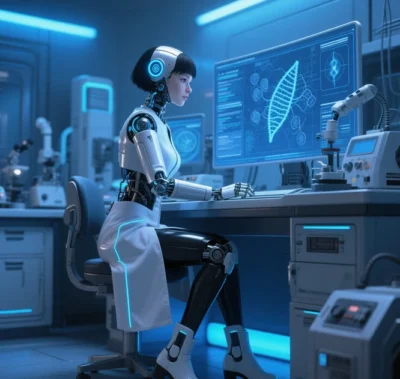
AI-Driven Viral Vector Technology (ViralVectorAI): A Revolutionary Breakthrough in Gene Therapy
1. Definition and Core Technologies
ViralVectorAI refers to the integration of artificial intelligence (AI) to optimize the design, production, and delivery efficiency of viral vectors (e.g., adeno-associated virus (AAV), lentivirus), enhancing the precision and safety of gene therapies. Key technologies include:
- AI-Driven Capsid Engineering: Uses deep learning to predict viral capsid structures and functions, enabling the design of targeted, low-immunogenicity vectors.
- Multimodal Data Integration: Combines genomics, proteomics, and high-throughput experimental data to build predictive models for vector performance (e.g., transduction efficiency, tissue specificity).
- Dynamic Optimization Platforms: AI algorithms adjust viral production processes in real time to reduce costs and improve yield.
2. Applications and Case Studies
2.1 Targeted Delivery Optimization
- Tissue-Specific Vector Design:
- Harvard researchers used deep learning to analyze AAV capsid sequences, identifying variants that evade immune detection and target the liver, retina, and central nervous system.
- MIT-Broad Institute models predict capsid surface charge distribution to enhance cardiac and muscle tissue enrichment.
- Barrier Penetration:
- AI-designed lipid nanoparticles (LNPs) fused with viral capsids enable blood-brain barrier penetration for neurodegenerative disease treatment.
2.2 Immunogenicity Control
- Immune Evasion Strategies:
- AI models predict pre-existing antibody binding epitopes in human serum, enabling the design of “stealth capsids” (e.g., VP3 mutants) for use in antibody-positive patients.
- Immunomodulatory peptides (e.g., CD47 mimics) are embedded into vectors to suppress innate immune responses.
2.3 Gene Editing Synergy
- CRISPR-Cas9 Delivery:
- AI optimizes AAV cargo capacity via split-vector systems (dual-AAV) to deliver large gene editors (e.g., Base Editors), achieving sustained Factor IX expression in hemophilia models.
- Non-integrating lentiviral variants are screened by AI to reduce insertion risks.
3. Technological Advantages
Dimension | Traditional Methods | AI-Driven Technology |
---|---|---|
Design Cycle | Months to years of random mutagenesis screening. | AI predicts candidate sequences, accelerating efficiency. |
Targeting Precision | Limited to natural serotypes. | Custom designs (e.g., brain-targeted AAV-PHP.eB) enhance organ specificity. |
Safety | High doses risk hepatotoxicity or immune storms. | AI models predict minimal effective doses, reducing clinical requirements. |
Production Cost | Complex purification, exceeding $1M per dose. | AI-optimized bioreactors increase yield. |
4. Challenges and Innovative Solutions
4.1 Data Limitations
- Challenge: Scarce high-quality data on vector-host interactions (e.g., single-cell delivery trajectories).
- Solutions:
- Transfer learning integrates cross-species data (mice, primates) to enhance model generalization.
- Generative adversarial networks (GANs) simulate virtual experiments.
4.2 Computational Complexity
- Challenge: Capsid design involves trillions of possible sequences.
- Solutions:
- Quantum annealing (e.g., D-Wave systems) optimizes search efficiency.
- Distributed cloud computing (e.g., AWS Batch) trains trillion-parameter models.
4.3 Ethical and Regulatory Risks
- Challenge: Unpredictable biological risks (e.g., neo-antigenicity).
- Solutions:
- Embedded biosensors (e.g., fluorescent reporters) track vector behavior in real time.
- Blockchain records AI design workflows for traceability.
5. Future Trends
- Automated Vector Foundries: AI-controlled microfluidic chips integrate design, production, and quality control.
- Human-Machine Coevolution: Combines AI with directed evolution to generate millions of variants weekly.
- Cross-Species Universal Vectors: AI models develop “universal” vectors for humans, pets, and endangered species.
6. Summary
ViralVectorAI is transforming gene therapy from trial-and-error R&D into predictive engineering, enabling viral vectors with higher precision, lower toxicity, and enhanced programmability. With tools like AlphaFold 3, AI-designed vectors could address 90% of genetic disease targets within five years, reducing treatment costs to below $100,000 per dose.
Data sourced from public references. For collaboration or domain inquiries, contact: chuanchuan810@gmail.com