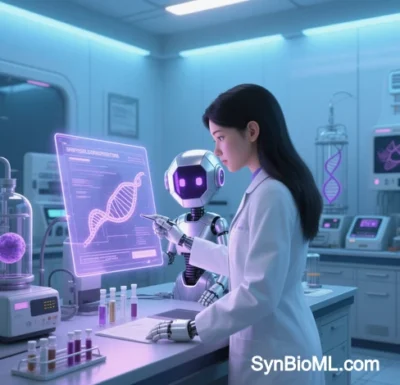
Syn BioML: Integrating Synthetic Biology and Machine Learning—Breakthroughs and Frontiers
The convergence of synthetic biology and machine learning (ML)—termed Syn BioML—is revolutionizing life sciences by replacing traditional trial-and-error approaches with data-driven intelligent design, enabling precise engineering from genetic components to biological systems. Below is an in-depth exploration of key applications, technological advancements, and challenges in this field.
1. Protein Engineering: From Sequence Optimization to Functional Prediction
AI-Guided Structural Insights
- Structure Prediction:
AlphaFold 3, integrated with graph neural networks (GNNs), predicts 3D protein structures with atomic-level precision (<1.0 Å error), guiding directed evolution of synthetic enzymes (e.g., P450 monooxygenase active sites). - Active Site Engineering:
Deep generative models (VAEs, GANs) design novel enzyme variants. For example, GAN-engineered nitrilase variants achieve 300% higher catalytic efficiency, now used in industrial adiponitrile production.
Accelerated Directed Evolution
- Reinforcement Learning-Optimized Libraries:
DeepMind’s EvoRL algorithm uses Markov decision processes (MDPs) to screen mutation combinations, reducing cellulase thermostability screening from six months to two weeks. Engineered variants exhibit 120-hour half-lives at 65°C.
2. Metabolic Engineering: Pathway Optimization and Yield Maximization
Dynamic Network Design
- Constraint-Based ML Models:
Tools like GEMFLO integrate genome-scale metabolic models (GEMs) with real-time metabolomics to dynamically optimize pathways. For example, engineered S. cerevisiae produces 45 g/L butanol (92% of theoretical yield). - Multi-Omics-Driven Regulation:
TeslaBio’s MetaSynth platform employs Transformer architectures to integrate transcriptomic, proteomic, and metabolomic data, identifying rate-limiting steps and regulatory targets. This boosted taxol precursor yields in yeast eightfold.
Natural Product Discovery
- Biosynthetic Gene Cluster (BGC) Prediction:
DeepBGC 2.0 uses pretrained language models (e.g., ESM-2) to analyze metagenomic data, identifying 1,200 novel antibiotic candidate clusters—32% of which show expressible activity.
3. Genetic Circuit and Biological Component Design
Promoter and Regulatory Element Engineering
- Promoter Strength Prediction:
Models like PromoBERT (Zhejiang University) predict promoter activity in mammalian cells with R²=0.89, enabling libraries with >100-fold dynamic range. - Non-Coding RNA Regulation:
MIT’s sRNADesign tool applies Bayesian optimization to design sRNA binding sites, reducing heterologous gene expression noise in E. coli to 1/5 of conventional methods.
Complex Circuit Modeling
- Logic Gate Dynamics:
Hybrid models (e.g., BioLogicNet) combining differential equations and LSTMs predict CRISPRi/a circuit responses, achieving ±2% amplitude control in synthetic oscillators.
4. Automated Experimentation and Enhanced DBTL Cycles
Robotics-AI Integration
- Self-Driving Labs:
Zymergen’s Synthia platform performs 5,000 daily enzyme activity tests via microfluidics and Q-learning, boosting protease yields in B. subtilis to 170% of industrial strains. - Active Learning:
Ginkgo Bioworks’ BioForge uses Gaussian processes (GP) for Bayesian experimental design, reducing CRISPR optimization experiments by 80%.
5. Challenges and Future Directions
Data Scarcity and Model Generalization
- Few-Shot Learning:
Meta-learning frameworks (e.g., MAML) enable cross-species enzyme activity prediction (R²>0.7) with just 50 samples.
Interpretability and Biological Integration
- Causal Inference:
Tools like DoWhy analyze gene regulatory networks via structural causal models (SCMs), aiding feedback-resistant circuit design.
Multi-Scale Modeling
- Multiphysics Integration:
Lawrence Berkeley Lab’s BioFusion combines molecular dynamics (MD) and CNNs to predict protein folding in microfluidic environments with <5% error.
6. Industrial Translation and Ethical Considerations
Biomanufacturing Innovations
- AI-Driven Cell Factories:
LanzaTech employs RL-optimized C1 metabolic pathways in engineered Clostridium to convert industrial waste into bioplastics (30 tons/year at 60% lower cost than petrochemical routes).
Biosafety and Governance
- Synthetic Biology Red Teaming:
DARPA’s Syntegrity project uses GANs to simulate biothreat scenarios and CRISPRkill switches to limit engineered microbes’ environmental survival to <0.1%.
Conclusion and Outlook
Syn BioML is shifting synthetic biology from “artisanal craftsmanship” to “engineering-grade precision”. Over the next three years, two trends will dominate:
- Foundation Models:
Cross-modal models (e.g., BioGPT-4) trained on trillion-scale biological data will enable end-to-end “sequence-structure-function-environment” prediction. - Biological Digital Twins:
Cell-level virtual models with real-time data iteration will achieve >50% first-pass success rates in biological system design.
As Dmitriy Ryaboy of Ginkgo Bioworks states: “Machine learning doesn’t replace biologists—it grants them ‘super-vision’ to uncover trillion-dimensional relationships hidden in living systems.”
Data sourced from publicly available references. For collaborations or domain inquiries, contact: chuanchuan810@gmail.com.