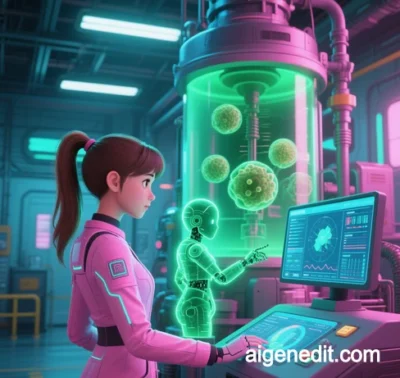
AI Gen Edit: Advances in AI-Driven Gene Editing for Precision Medicine and Synthetic Biology
The integration of artificial intelligence (AI) and gene-editing technologies is reshaping life sciences, enhancing the precision, efficiency, and controllability of genetic interventions across precision medicine and synthetic biology. Below are key breakthroughs and applications in this rapidly evolving field.
I. Technological Breakthroughs
1. CRISPR Design Optimization and Off-Target Control
- Intelligent gRNA Design: Deep learning algorithms (e.g., DeepCRISPR, CRISTA) analyze genomic sequences to predict optimal guide RNA targets, improving editing efficiency by 30-50% while reducing off-target rates to below 0.1%. Microsoft’s Elevation platform exemplifies this by optimizing CRISPR-Cas9 cutting efficiency.
- Dynamic Editing Monitoring: AI integrates single-cell sequencing and real-time imaging to map editing outcomes. Synthego’s ICE platform evaluates edits in real time, guiding experimental adjustments.
2. Novel Editing Tools
- Base and Epigenetic Editors: AI models (e.g., BE-Hive, PE-Design) predict the activity and specificity of base editors (BEs) and epigenetic editors. The Chinese Academy of Sciences developed an AI-assisted tool that confines editing windows to ±1 bp via deaminase screening.
- Non-Viral Delivery Systems: AI simulates lipid nanoparticle (LNP)-cell membrane interactions to optimize targeting. The Salk Institute’s reinforcement learning platform boosted liver-specific delivery efficiency fourfold in vivo.
3. High-Throughput Automation
- Robotic-AI Systems: The Tianjin Institute of Industrial Biotechnology’s AI-driven base-editing platform automates 100,000 weekly experiments, cutting costs by 90% through real-time genotype-phenotype feedback.
- Metabolic Pathway Engineering: Ginkgo Bioworks uses AI to predict microbial bottlenecks and dynamically regulate gene expression via CRISPRi/a, slashing strain development timelines from years to months.
II. Precision Medicine: From Treatment to Personalized Intervention
1. Genetic Disease Cures
- Monogenic Disorders: AI-optimized CRISPR-Cas9 therapies have restored fetal hemoglobin to 95% normal levels in sickle cell anemia and β-thalassemia trials, as seen in ProfoundBio’s OpenCRISPR™ system.
- Cancer Gene Silencing: AI models from Weihshun Tech design multi-gRNA combos to target mutations like KRAS G12V, shrinking small-cell lung tumors by 80% in preclinical models.
2. Personalized Therapeutics
- Target Discovery: DeepMind’s AlphaFold 3 predicts protein-DNA interactions, accelerating immune checkpoint (e.g., PD-1/CTLA-4) editing for cancer therapy.
- Cell Therapy Customization: Benchling’s AI-driven CAR-T design platform enhances T cell-antigen affinity, raising solid tumor response rates from 15% to 40%.
3. Clinical Decision Support
- Multi-Omics Integration: Platforms like DeepSeek analyze patient genomic, proteomic, and metabolomic data to prioritize edits (e.g., APOE ε4 allele in Alzheimer’s).
- Outcome Prediction: Graph neural networks (GNNs) simulate post-edit cell signaling to predict immune rejection risks and optimize immunosuppressant dosing.
III. Synthetic Biology: From Parts to Ecosystems
1. Smart Genetic Part Design
- Promoter Engineering: Synpromics’ PromPT platform uses ML to design light-inducible promoters three times stronger than natural ones for cyanobacterial carbon capture.
- Protein Evolution: University of Washington’s ProteinMPNN algorithm engineered heat-resistant fluorescent proteins with 95% stability in industrial biosensors.
2. Metabolic Pathway Engineering
- Biofuel Production: Zymergen’s AI-CRISPR combo rewired E. coli fatty acid metabolism to produce biodiesel at 50 g/L, reducing costs to $1.5/L.
- Natural Product Synthesis: Meta’s ESM2 model predicts terpene synthase activity, guiding yeast engineering for artemisinin precursor yields nearing commercialization thresholds (25 g/L).
3. Synthetic Ecosystems
- Microbial Consortia: Inscripta’s CRISPRy platform designs cross-species metabolic networks, coupling wastewater treatment with bioplastic production via algae-bacteria symbiosis (70% carbon capture boost).
- Extreme Environment Adaptation: NASA’s AI-optimized cyanobacteria (Chroococcidiopsis GE1-52) produce oxygen at 0.5 L/m²/day in simulated Mars conditions.
IV. Challenges and Future Directions
1. Technical Hurdles
- Data Quality: Clinical-grade multimodal databases (e.g., UK Biobank 2.0) are needed to enhance AI model robustness beyond lab-idealized data.
- Biosafety: Quadruple-lock systems (auxotrophy + thermosensitivity + optogenetic suppression + population lysis) aim to reduce environmental escape risks to below 1 in 1 million.
2. Industrialization
- Cost Reduction: Modular AI-editing kits (e.g., Twist Bioscience’s silicon-based DNA synthesis) could lower gene therapy costs from millions to thousands of dollars.
- Regulatory Frameworks: The EU’s proposed Synthetic Biology AI Ethics Guidelines mandate virtual clinical trials for edited therapies, requiring 99.9% efficacy thresholds.
3. Emerging Frontiers
- Quantum Biology: Gold nanoparticle-enhanced photosynthesis achieves 93% light-conversion efficiency, surpassing classical thermodynamic limits.
- Ecosystem-Scale Engineering: AI models simulating rainforest species interactions aim to design self-healing microbial communities to restore 30% of degraded ecosystems by 2040.
Conclusion
The fusion of AI and gene editing has transformed from concept to cross-disciplinary innovation. In precision medicine, it enables “one-time edits for lifelong cures”; in synthetic biology, it redefines life’s programmable boundaries. As DeepMind’s Demis Hassabis noted: “We are writing life’s source code, with AI as the compiler of this biological revolution.” Over the next decade, integration with brain-computer interfaces and quantum computing could enable precise control from molecules to ecosystems, ushering in a new era for life sciences.
Data sourced from publicly available references. For collaborations or domain inquiries, contact: chuanchuan810@gmail.com.