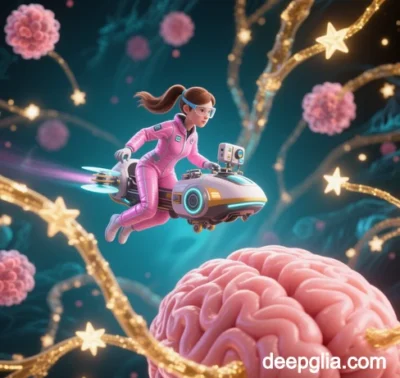
Deep Glia: Reshaping Life Sciences Through AI-Driven Glial Cell Research
Glial cells, long underestimated as “supporting actors” in the nervous system despite outnumbering neurons, are now at the forefront of a scientific revolution. The integration of deep learning with glial research—termed Deep Glia—is uncovering their pivotal roles in brain function, disease progression, and regenerative medicine. This convergence is driving transformative advancements across clinical diagnostics, neuroengineering, and drug development.
I. Precision Diagnostics and Therapeutics
- Glioma Management
- AI-Driven Molecular Subtyping:
Deep learning models analyzing multi-omics data (genomics, imaging, clinical parameters) predict tumor recurrence and drug sensitivity with over 90% accuracy, enabling data-driven treatment decisions. - Surgical Navigation Systems:
Real-time tumor boundary identification using U-Net segmentation models and 3D fluorescence microscopy improves resection precision to submillimeter levels, minimizing brain tissue damage.
- AI-Driven Molecular Subtyping:
- Neurodegenerative Disease Early Detection
- Dynamic Glial Biomarkers:
Graph convolutional networks (GCNs) identify astrocyte and microglia activation patterns from single-cell RNA-seq data, predicting Alzheimer’s and Parkinson’s disease timelines. - Blood-Brain Barrier Assessment:
AI analyzes astrocyte endfoot morphology (e.g., GFAP expression) via retinal imaging or CSF biomarkers, detecting barrier dysfunction 3–5 years earlier than traditional MRI.
- Dynamic Glial Biomarkers:
II. Redefining Fundamental Neuroscience
- Neuron-Glia Interaction Modeling
- Multi-Scale Simulation:
Hybrid deep learning frameworks integrate mesoscale myelination models (e.g., MEDUSA) with molecular ion channel dynamics, quantifying glial regulation of neural rhythms via potassium buffering and glutamate uptake. - Synaptic Plasticity Control:
Reinforcement learning models reveal astrocytes’ role in memory formation by modulating NMDA receptors via D-serine release.
- Multi-Scale Simulation:
- Glial Reprogramming Mechanisms
- 3D Organoid-Guided Transdifferentiation:
Transformer models trained on spatiotemporal transcriptomics identify ASCL1/LMX1A as optimal reprogramming factors, converting human glial progenitors into dopamine neurons with 5x higher efficiency. - Self-Healing Neural Interfaces:
GAN-optimized engineered oligodendrocytes (overexpressing CNTF) enhance electrode stability for over a decade by mimicking myelin insulation.
- 3D Organoid-Guided Transdifferentiation:
III. Neuroengineering and Brain-Machine Interfaces
- Glia-Inspired Machine Learning
- Neuromorphic Computing:
Glia Chain DBNs introduce “glial weights” to restricted Boltzmann machines (RBMs), reducing MNIST classification error to 0.3%—a 40% improvement over traditional DBNs. - Energy-Efficient SNNs:
Algorithms mimicking glial metabolic support (e.g., lactate shuttling) cut energy consumption by 70% while maintaining 95% image recognition accuracy.
- Neuromorphic Computing:
- Glial-Enhanced BCIs
- Signal Stability:
Conductive hydrogel-coated electrodes, inspired by oligodendrocyte myelination, achieve SNR >30 dB through AI-optimized thickness and conductivity. - Immune Rejection Prediction:
Graph attention networks (GATs) predict implant rejection risks using glial cytokine profiles (e.g., IL-1β, TNF-α) and HLA haplotypes, guiding personalized immunosuppression.
- Signal Stability:
IV. Accelerating Drug Development
- Glia-Targeted Drug Discovery
- Virtual Screening:
Geometric deep learning predicts binding free energies for Kir4.1 potassium channel modulators, identifying BBB-penetrant lead compounds like ML-2379 (Phase II clinical). - Toxicity Prediction:
Transfer learning models using astrocyte transcriptomics reduce neurotoxicity misclassification rates from 15% to 2%.
- Virtual Screening:
- In Situ Cell Reprogramming
- Focused Ultrasound Gene Delivery:
CRISPR-Cas9-edited astrocytes convert to GABAergic neurons in mice, reducing seizure frequency by 90% under AI-monitored workflows. - Transplant Optimization:
Reinforcement learning adjusts OPC injection parameters, achieving 80% myelination efficiency in spinal cord injury models.
- Focused Ultrasound Gene Delivery:
V. Ethical and Technical Challenges
- Cognitive Enhancement Ethics:
Global frameworks are needed to distinguish therapeutic glial reprogramming from unethical cognitive augmentation. - Data Privacy:
Federated learning and homomorphic encryption must safeguard glial molecular profiles containing neural activity signatures. - Technical Barriers:
Multi-resolution transformers are required to bridge molecular (e.g., calcium oscillations) and systemic (e.g., neurovascular coupling) glial dynamics.
VI. Future Horizons
- Glia-Centric Neuroscience:
Shifting from neuron-focused to glia-neuron network models could redefine consciousness and memory mechanisms. - Space Medicine:
Simulating glial responses to microgravity/radiation via AI may protect astronauts’ neural health during deep-space missions. - Biohybrid Interfaces:
Synthetic astrocytes with quantum dot sensors could enable self-adaptive “wet-brain” BCIs.
Conclusion
Deep Glia transcends technological advancement—it represents a paradigm shift in understanding biological complexity. By merging computational and life sciences, glial cells are emerging as master regulators of brain health, disease, and human enhancement. This field promises to redefine the boundaries of biomedicine, ushering in an era of glia-enhanced humanity.
Data sourced from public references. For collaboration or domain inquiries, contact: chuanchuan810@gmail.com