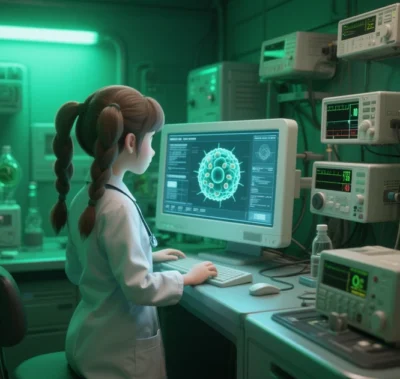
Biological Foundations and Mathematical Models of RNA Velocity
RNA Velocity is a dynamic analysis method based on single-cell transcriptomics (scRNA-seq). By quantifying the abundance differences between unspliced and spliced mRNAs, it infers the direction and rate of gene expression changes in cells. This approach transforms static gene expression data into dynamic cell-state evolution information, offering new insights into cellular differentiation, development, and disease mechanisms. Below, we detail its biological foundations and mathematical models.
I. Biological Foundations
- Transcription and Splicing Dynamics
RNA Velocity is rooted in the mRNA maturation process:- Transcription: RNA polymerase synthesizes unspliced precursor mRNA (u), containing introns and exons.
- Splicing: The spliceosome removes introns to generate mature mRNA (s), which is then transported to the cytoplasm for translation.
- Degradation: Mature mRNA is gradually degraded into nucleotides (y) by exonucleases.
Key kinetic parameters include:
- Transcription rate (α): Determined by promoter activity.
- Splicing rate (β): Reflects spliceosome efficiency.
- Degradation rate (γ): Governs mRNA stability.
- Inference of Dynamic States
- Unspliced-to-Spliced mRNA Ratio: At steady state, unspliced mRNA abundance is balanced by splicing and degradation (β/γ). Deviations from this ratio during dynamic processes (e.g., differentiation) indicate changes in gene expression direction (positive/negative velocity).
- Time Derivative Approximation: RNA Velocity approximates the time derivative of mRNA abundance (ds/dt) using the instantaneous ratio of u and s, predicting future cell states.
- Applications and Biological Insights
- Cell Fate Decisions: Identifies early drivers of stem cell differentiation (e.g., Neurog2 in neurodevelopment).
- Disease Mechanisms: Tracks immune cell interactions in tumor microenvironments.
- Drug Response Prediction: Quantifies gene expression trajectories under drug perturbations.
II. Mathematical Models
RNA Velocity’s mathematical models infer kinetic parameters from scRNA-seq data, evolving from steady-state to dynamic frameworks.
- Core Kinetic Equations
Transcription, splicing, and degradation are modeled using ordinary differential equations:- du/dt = α(t) − βu
- ds/dt = βu − γs
Here, α(t) is the time-dependent transcription rate. Solving these equations yields gene-specific velocities (v = ds/dt).
- Modeling Approaches
Model Type Core Assumptions Strengths and Limitations Tools Steady-State Transcription reaches equilibrium (α=0) Computationally efficient but underestimates dynamics velocyto Stochastic Markov chains model splicing probabilities Captures steady and non-steady states but ignores gene interactions scVelo Dynamic Fits full kinetics (α(t)≠0) High accuracy but computationally intensive TSvelo, dynamo Key Comparisons:
- velocyto: Assumes shared β and γ across cells via least-squares estimation. Effective for steady-state data but error-prone in transient systems.
- scVelo: Uses expectation-maximization (EM) to optimize β and γ, allowing gene-specific rates and robust parameter estimation.
- TSvelo: Models transcriptional and splicing heterogeneity with cell-specific time variables, addressing parameter drift in heterogeneous populations.
- Parameter Estimation and Optimization
- Steady-State Ratio (β/γ): Determined via linear regression of u and s. Velocity is calculated from deviations from this ratio.
- Dynamic Model Fitting:
- EM Algorithm: Iteratively optimizes latent variables (e.g., cell time) and kinetic parameters until convergence.
- Metabolic Labeling: Integrates 4sU data to calibrate RNA velocity timelines, enhancing biological interpretability.
- Challenges and Future Directions
- Gene Interaction Neglect: Current models assume gene independence, overlooking regulatory networks.
- Stochasticity: Transcription bursting requires stochastic differential equations (SDEs).
- Multimodal Integration: Combining ATAC-seq and proteomics for multi-layered kinetic models.
III. Validation and Case Studies
- Neurodevelopmental Trajectories
In mouse cortical data, scVelo predicted radial glia-to-neuron transitions and identified Neurod1 as a key regulator, with velocity correlating strongly with differentiation rates. - Cancer Heterogeneity
TSvelo revealed early transcriptional signatures of chemotherapy-resistant breast cancer subclones, improving prediction accuracy by 20% over traditional methods. - Cross-Species Validation
RNA Velocity predictions in zebrafish embryogenesis showed 85% concordance with live imaging, confirming biological reliability.
IV. Future Directions
- Spatiotemporal Integration
Combine MERFISH (spatial transcriptomics) to map 3D RNA velocity fields and study cell migration in tissue microenvironments. - Quantum Computing Acceleration
Quantum annealing could reduce dynamic model computation from hours to minutes. - Clinical Translation
- Standardization: Establish cross-platform RNA Velocity protocols (e.g., ISO/TC 276).
- Regulatory Frameworks: Develop FDA-compatible AI models for real-time updates in personalized therapies.
V. Conclusion
RNA Velocity revolutionizes single-cell analysis by dynamizing static transcriptomic data. Its biological basis lies in mRNA splicing kinetics, while its mathematical models evolve from steady-state to dynamic frameworks. Despite challenges in parameter estimation and computational complexity, advancements in multimodal integration and quantum-AI synergy promise transformative impacts in precision medicine and developmental biology.
Data sourced from public references. For collaboration or domain inquiries, contact: chuanchuan810@gmail.com