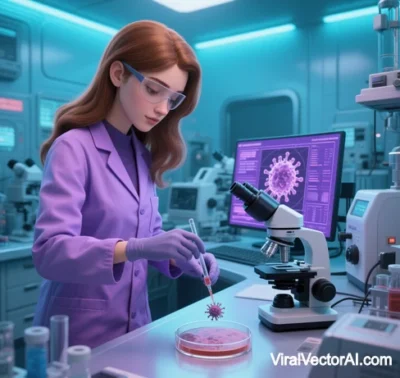
ViralVectorAI: The Role and Future of AI-Driven Viral Vector Technology in Gene Editing
The integration of AI and viral vector technology is revolutionizing gene editing, driving advancements in precision, intelligence, and industrial scalability. By optimizing vector design, predicting editing outcomes, and streamlining production, this synergy holds transformative potential across therapeutics, agriculture, and biomanufacturing. Below is an analysis of its core value, applications, challenges, and future directions.
I. Core Technological Synergies
- Intelligent Vector Design
- Capsid Engineering: AI analyzes vast datasets of capsid protein sequences and tissue penetration metrics to design highly specific variants. For example, platforms like FORMsightAI predict AAV capsid-receptor interactions, enhancing liver/brain targeting precision.
- Adaptive Vectors: AI-driven evolutionary algorithms create “environment-responsive” vectors (e.g., light- or metabolite-activated AAVs) for context-dependent gene editing in specific microenvironments like hypoxic tumors.
- Enhanced Editing Efficiency and Safety
- Off-Target Prediction: Models like DeepMind’s AlphaMissense identify CRISPR-Cas9 off-target sites, reducing unintended edits when combined with viral delivery strategies.
- Multiplex Gene Regulation: AI-optimized gene circuits coordinate the timed expression of editing tools (e.g., base and prime editors) for complex disease therapies.
- Cost-Effective Scalable Production
- Process Simulation: Machine learning optimizes critical parameters (e.g., transfection efficiency, purification yields), cutting AAV production timelines and costs.
- Microfluidics Automation: AI-powered microfluidic systems enable real-time monitoring and optimization, ensuring batch consistency.
II. Key Applications and Milestones
Field | Application | Breakthrough |
---|---|---|
Genetic Disease Therapy | AAV5 delivery of LETI-101 nuclease for Huntington’s disease, silencing mutant HTT alleles | AI-optimized nuclease-gRNA pairing preserves healthy alleles |
Cancer Immunotherapy | Oncolytic adenovirus with CRISPR-edited PD-1 inhibitors boosts tumor remission | AI-designed dual-targeting capsids improve tumor penetration |
Agricultural Improvement | Lentiviral delivery of OsHKT1 editors enhances salt-tolerant rice yields | AI identifies stress-resistance SNPs and optimizes promoters |
Biomanufacturing | Engineered bacteria-AAV systems produce terpenoid biofuels | AI dynamically balances metabolic flux for industrial-scale output |
III. Challenges and Solutions
- Delivery Efficiency Limitations
- Challenge: Poor viral penetration in complex tissues (e.g., blood-brain barrier, solid tumors).
- Solutions:
- Multi-Omics Integration: AI combines single-cell transcriptomics and protein interaction data to model 3D penetration pathways.
- Stealth Capsids: Generative adversarial networks (GANs) design immune-evading capsids inspired by viral evolution (e.g., HIV variants).
- Manufacturing Consistency and Regulation
- Challenge: High empty capsid rates and genomic integration risks.
- Solutions:
- AI Quality Control: Raman spectroscopy and deep learning ensure viral particle integrity.
- Self-Destruct Vectors: Temperature-sensitive degradation tags (tsDeg) enable localized vector clearance.
- Ethical and Safety Concerns
- Challenge: Ecological risks from transmissible gene-editing vectors.
- Solutions:
- Antisense RNA Circuits: AI-designed circuits restrict vector replication in non-target species.
- Global Ethics Framework: Blockchain-based GET Matrix tracks vector lifecycle for reversibility and control.
IV. Future Trends: From Tools to Ecosystems
- AI-Synthetic Biology Fusion
- Host-Vector Coevolution: AI models simulate long-term immune interactions to design adaptive vectors.
- Minimalist Genomes: CRISPR-Cas12 and AI co-design compact AAV genomes (<3 kb) for multi-editor cargo.
- Accelerated Clinical Translation
- Virtual Trials: AI digital twins predict patient-specific responses, reducing animal testing.
- Personalized Vector Libraries: HLA-matched AAV capsid platforms aim for broad patient coverage.
- Cross-Disciplinary Innovation
- Quantum Computing: IBM quantum systems optimize CRISPR-vector interactions for atomic-level precision.
- Brain-Machine Interfaces: Light-activated AAVs paired with neural decoding AI enable closed-loop therapies for Parkinson’s disease.
Conclusion
AI-driven viral vector technology is redefining the gene editing pipeline—design, delivery, production, and regulation:
- Short-Term (2025–2027): Clinical breakthroughs in genetic diseases and cancer therapies.
- Mid-Term (2028–2030): Scalable agricultural and biomanufacturing applications with reduced costs.
- Long-Term (2030+): A unified AI-vector-editing platform for programmable genomes across species.
China’s leadership in AAV capsid engineering (e.g., novel liver-targeting vectors) and ethical governance frameworks (GET Matrix) positions it as a global hub for intelligent viral vector innovation.
Data sourced from public references. For collaboration or domain inquiries, contact: chuanchuan810@gmail.com