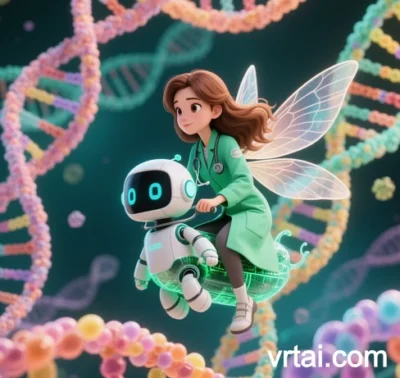
VRT AI: Applications in Medical Rehabilitation and Virtual Reality Therapy
VRT AI—the integration of virtual reality therapy (VRT) and artificial intelligence (AI)—is advancing medical rehabilitation through intelligent, personalized, and precise interventions. Below is a systematic analysis of its technical framework, clinical applications, breakthroughs, and ethical challenges:
I. Technical Framework: Synergy Between VRT and AI
VRT AI operates on a closed-loop “perception-decision-feedback” system:
- Multimodal Sensing Layer
- Biosignal Acquisition: VR headsets, wearable sensors (EEG/EMG/ECG), and motion capture devices collect real-time physiological data (balance, brainwaves, heart rate) and movement trajectories.
- Environmental Interaction: AI analyzes behavioral patterns (gaze focus, navigation paths) to assess treatment adherence and cognitive states.
- AI Decision Engine
- Dynamic Difficulty Adjustment: Reinforcement learning optimizes task difficulty (e.g., platform tilt in balance training) based on real-time performance.
- Personalized Protocols: NLP processes medical histories to generate tailored VRT plans (e.g., visuo-vestibular integration for vestibular dysfunction).
- Immersive Feedback Layer
- Multisensory Stimulation: AI-driven virtual environments (e.g., simulated supermarkets or cliffs) enhance neuroplasticity through visual, auditory, and tactile feedback.
- Real-Time Biofeedback: EMG or EEG signals control virtual avatars, creating a “motor intent-to-virtual action” loop for motor recovery.
II. Clinical Applications
- Vestibular and Balance Rehabilitation
- Compensatory Mechanism Activation: Simulated dynamic visual conflicts (e.g., moving light bars) train vestibulo-ocular reflex (VOR) adaptation, reducing dizziness.
- Fall Risk Prediction: AI models analyze gait data (stride, swing speed) and balance metrics to adjust virtual obstacle courses.
- Neurological Rehabilitation
- Post-Stroke Motor Recovery: VR mirror therapy with AI motion recognition synchronizes virtual and affected limbs, enhancing corticospinal tract remodeling.
- Parkinson’s Freezing-of-Gait Alerts: Deep learning detects gait phase anomalies, triggering auditory/visual cues to reduce freezing episodes.
- Mental Health Interventions
- PTSD Exposure Therapy: AI generates controlled trauma scenarios (e.g., battlefields) with adjustable environmental details for systematic desensitization.
- Anxiety Regulation: HRV feedback adapts virtual meditation environments to improve autonomic nervous system regulation.
- Chronic Pain Management
- Multimodal Analgesia: AI-composed music paired with virtual snow/forest scenes distracts attention and reduces pain perception.
- Neuroplasticity: fMRI shows VRT AI suppresses pain-related anterior cingulate cortex activity while boosting endogenous opioid release.
III. Cutting-Edge Innovations
- Brain-Computer Interface (BCI) Integration
- Intent-Driven Training: CNN-decoded motor imagery EEG signals control virtual prosthetics to accelerate motor cortex reorganization.
- Closed-Loop Neuromodulation: Real-time subthalamic nucleus (STN) analysis optimizes deep brain stimulation (DBS) parameters for Parkinson’s treatment.
- Digital Twins and Prognostics
- Personalized Avatars: Biomechanical models based on joint mobility and muscle strength simulate rehabilitation outcomes.
- Long-Term Efficacy Modeling: GNNs predict relapse risks by analyzing longitudinal balance and cognitive data.
- Remote Rehabilitation Ecosystems
- 5G-VR Platforms: Edge computing enables low-latency remote training supervised by AI, improving rural patient adherence.
- Blockchain Data Security: Smart contracts protect privacy while enabling federated learning for cross-institutional model training.
IV. Challenges and Ethical Considerations
- Technical Barriers
- Signal Interference: VR headset electromagnetic noise affects EEG quality, necessitating noise-filtering algorithms.
- Model Interpretability: “Black-box” AI decisions require tools like SHAP values for medical compliance.
- Clinical Translation Hurdles
- Cost and Accessibility: High-end VR devices remain unaffordable for low-income populations.
- Standardization Gaps: Lack of interoperability between VRT AI systems demands ISO/IEEE data interface standards.
- Ethical Risks
- Reality Confusion: Over-immersion risks in children and psychiatric patients require session duration limits.
- Algorithmic Bias: Training data skewed toward specific ethnic groups reduces accuracy for diverse populations.
V. Future Directions (2025–2030)
- Quantum Computing: Quantum neural networks (QNNs) could decode millions of neurons in real time for complex motor rehabilitation.
- Metaverse Healthcare: Web3.0-based virtual rehab communities may host AI-guided group therapies (e.g., Parkinson’s balance challenges).
- Synthetic Biology Integration: CRISPR-enhanced neuroplasticity paired with VRT AI could treat spinal cord injuries (preclinical trials ongoing).
Conclusion
VRT AI is redefining medical rehabilitation through immersive environments and intelligent decision-making, overcoming the spatiotemporal limits of traditional therapies. While challenges in technical maturity, ethics, and healthcare integration persist, breakthroughs in neural interfaces, quantum computing, and the metaverse could establish VRT AI as universal infrastructure for chronic disease management and functional recovery within five years.
Data sourced from public references. For collaboration or domain inquiries, contact: chuanchuan810@gmail.com