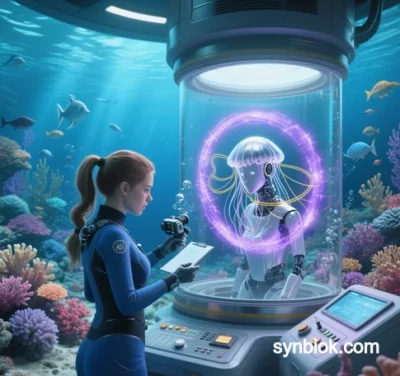
Core Challenges in Synthetic Biology Kinetics: A Deep Dive
I. Foundational Challenges in Model Construction
Parameterization Challenges & Data Noise
Kinetic models (e.g., ODEs, FBA) for synthetic biosystems rely heavily on experimental parameters such as enzymatic reaction rates (kcat) and transcription factor binding constants. However, experimental measurement errors (±20–50%) and single-cell heterogeneity (CV >30%) lead to significant prediction biases. For example:
- The KM values of rate-limiting enzymes can vary by 10-fold between in vitro and in vivo environments.
- Promoter strengths follow log-normal distributions at the single-cell level, rendering mean-based models inadequate.
Multiscale Integration Barriers
Synthetic biosystems involve coupled dynamics across molecular-cellular-population scales, but current frameworks (e.g., hybrid ODE-FBA models) face limitations:
- Time-Scale Separation Failure: Strong coupling between gene expression (minutes) and metabolic flux (seconds) in scenarios like optogenetic control requires delay differential equations, exponentially increasing computational complexity.
- Spatial Heterogeneity Neglect: Traditional models ignore diffusion gradients in biofilms or microreactors, causing >40% errors in industrial fermenter metabolic flux predictions.
AI Integration Limitations
While deep learning (e.g., Transformers, GNNs) aids parameter prediction, its effectiveness is constrained by:
- Uncertainty Quantification Gaps: Most AI models (e.g., DeepPrime) lack error propagation mechanisms, yielding unreliable confidence intervals for low-SNR data.
- Poor Biological Interpretability: Black-box models fail to reveal causal mechanisms, such as CRISPR-dCas9 activation thresholds linked to promoter conformations.
II. Intrinsic Complexities of Biological Systems
Nonlinear Dynamics & Chaotic Effects
Synthetic genetic circuits (e.g., oscillators, logic gates) often exhibit unintended behaviors due to nonlinear feedback (e.g., protein degradation vs. dilution rate competition):
- Bistability Boundary Ambiguity: Resource-limited conditions (e.g., ATP scarcity) can reduce theoretical toggle switches to monostable states.
- Chaotic Oscillations: Repressilator systems show 300% period variability depending on chromosomal integration sites.
Host-Circuit Resource Competition
Metabolic burden from engineered strains (e.g., ATP consumption by heterologous genes) disrupts kinetics, but models inadequately quantify this:
- Resource Balance Model (RBCM) Shortcomings: Focus on global resources (e.g., ribosomes) while ignoring local metabolite competition (e.g., NADPH).
- Orthogonality Deficits: Cross-binding of heterologous promoters to host RNA polymerase triggers “parasitic transcription,” consuming up to 15% of cellular resources.
Evolutionary Dynamics Uncertainty
Long-term stability of synthetic systems is influenced by mutation rates (μ≈10−9 bp−1 gen−1) and selection pressures:
- Functional Decay Thresholds: Plasmid loss probabilities for antibiotic resistance genes deviate from models by 20% after 100 generations.
- Mutation Hotspots: Persistent CRISPR-Cas9 expression induces host SOS responses, accelerating genomic rearrangements.
III. Engineering Barriers in Technology Translation
Scale-Up Prediction Mismatches
Lab-scale models (e.g., shake-flask cultures) fail under industrial bioreactor conditions due to hydrodynamic effects and metabolic heat accumulation:
- Oxygen Transfer Rate (OTR) Discrepancies: kLa variations between 5L and 5000L fermenters cause >50% errors in TCA cycle flux predictions.
- pH Gradient Nonlinearity: Local pH fluctuations (±0.5 units) in large-scale cultures alter enzyme activity, disrupting dynamic circuits.
Cross-Host Compatibility Issues
Identical circuits show divergent kinetics across hosts due to:
- Codon Usage Bias: Translation rate differences (up to 7x) between E. coli and yeast disrupt metabolic flux balance.
- Chaperone Interference: Bacillus subtilis GroEL/ES systems misfold eukaryotic proteins, altering enzyme kinetics.
Real-Time Monitoring Gaps
Closed-loop model validation requires high spatiotemporal resolution, but current methods lag:
- Metabolite Detection Delays: LC-MS minute-scale sampling misses second-level metabolic oscillations.
- Single-Cell Tracking Costs: Microfluidic-fluorescence systems for genome-wide monitoring exceed $100,000 per experiment.
IV. Breakthrough Solutions (2024–2025)
Challenge | Innovation | Improvement |
---|---|---|
Parameterization | Microfluidic single-cell mass spectrometry | 1000x throughput, <5% error |
Multiscale Integration | Lattice-Boltzmann fluid-metabolic models | Fermenter flux prediction error ±8% |
Host-Circuit Competition | Orthogonal translation systems (e.g., CFPS) | Decouples heterologous/host metabolism |
Real-Time Monitoring | Quantum dot-dCas9 imaging nanoprobes | Millisecond gene expression resolution |
V. Future Outlook
Synthetic biology kinetics demands three paradigm shifts:
- Digital Twins: Integrate high-throughput data with AI to create dynamic cell factory mirrors.
- Non-Equilibrium Thermodynamics: Quantify energy efficiency via entropy production rates.
- Synthetic-Natural System Theory: Decode engineered/native microbiome interactions to mitigate ecological risks.
Interdisciplinary collaboration—spanning physicists, chemists, and engineers—is critical to shatter the “glass ceiling” of current kinetic modeling and transition from trial-and-error to rational design.
Data sourced from public references. Contact: chuanchuan810@gmail.com.