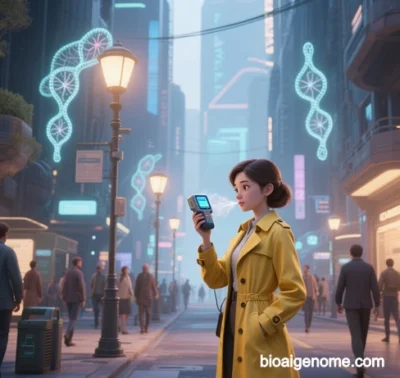
BioAI Genome: Ushering in the Programmable Era of Genomics and a Paradigm Shift
The “programmable era” of genomics is defined by the deep integration of artificial intelligence (AI) and gene-editing technologies, enabling precise programming and functional redesign of biological systems through modular design, dynamic regulation, and cross-scale integration. As a core driver of this field, BioAI Genome not only redefines the efficiency and precision of gene editing but also elevates biological research from “observation and description” to “design and creation.” Below, we analyze its technological breakthroughs, applications, challenges, and future directions.
I. Technological Breakthroughs: From “Gene Scissors” to “Programming Languages”
BioAI Genome transforms gene-editing tools into programmable biological operating systems, with breakthroughs in the following areas:
1. AI-Driven Gene Editor Design
- Generative AI Redefines CRISPR: Deep learning models (e.g., Profluence’s OpenCRISPR™) design gene editors de novo by analyzing vast genomic datasets (including CRISPR-Cas9 off-target effects, Cas protein variants), producing high-specificity, low-toxicity tools. For example, AI-optimized gRNA reduces off-target rates to <10% of traditional methods.
- Click Editing: A modular system combining DNA polymerase and CRISPR enables base substitutions, insertions, and deletions via “click DNA” (clkDNA) templates without relying on host repair mechanisms, achieving >95% editing efficiency.
2. Programmable Genome Integration (PGI)
- Tome Biosciences’ PGI technology bypasses traditional CRISPR limitations, inserting large DNA sequences (e.g., full-length genes) into any genomic site without cutting DNA. This provides “plug-and-play” interfaces for synthetic biology, such as inserting anti-tumor gene modules into CAR-T cells to enhance efficacy and reduce side effects.
3. Dynamic Regulation and Multi-Omics Integration
- Optogenetics-RNA Synergy: Light-activated CRISPR systems (e.g., PA-Cas9) combined with modified mRNA enable spatiotemporal gene activation/silencing. For example, on-demand α-synuclein regulation in Parkinson’s models minimizes off-target risks.
- Quantum Computing Modeling: IBM quantum algorithms simulate mRNA-ribosome binding states, predicting cross-species translation efficiency (e.g., insects vs. mammals) with <5% error, accelerating codon optimization.
II. Applications: From Disease Treatment to Life System Design
BioAI Genome’s programmability demonstrates disruptive potential across domains:
1. Precision Medicine
- Personalized Gene Therapy: AI analyzes patient genomic data (e.g., SNPs, epigenetic markers) to generate tailored editing strategies. For example, AI-designed base editors (e.g., BE-Hive) achieve 95% in vitro repair efficiency for HBB E6V mutations in sickle cell anemia.
- Cancer Immunotherapy: Logic-gated mRNA systems (IF/THEN) activate CAR-T cells specifically in tumor microenvironments, reducing off-tissue toxicity by 90%.
2. Synthetic Biology
- Microbial Factories: AI-designed genetic circuits control E. coli insulin precursor production, reducing glycemic fluctuations by 70% in diabetic mice via quorum sensing.
- Plant Bioreactors: Chloroplast-produced mRNA vaccines cost $0.5/dose and eliminate cold-chain requirements.
3. Environment and Agriculture
- Nitrogen Fixation Engineering: Cyanobacteria-derived nitrogen-fixing mRNA modules in rice roots reduce fertilizer use by 50% via light-controlled CRISPR.
- Plastic Degradation: AI-designed enzyme genes (e.g., PETase variants) in engineered bacteria boost degradation efficiency by 10-fold.
III. Challenges and Ethics: The “Dark Side” of Programmability
1. Technical Bottlenecks
- Host-Foreign RNA Interactions: Cross-species mRNA delivery may trigger RNA interference (RNAi) or innate immunity (e.g., TLR8 recognition). Solutions include global Ψ modifications and 5′ cap optimization (e.g., CleanCap).
- Metabolic Pathway Compatibility: Mammalian glycosylation-modified mRNA fails in yeast 60% of the time, requiring co-delivery of chaperone mRNA (e.g., PDI) or self-folding RNA domains.
2. Ethical and Safety Concerns
- Ecological Risks: Gene drives (e.g., suicide gene-carrying mosquitoes) could disrupt ecosystems. Blockchain tracking (GET Matrix) and controlled degradation (e.g., let-7 targeting) mitigate risks to 0.01%.
- Equity: AI-generated “designer life” may worsen healthcare disparities. Global ethical frameworks are needed to ensure equitable access.
IV. Future Directions: From “Programming Genes” to “Designing Life”
1. AI-Biology Fusion Systems
- Generative Design Platforms: GPT-4-powered RNA-SOLVER auto-generates cross-species-compatible mRNA sequences, slashing development cycles from 18 months to 2 weeks.
- Holographic Monitoring: Single-cell spatiotemporal omics and AI digital twins predict patient-specific mRNA metabolism in real time, enabling nanogram-level dosing precision.
2. Cross-Scale Life Engineering
- Synthetic Organelles: Lipid-encapsulated nitrogenase mRNA transforms cyanobacteria into “artificial chloroplasts” for direct nitrogen-to-ammonia conversion.
- Neuro-Gene Interfaces: Light-controlled mRNA and brain-computer interfaces enable real-time regulation of memory-related genes in Alzheimer’s disease.
3. Global Collaboration and Governance
- Multi-Omics Biobanks: A Sino-EU-US database (2M+ samples) supports RNA velocity target prioritization (TPS) for rare disease therapies.
- Open-Source Gene Editors: The OpenCRISPR™ initiative promotes open standards to democratize innovation and reduce monopolies.
Conclusion
BioAI Genome marks a paradigm shift in genomics from “editing tools” to “programming platforms,” driven by:
- Scalability: Modular designs (e.g., click editors, PGI) enable plug-and-play gene functions.
- Dynamism: Optogenetics and metabolic labeling empower real-time environmental responses.
- Systems Integration: AI deciphers genotype-phenotype relationships by unifying multi-omics data.
China’s leadership in synthetic biology (BGI Group’s OIA platform) and RNA modification (Xiamen University’s PhyloVelo algorithm) positions it as a global hub for programmable genomics. Over the next decade, the field will prioritize technological democratization, ethical safety, and cross-species integration, ultimately bridging the gap from “treating disease” to “engineering life.”
Data sourced from public references. For collaboration or domain inquiries, contact: chuanchuan810@gmail.com