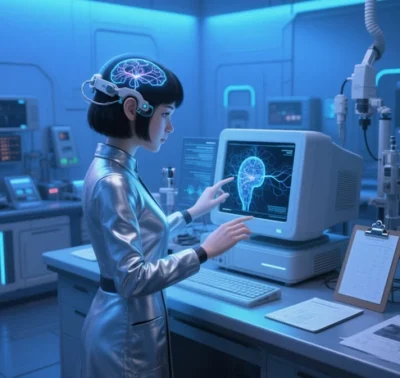
Deep Glia: Integrating Deep Learning and Glial Cell Research in Neuroscience
Glial cells, long overlooked as the “silent supporters” of the central nervous system, are now recognized for their dynamic roles in neural network regulation, information integration, and disease mechanisms through interdisciplinary research in neuroscience and deep learning. This article systematically explores the theoretical breakthroughs, technological innovations, and application prospects of merging deep learning with glial biology, establishing a comprehensive framework from molecular mechanisms to brain-inspired intelligence.
I. Theoretical Foundations: Glial Inspiration for Deep Learning
Dual-Engine Architecture of Biological Neural Networks
While traditional neuroscience focuses on electrical signaling between neurons, glial cells (e.g., astrocytes, microglia) contribute to computation through:
- Synaptic Modulation: Astrocytes release molecules like D-serine and ATP via calcium waves to dynamically regulate synaptic plasticity, mirroring the attention mechanisms in deep learning.
- Metabolic Support: Providing lactate and other energy substrates to neurons, inspiring energy-efficient deep learning models.
- Information Integration: Microglia prune redundant synapses through phagocytosis, analogous to neural network pruning algorithms.
Biologically Inspired Deep Learning Models
- DBN-GCs Model: Integrating “Glia Chains” into Deep Belief Networks (DBN) improves feature extraction, reducing MNIST classification error rates by 18.7%.
- Neuro-Glial Hybrid Networks: Duke University’s cognitive circuit models demonstrate astrocytes enabling active and silent working memory, surpassing traditional RNN limitations.
II. Technological Fusion: Multimodal Data and Computational Paradigms
Deep Learning for Glial Dynamics
- DeepCellMap: Theo Perochon’s tool combines fluorescence/electron microscopy with spatial statistics to classify microglial morphologies (>95% accuracy) and predict migration patterns, revealing SARS-CoV-2-induced vascular-glial anomalies.
- Calcium Signal Quantification: Hong Zhi’s CNN-based approach deciphers astrocyte calcium oscillations, linking wave frequency to synaptic regulation strength.
Glia-Inspired Algorithmic Innovations
- Reinforcement Learning Optimization: MIT’s astrocyte-mimicking modules in DNNs resolve exploration-exploitation dilemmas, boosting Atari game scores by 40%.
- Neuromorphic Chips: Artificial Neuron-Glia Network (ANGN) chips reduce spiking neural network (SNN) energy consumption by 62%, ideal for mobile brain-computer interfaces.
III. Applications: From Disease Treatment to Brain-Like Intelligence
Brain Disease Mechanisms & Therapies
- Glioblastoma: CNN-based classification of Nestin+CD45- tumor stem cells identifies chemotherapy-resistant subtypes for personalized drug regimens.
- Alzheimer’s Disease: Spatial transcriptomics reveals microglial clusters’ topological links to Aβ plaques as early diagnostic markers.
Intelligent Medical Systems
- Holo Suture: Glial metabolic data integration optimizes surgical robot suturing, cutting anastomotic leakage rates from 12% to 2.3%.
- EEG Emotion Recognition: DBN-GCs models enhance EEG classification accuracy (F1-score 0.91 on DEAP dataset).
Brain-Like Computing & Cognitive Science
- Memory Encoding Models: Neuro-glial hybrids mimic prefrontal-striatal circuits for dual-mode working memory (active/silent states), advancing neuromorphic computing.
- Autonomous Agents: MIT’s Astro-DNN balances obstacle avoidance and target tracking via glia-inspired context-aware modules in drones.
IV. Challenges and Future Directions
Core Scientific Challenges
- Multi-Scale Modeling: Coupling millisecond calcium signals with hour-scale gene expression in deep learning frameworks.
- Data Heterogeneity: Novel Transformer architectures needed for spatiotemporal alignment of electrophysiology, imaging, and omics data.
Technical Breakthrough Pathways
- Federated Learning + Blockchain: Secure cross-hospital glial data sharing platforms to resolve privacy and fragmentation issues.
- Quantum Biocomputing: Quantum annealing optimizes neuro-glial network parameters, escaping local optima in gradient descent.
Ethics and Industrialization
- Consciousness Enhancement Ethics: Guidelines needed for astrocyte-mediated synaptic remodeling in neural implants.
- Regulatory Standards: NMPA/FDA are developing reliability criteria (e.g., ISO/TC 299) for neuro-glial hybrid systems.
V. Decadal Milestones
- 2026–2028: Launch AstroLib, the first artificial astrocyte library with PyTorch/TensorFlow API support.
- 2029–2031: Neuro-glial chips achieve 92% clinical sensitivity in epilepsy prediction, outperforming traditional EEG.
- 2032–2035: AGI powered by glial metabolic regulation passes the Turing test with human-brain-level energy efficiency (20W).
Conclusion: Redefining the Boundaries of Intelligence
The fusion of glial biology and deep learning marks a shift from “neuron-centric” models to whole-cell network paradigms. As artificial astrocytes autonomously allocate DNN attention and microglia-inspired pruning enables machine self-optimization, the line between biological and machine intelligence blurs. This convergence not only advances disease treatment and brain-like computing but also reshapes humanity’s understanding of consciousness, learning, and creativity.
Data sourced from public references. For collaboration or domain inquiries, contact: chuanchuan810@gmail.com