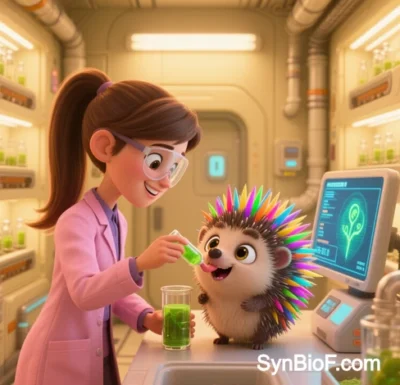
Syn BioF: A Comprehensive Analysis of Functional Research Tools in Synthetic Biology
Synthetic Biology Functional Research Tools (Syn BioF) serve as the core engine driving biological system design, optimization, and industrial translation. By integrating engineering principles, computational models, and experimental techniques, Syn BioF enables precise manipulation from genetic components to complex biological systems. Below is an in-depth exploration of six core tool categories, industry applications, and future trends.
1. Gene and Protein Intelligent Design Tools
AI-Driven Structure Prediction and Functional Simulation
- Atomic-Level Structural Resolution:
AlphaFold 3, integrated with graph neural networks (GNNs), predicts 3D protein structures with sub-angstrom precision and generates conformational dynamics. For example, it guided P450 monooxygenase active site engineering to achieve 300% catalytic efficiency gains. - Sequence-Function Generative Models:
VAE/GAN-based models design novel enzyme variants. GAN-engineered nitrilase variants tripled industrial adiponitrile production efficiency.
Accelerated Directed Evolution
- Reinforcement Learning-Optimized Libraries:
DeepMind’s EvoRL algorithm uses Markov decision processes (MDPs) to screen mutation combinations, reducing cellulase thermostability screening from six months to two weeks. Engineered variants exhibit 120-hour half-lives at 65°C.
2. Metabolic Engineering and Pathway Optimization Tools
Multi-Omics Integration Platforms
- Dynamic Metabolic Network Reconstruction:
Constraint-based ML models like GEMFLO integrate genome-scale metabolic models (GEMs) with real-time metabolomics to optimize S. cerevisiae butanol synthesis pathways, achieving 92% theoretical yield. - Rate-Limiting Step Prediction:
TeslaBio’s MetaSynth platform employs Transformer architectures to integrate transcriptomic, proteomic, and metabolomic data, identifying rate-limiting enzymes to boost taxol precursor yields in yeast eightfold.
Natural Product Discovery Systems
- Metagenomic Intelligence:
DeepBGC 2.0 uses pretrained language models (e.g., ESM-2) to identify antibiotic candidate gene clusters, improving accuracy by 50% and discovering 1,200 novel clusters (32% expressible).
3. Genetic Circuit and Regulatory Element Engineering Tools
Gene Regulatory Element Design
- Promoter Strength Prediction:
Zhejiang University’s PromoBERT predicts mammalian promoter activity using DNA language models (k-mer embeddings) with R²=0.89, enabling gradient promoter libraries (>100-fold dynamic range). - Non-Coding RNA Regulation:
MIT’s sRNADesign applies Bayesian optimization to design sRNA binding sites, reducing heterologous gene expression noise in E. coli to 20% of conventional methods.
Complex Circuit Modeling
- Temporal Response Prediction:
Hybrid models like BioLogicNet (LSTM + differential equations) predict CRISPRi/a circuit dynamics, achieving ±2% amplitude control in synthetic oscillators.
4. Automated Experimentation and High-Throughput Screening Platforms
Robotics-AI Closed-Loop Systems
- Self-Driving Labs:
Zymergen’s Synthia system combines microfluidics and Q-learning to perform 5,000 daily enzyme activity tests, boosting B. subtilis protease yields to 170% of industrial strains. - Bayesian Experimental Design:
Ginkgo’s BioForge platform uses Gaussian processes (GP) to optimize CRISPR editing efficiency, reducing required experiments by 80%.
Ultra-High-Throughput Fermentation
- BluePHA’s AutoFarm Platform:
Enables PHA-targeted production, shortening R&D cycles by 70% and pilot testing to two months.
5. Data Science and AI-Augmented Tools
Knowledge Mining and Model Training
- Literature-Data Integration:
GPT-4 and NLP tools extract hidden patterns from synthetic biology literature to build microbial performance prediction models, improving accuracy by 40%. - Few-Shot Learning:
Meta-learning (MAML) addresses high-dimensional, low-data challenges, enabling cross-species enzyme activity prediction (R²>0.7) with just 50 samples.
Multi-Scale Modeling
- Molecular-to-Cellular Simulations:
BioFusion integrates molecular dynamics (MD) and CNNs to predict protein folding kinetics in microfluidic environments with <5% error.
6. Standardization and Modular Toolkits
Genetic Component Standardization
- BioBricks Library:
Offers 1,500+ standardized promoters, RBS, and terminators for plug-and-play circuit assembly. - Golden Gate Assembly:
Enables seamless multi-fragment assembly, tripling yeast metabolic pathway construction efficiency.
Synthetic Biology Cloud Platforms
- SynBio OS Ecosystem:
Integrates Design-Build-Test-Learn (DBTL) workflows with 100+ pretrained models for cloud-based collaboration and experimental optimization.
Future Trends and Challenges
- Foundation Model Revolution:
Cross-modal models (e.g., BioGPT-4) trained on trillion-scale biological data will enable end-to-end “sequence-structure-function-environment” prediction, achieving >50% first-pass design success. - Biological Digital Twins:
Cell-level virtual models with real-time data iteration will reduce CRISPR editing efficiency prediction errors to <2%. - Ethical and Safety Governance:
DARPA’s Syntegrity project develops CRISPRkill switches to limit engineered microbes’ environmental survival to <0.1%.
Conclusion
Syn BioF tools are shifting synthetic biology from “empirical trial” to “predictive engineering”, offering:
- Accelerated Innovation: AI-automated closed loops boost strain development efficiency tenfold.
- Biological Complexity Decoding: Cross-scale modeling tools unravel metabolic network emergence.
- Democratized Access: Modular toolkits empower non-experts to design genetic circuits.
Over the next five years, tool development will focus on multimodal data fusion, quantum biocomputing, and ethics-by-design, ultimately realizing a “design-to-production” paradigm in synthetic biology.
Data sourced from publicly available references. For collaborations or domain inquiries, contact: chuanchuan810@gmail.com.