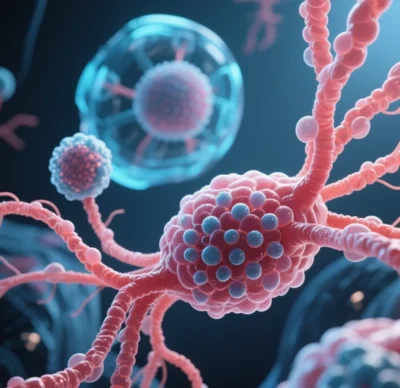
CRISPRSingle and Systems-Level Multi-Omics Integration: Building a Closed-Loop System for Functional Genomics
1. Technical Framework and Core Logic
CRISPRSingle refers to a technology integrating CRISPR gene editing at the single-cell level with multi-omics analysis. Its core objective is to establish a closed-loop system of “edit-observe-model” through precise genetic perturbations (editing) and high-dimensional data capture (multi-omics). This integration addresses the bottleneck of cellular heterogeneity in traditional bulk analyses, enabling a shift from “correlation-based hypotheses” to “mechanism-driven validation.” The framework operates across three layers:
- Single-cell CRISPR Perturbation: Targeted gene knockout, point mutations, or epigenetic modifications using CRISPR-Cas9, base editing, or epigenetic editing tools.
- Multi-modal Omics Capture: Integrates single-cell transcriptomics (scRNA-seq), proteomics (CITE-seq), epigenomics (scATAC-seq), and spatial omics (e.g., DBiT-seq) to resolve multidimensional molecular phenotypes post-editing.
- Systems-Level Causal Inference: Employs machine learning models (e.g., causal network inference, Bayesian hierarchical models) to map dynamic relationships between genetic perturbations and phenotypic changes.
2. Key Technological Breakthroughs
2.1 High-Throughput Single-Cell CRISPR Screening
- Perturb-seq/CROP-seq: Links sgRNA to single-cell barcodes via lentiviral vectors, enabling parallel perturbation and phenotyping of thousands of genes at single-cell resolution. For example, MIT researchers mapped functional landscapes of immune-related genes in human T cells.
- CRISPR-DBiT: Combines spatial transcriptomics to analyze microenvironmental effects of gene editing (e.g., heterogeneity in PD-1 inhibitor responses within tumor microenvironments).
2.2 Synchronized Multi-Omics Capture
- CITE-seq/REAP-seq: Simultaneously profiles transcriptomes and surface proteomes using antibody-DNA conjugates, revealing cascade effects of gene editing on signaling pathways (e.g., NF-κB, MAPK).
- SNuBar-seq: Integrates CRISPR screening with metabolomics to track metabolite dynamics post-perturbation (e.g., 2-HG accumulation driven by IDH1 mutations in leukemia models).
2.3 Closed-Loop Feedback Systems
- CRISPR-Loop: Trains generative adversarial networks (GANs) on single-cell multi-omics data to predict optimal editing strategies and iteratively refine sgRNA libraries.
3. Applications and Case Studies
3.1 Decoding Complex Disease Mechanisms
- Cancer Immunotherapy: CRISPRSingle-mediated knockout of checkpoint genes (e.g., PD-1, CTLA-4) in T cells, combined with single-cell TCR sequencing and cytokine profiling, identifies synergistic gene combinations (e.g., CD28 co-stimulation with IFN-γ signaling) to enhance antitumor activity.
- Neurodegenerative Diseases: Edits Alzheimer’s risk genes (e.g., APOE, TREM2) in iPSC-derived neurons and uses spatial metabolomics to pinpoint metabolic switches driving amyloid plaque formation.
3.2 Precision Drug Development
- Target Discovery: Identifies EGFR inhibitor resistance genes in organoid models, uncovering non-coding lncRNA EMERALD as a phase separation-driven bypass signaling activator.
- Off-Target Assessment: Integrates single-cell whole-genome sequencing (scWGS) and chromatin accessibility analysis to map unintended structural variants (e.g., LINE-1 retrotransposon activation).
3.3 Synthetic Biology and Cell Engineering
- CAR-T Smart Engineering: Incorporates AND-logic gate regulators (e.g., synNotch receptors) into CAR-T precursors, validated via multi-omics for dual tumor-antigen recognition.
- Microbial Metabolic Engineering: Edits 12 mevalonate pathway genes in yeast and optimizes artemisinin precursor production using single-cell metabolic flux analysis.
4. Advantages and Challenges
Advantages
Dimension | Breakthrough Value |
---|---|
Resolution | Single-cell precision reveals heterogeneity in editing outcomes (e.g., sgRNA efficiency across cell cycle phases). |
Causality | Perturbation-response paired data constructs gene regulatory networks (GRNs), transcending correlation-based studies. |
Scalability | Supports end-to-end applications from basic research (TF screening) to clinical translation (personalized cell therapies). |
Challenges and Solutions
Challenge | Innovative Solutions |
---|---|
Data Integration Complexity | Develop multi-omics alignment algorithms (e.g., MOFA+) and unified coordinate systems (UMAP3D). |
Editing Efficiency Limits | Use compact Cas12f nucleases and microfluidic electroporation to enhance single-cell delivery. |
Computational Demands | Deploy cloud-accelerated causal inference platforms (e.g., Google DeepVariant for CRISPR). |
5. Future Trends and Ethical Considerations
5.1 Technological Convergence
- Single-Cell Epigenetic Editing: Combine dCas9-DNMT3A with scATAC-seq for spatiotemporal DNA methylation reprogramming.
- In Vivo Dynamic Monitoring: Develop CRISPR-activated reporter systems (e.g., CRISPR-SLIDE) to track edited cells in real time.
5.2 Ethical and Regulatory Frameworks
- Data Sovereignty: Establish decentralized blockchain platforms (e.g., CellHash) to protect patient privacy and ensure traceability.
- Biosafety: Embed suicide switches (e.g., CRISPR-Kill) in synthetic biology applications to prevent engineered microbe leakage.
6. Conclusion
CRISPRSingle and systems-level multi-omics integration mark a paradigm shift in functional genomics from descriptive science to engineered solutions. By enabling causal dissection at single-cell resolution and closed-loop feedback, this approach accelerates discovery of biological principles and unlocks transformative potential in cancer immunotherapy and rare disease treatment. However, challenges in data integration, tool miniaturization, and ethical governance remain. By 2030, quantum computing and AI advancements may propel the field from “single-cell editing” to “tissue-level reprogramming.”
Data sourced from public references. For collaboration or domain inquiries, contact: chuanchuan810@gmail.com