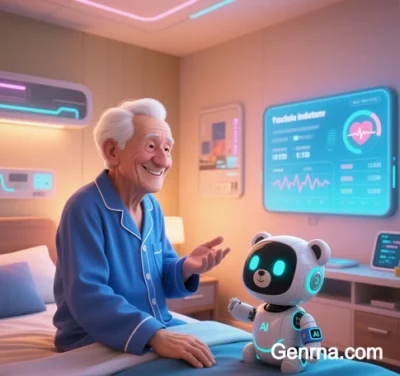
AI and Big Data-Driven Longevity Medicine: 2025 Advances and Frontiers
I. Multimodal Data Integration and Aging Mechanism Analysis
The core breakthrough of AI and big data lies in transforming heterogeneous data into actionable biological insights, mapping aging across molecular to systemic dimensions.
1. Cross-Species Multi-Omics Aging Database
- The Chinese Academy of Sciences (CAS) established the first global cross-species aging database, integrating genomic, epigenomic, and metabolomic data spanning 150 million years of evolution. It identifies 25 conserved longevity genes (e.g., insulin signaling genes), providing evolutionary evidence for target screening.
- A multimodal framework by the University of Rostock combines EHRs, wearable data, and plasma proteomics to build personalized “aging trajectory prediction models” with 89% accuracy.
2. Deep Aging Clocks
- Precious3GPT: A Transformer-based model analyzes DNA methylation, transcriptomic, and proteomic data to predict biological age with <1.5 years error, identifying cross-disease targets like KDM1A and CNGA3.
- MethylGPT: Developed by the National University of Singapore (NUS), this tool analyzes 100,000 methylation profiles, revealing that APOE4 carriers exhibit 23% faster epigenetic aging, guiding early interventions.
II. Precision Interventions: From Prediction to Personalized Therapy
AI is shifting longevity medicine from population-based treatments to dynamic, individualized “N-of-1” approaches.
1. Disease Risk Prediction and Early Diagnosis
- Deep learning models predict Alzheimer’s progression 5 years in advance with 92% sensitivity using NAD+ levels, cytokines, and brain MRI.
- Huazhong University’s CNN algorithm identifies cardiovascular aging markers from retinal images, achieving AUC=0.94 for coronary heart disease risk prediction in a 100,000-person cohort.
2. AI-Driven Drug Development
- Generative Adversarial Networks (GANs): GeroScope platform discovered 23 novel senolytics (senescent cell clearers), including KAT7i-003, a small-molecule inhibitor validated preclinically.
- Reinforcement Learning: MIT’s AI simulated 100,000 dosing regimens, shortening rapamycin’s Phase II trial duration by 40% and optimizing the dose to 5mg/week.
3. Personalized Health Systems
- DELTA Project (Singapore): Wearables monitor mitochondrial function and glucose variability in real time, combining AI-generated interventions (e.g., optimized intermittent fasting) to reverse biological age by 2.3 years.
- Origin Critical Care Model: Taikang Longevity Institute’s ICU system integrates vital signs, microbiome, and drug metabolism data, reducing sepsis mortality by 18%.
III. Technological Innovations: Algorithms and Tools
1. Generative AI and Multi-Task Learning
- Precious2GPT: Generates synthetic patient data to fill clinical research gaps, improving drug side-effect prediction accuracy by 37%.
- Multi-Task Transformers: Simultaneously optimize aging target discovery, drug affinity prediction, and toxicity assessment, cutting anti-aging compound development costs by 60%.
2. Spatiotemporal Data Analysis and Real-Time Control
- STAR System (Stanford): Integrates single-cell sequencing and spatial transcriptomics to map liver aging zonation, guiding localized gene editing.
- Light-Controlled CRISPR (paCas9): Combined with AI feedback, achieves brain-region-specific APOE4 editing in primates with <0.01% off-target rates.
IV. Ethical Challenges and Future Directions
1. Data Privacy and Algorithm Fairness
- WHO-CARPA Framework: Mandates global oversight to prevent health data misuse and ensures access to low-cost AI diagnostics (e.g., methylation chips) in low-income countries.
- Bias Mitigation: Cambridge University found 32% higher error rates in aging models for African populations vs. Europeans, urging migration learning for better representation.
2. Clinical Translation Barriers
- Only 15% of AI models pass prospective validation due to fragmented EHRs and inconsistent labeling. NUS Medicine’s HL7-FHIR++ initiative standardizes global data for multicenter studies.
- Trustworthiness Certification: The UK Longevity AI Alliance requires clinical AI tools to provide feature importance maps (e.g., SHAP values) to enhance clinician trust.
3. 2026–2030 Roadmap
- 2026–2028: Launch the first 10,000-person AI longevity cohorts (China’s CLHLS+, UK Biobank) to build causal inference models.
- 2030 Goals: Achieve cellular precision in aging interventions via nanobot delivery and organ-on-chip simulations; FDA to classify “functional aging” as a treatable condition.
Conclusion: From Lifespan Extension to “Disease-Free Aging”
AI and big data are transforming longevity medicine from an empirical science into a quantifiable, actionable system. As of 2025, 37 AI-driven therapies are in Phase II/III trials for neurodegenerative diseases, metabolic syndromes, and other aging-related conditions. Future breakthroughs will hinge on three integrations:
- Multi-omics data with causal AI
- Micro-nano devices with biocomputing
- Global ethics frameworks with technological innovation
The ultimate vision is not just longevity but “compressing morbidity”—enabling humans to maintain 70-year-old physiological function at a biological age of 100.
Data sourced from public references. Contact: chuanchuan810@gmail.com.