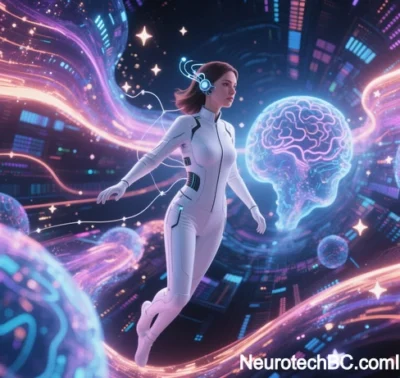
Applications and Recent Breakthroughs of Neurotech BCI in Medical Rehabilitation
Brain-Computer Interface (BCI) technology, a convergence of neuroscience and engineering, is revolutionizing the boundaries of medical rehabilitation. By decoding neural signals to enable human-machine interaction, BCI offers precise and dynamic interventions for patients with neurological impairments. Below is an in-depth exploration of its core applications and cutting-edge advancements:
I. Motor Function Rehabilitation: Restoring Neural-Muscular Control
- Active Recovery for Stroke and Spinal Cord Injury (SCI)
- Closed-Loop Neural Remodeling: BCI translates motor imagery (MI) or movement intention signals (e.g., EEG, ECoG) into commands for exoskeletons, functional electrical stimulation (FES), or robotic arms. For example, a Swiss Federal Institute of Technology system uses spinal stimulation to bypass injuries, enabling SCI patients to stand and walk.
- Multimodal Feedback Enhancement: Combining VR and tactile feedback, BCI training activates co-remodeling of motor and sensory cortices. Brazil’s Walk Again Project improved motor coordination in SCI patients through EEG-controlled exoskeletons with plantar feedback.
- Parkinson’s Disease and Movement Disorder Management
- Closed-Loop Deep Brain Stimulation (DBS): Real-time monitoring of abnormal basal ganglia oscillations (e.g., beta waves) allows dynamic adjustment of stimulation parameters. Zhejiang University’s closed-loop stimulator reduced motor slowness in Parkinson’s patients.
- Tremor Suppression: BCI systems predict tremors milliseconds before onset and trigger inhibitory stimulation, significantly reducing tremor amplitude.
II. Language and Communication Restoration: Decoding Silent Speech
- Brain Language Mapping
- Speech Synthesis: Stanford’s ECoG-based system decodes vocal tract movements to synthesize natural speech, while Chinese teams achieved real-time Chinese speech decoding for aphasia patients.
- Thought-to-Text Conversion: Non-invasive BCI using P300 or SSVEP paradigms maps attention to virtual keyboards, enabling ALS patients to communicate at accelerated rates.
- Emotional Expression Enhancement
- Limbic Signal Decoding: fNIRS signals from the anterior cingulate and insula identify depressive or anxious states. Neurofeedback training modulates amygdala activity, alleviating symptoms in major depressive disorder.
III. Neuromodulation and Pain Management: Proactive Interventions
- Chronic Pain Mitigation
- Pain Signal Localization: BCI identifies neuropathic pain types (e.g., burning, stabbing) via θ-γ oscillations in the prefrontal and anterior insular cortices, triggering targeted spinal stimulation or drug release.
- VR-BCI Therapy: Immersive tasks in virtual environments combined with neurofeedback reduce chronic back pain intensity.
- Epilepsy Prediction and Control
- Seizure Precursor Detection: LSTM-based BCI predicts seizures minutes in advance, activating responsive vagus nerve stimulation (VNS) to reduce frequency.
IV. Cognitive and Emotional Rehabilitation: Rewiring Neural Networks
- Attention and Memory Training
- Closed-Loop Cognitive Enhancement: BCI adapts task difficulty based on prefrontal θ and parietal α wave synchrony, extending attention spans in ADHD patients.
- Alzheimer’s Intervention: Theta-pulse transcranial magnetic stimulation (TMS) targeting the default mode network (DMN) improves cognitive scores in early-stage patients.
- PTSD Therapy
- Fear Memory Erasure: BCI feedback training strengthens amygdala-prefrontal connectivity, paired with exposure therapy to reduce PTSD symptoms.
V. Technological Innovations: Overcoming Signal Challenges
- Hardware Advancements
- Flexible Electronics: KAIST’s nanomesh electrodes conform to brain folds, achieving single-neuron resolution with superior signal-to-noise ratios.
- Optogenetic BCI: Caltech’s miniaturized fiber probes use channelrhodopsin (ChR2) for precise neural circuit modulation, restoring visual cortex function in mice.
- Algorithmic Breakthroughs
- Deep Learning Decoding: Transformer-based EEGNet-V2 achieves high accuracy in motor imagery classification.
- Cross-Subject Adaptation: Meta-BCI frameworks personalize systems with minimal calibration data, boosting command recognition in ALS patients.
- System Integration
- Wireless Closed-Loop BCI: MIT’s “neurograins” enable continuous cortical monitoring and adaptive stimulation via body-area networks.
VI. Challenges and Ethical Considerations
- Technical Limitations
- Signal Stability: Long-term electrode implants face signal degradation due to inflammation.
- Latency Issues: Current system delays hinder fine motor control applications.
- Ethical Risks
- Neural Privacy: Brain signals risk exposing subconscious intentions, necessitating “neurodata firewalls.”
- Equity in Enhancement: BCI-driven cognitive boosts may widen educational disparities, demanding global ethical guidelines.
VII. Future Trends: From Rehabilitation to Human Augmentation
- Multimodal Integration
- Brain-Spine-Peripheral Interfaces: DARPA’s projects integrate BCI, spinal interfaces, and bioelectronics to restore full mobility in paralysis.
- Home-Based Rehabilitation
- Wearable BCI: Lightweight EEG headsets (e.g., Huawei-West China Hospital collaboration) lower costs for at-home stroke recovery.
- Brain-Inspired AI Fusion
- Digital Twin Brain Models: Meta’s brain atlas simulates whole-brain dynamics to optimize BCI strategies.
Conclusion
Neurotech BCI has transitioned from labs to clinics, offering not just functional recovery but also biological healing through neural plasticity. As flexible electronics, optogenetics, and quantum computing converge, BCI will evolve into miniaturized, intelligent, and universal systems, redefining human potential. However, rapid technological growth requires robust ethical frameworks to ensure equitable benefits and prevent societal divides.
Data sourced from public references. For collaboration or domain inquiries, contact: chuanchuan810@gmail.com