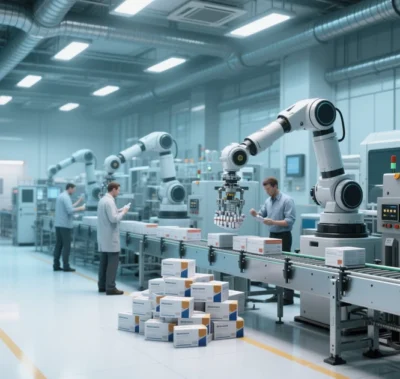
BioAIPharma Applications and Case Studies: AI-Driven Transformation in Biopharmaceuticals
Artificial Intelligence (AI) is revolutionizing biopharmaceutical research and development (BioAIPharma), permeating every stage from target discovery to clinical trials, manufacturing, and precision medicine. Below, we explore how AI reshapes industry paradigms through real-world applications and case studies.
1. Target Identification and Biomarker Discovery
AI accelerates target validation by integrating multi-omics data and disease models:
- AlphaFold2’s Protein Structure Revolution:
Google DeepMind’s AlphaFold2 achieved atomic-level accuracy in protein structure prediction, surpassing traditional methods like cryo-EM.
Case Study: Pfizer used AlphaFold2 to analyze SARS-CoV-2 spike protein conformations, accelerating the optimization of Paxlovid. - Insilico Medicine’s PandaOmics Platform:
Combines multi-omics data (transcriptomics, epigenomics, clinical data) with causal AI to identify disease targets and assess druggability.
Outcome: Discovered a novel target for idiopathic pulmonary fibrosis (IPF) and nominated a preclinical candidate in 18 months (vs. 4–6 years traditionally). - BioAI’s Multimodal Causal AI:
Integrates histopathology images, genomic data, and EHRs to develop digital biomarkers for rapid genetic mutation screening (e.g., EGFR, KRAS).
2. Drug Design and Molecular Optimization
AI-driven generative chemistry and simulations overcome molecular design bottlenecks:
- Relay Therapeutics’ Dynamo™ Platform:
Designs small molecules based on protein dynamic conformations (not static structures), predicting binding pocket changes.
Case Study: RLY-4008, targeting FGFR2 allosteric sites, showed high selectivity in cholangiocarcinoma trials, avoiding off-target toxicity. - Insilico’s Chemistry42:
Uses GANs to generate millions of molecules, optimized via reinforcement learning for ADMET (absorption, distribution, metabolism, excretion, toxicity) properties.
Outcome: Developed the first fully AI-discovered TNIK inhibitor (for renal fibrosis), now in Phase II trials. - Sanofi’s DeepChord Platform:
Leverages graph neural networks (GNNs) to predict drug-target interactions, streamlining ADC linker design and reducing lab iterations.
3. Clinical Trial Optimization and Patient Stratification
AI addresses high costs and delays in clinical trials through predictive analytics:
- HEALWELL AI’s Smart Trial Network:
Integrates global clinical trial data to auto-match patients using NLP, cutting recruitment time by 40%. - Intelligent Medical Objects’ SEETrials:
GPT-4-powered tool extracts safety and efficacy metrics from trial summaries, aiding real-time protocol adjustments (e.g., dose escalation). - Paradigm’s Adaptive Trial Design:
Bayesian models predict patient response rates, boosting Phase III success rates for NSCLC trials to 65% (vs. 50% traditionally).
4. AI in Manufacturing and Commercialization
AI drives Industry 4.0 transformation in biopharma production:
- Modular Bioreactor Control:
Siemens’ AIoT platform monitors cell culture parameters (e.g., oxygen, pH) in real time, increasing monoclonal antibody yields by 30%. - Pfizer’s Supply Chain Risk Prediction:
GNNs model global API supply chains to predict shortages (e.g., lipid nanoparticles during COVID-19), optimizing inventory turnover by 22%.
5. Industry Collaboration and Future Trends
- Cross-Disciplinary Fusion:
- Quantum-AI Synergy: NVIDIA’s BoNeMo Cloud simulates drug-target binding free energy with 50% higher accuracy than traditional methods.
- Spatial Transcriptomics + AI: 10x Genomics and BioAI map tumor microenvironment heterogeneity to enhance CAR-T infiltration.
- Ethical and Equity Challenges:
- Data Bias Mitigation: Roche’s Global Genomic Diversity Initiative uses federated learning to reduce racial bias in target discovery.
- Biosafety: Ginkgo Bioworks engineers temperature-sensitive kill switches for gene-edited microbes to prevent environmental leaks.
Conclusion: Three Paradigm Shifts in BioAIPharma
- From Trial-and-Error to Causal Reasoning: Multimodal AI deciphers disease biology, replacing traditional R&D approaches.
- From Siloed Innovation to Ecosystem Collaboration: Pharma giants (e.g., AstraZeneca, Roche) partner with AI startups (e.g., Insilico, BioAI) to share data and computational resources.
- From Treatment to Prevention: AI-powered digital biomarkers (e.g., BioAI’s H&E slide analysis) enable early screening and precision health management.
Over the next five years, AI could slash drug development costs by 50% and shorten clinical phases to 3–4 years. However, challenges like data standardization, interdisciplinary talent gaps, and ethical governance must be addressed to realize this vision.
Data sourced from public references. For collaboration or domain inquiries, contact: chuanchuan810@gmail.com