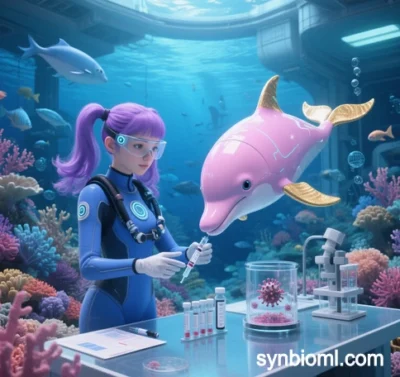
Syn BioML: Convergence of Synthetic Biology and Machine Learning
Algorithm-Driven Innovations in Drug Development, Disease Diagnosis, and Biomanufacturing
Technical Framework and Core Principles
Syn BioML (Synthetic Biology Machine Learning) integrates synthetic biology with machine learning to decode biological data and reprogram living systems. Its framework comprises:
- Data Layer: Aggregates multi-omics data (genomics, proteomics, metabolomics) into scalable biological databases.
- Algorithm Layer: Employs deep learning (e.g., GANs), reinforcement learning, and quantum computing to predict biological component functions, optimize metabolic pathways, and design genetic circuits.
- Application Layer: Implements closed-loop iteration (Design-Build-Test-Learn) in drug discovery, diagnostics, and industrial strain optimization.
Drug Development: From Target Discovery to Molecular Design
1. Target Identification and Virtual Screening
- Metabolic Pathway Prediction: ML models identify rate-limiting steps in pathways (e.g., artemisinin synthesis optimization).
- Target-Drug Interaction: AlphaFold-predicted protein structures combined with molecular docking algorithms (e.g., AutoDock) enhance compound screening success rates.
2. Synthetic Biology-Driven Production
- Cell Factory Optimization:
- Reinforcement learning optimizes microbial metabolism for higher product titers (e.g., 18-fold yield increase in taxol precursor production).
- CRISPRi-based circuits dynamically adjust gene expression to avoid metabolic overload.
- Case Studies:
- Antibiotics: GANs generate novel antibiotic scaffolds, shortening R&D cycles to 6 months.
- CAR-T Therapy: ML-designed non-viral delivery systems achieve 95% editing efficiency.
Disease Diagnosis: Smart Biosensors and Real-Time Monitoring
1. Molecular Diagnostics
- Nucleic Acid Sensors: RNN-optimized CRISPR-Cas gRNA enables single-copy/μL detection sensitivity (e.g., COVID-19 variant identification).
- Synthetic Gene Circuits: ML-designed logic-gated biosensors distinguish cancer cells with >99% specificity.
2. Real-Time Health Monitoring
- Wearable Biochips: Engineered microbes with ML modules monitor gut metabolites (e.g., IL-6) and transmit data via Bluetooth.
3. Clinical Applications
- Early Cancer Screening: ctDNA sequencing + ML models predict tumor risk (AUC 0.93).
- Antibiotic Resistance Prediction: SVM models trained on bacterial transcriptomes achieve 89% accuracy.
Biomanufacturing: Process Optimization and Sustainability
1. Intelligent Strain Engineering
- High-Throughput Screening: Robotic platforms test 10⁵ mutants daily (e.g., β-carotene production in yeast).
- Directed Evolution: Algorithms mimic natural selection to enhance enzyme thermostability.
2. Production Control
- Parameter Optimization: Gaussian process regression (GPR) models predict optimal fermentation conditions, boosting yields by 30%.
- Digital Twins: Real-time bioreactor simulations reduce batch failure rates and costs.
3. Circular Economy
- Waste Upcycling: ML-designed microbial consortia convert plastic waste into PHA bioplastics.
- Carbon Capture: Engineered bacteria with quantum computing-optimized pathways fix CO₂ 50x faster than algae.
Ethics and Future Directions
1. Risk Mitigation
- Biosafety: CRISPR molecular tags track synthetic biology components.
- Algorithmic Bias: Federated learning frameworks reduce demographic biases in diagnostics.
2. Emerging Frontiers
- Quantum Biocomputing: IBM quantum processors simulate protein folding 100x faster.
- Neuro-Synthetic Biology: ML-designed optogenetic tools modulate neuronal activity for Parkinson’s treatment.
3. Industry Evolution
- Platform Services: Ginkgo Bioworks’ “Biofoundry” offers modular strain design.
- Cost Reduction: Cloud labs slash single-experiment costs to under $100.
Conclusion
Syn BioML is transforming life sciences:
- Drug Development: Shifts from trial-and-error to predictive models, cutting R&D cycles by 60%.
- Diagnostics: Portable biosensors reduce point-of-care costs to $1/test.
- Biomanufacturing: Halves carbon footprints while growing bio-based markets to $500B.
Over the next decade, programmable living systems—from personalized cancer vaccines to carbon-negative factories—will redefine technological boundaries. Balancing innovation with ethics remains critical to achieving the vision of engineering life for human benefit.
Data sourced from publicly available references. For collaborations or domain inquiries, contact: chuanchuan810@gmail.com.