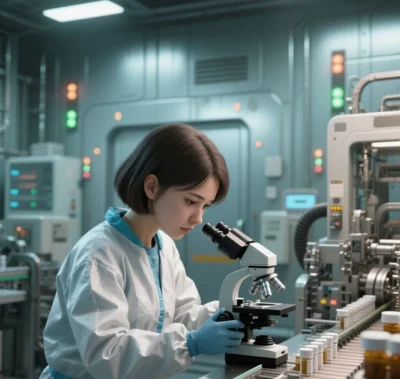
Key Challenges and AI-Driven Solutions in RNA Therapeutics (RNAThera)
RNA therapeutics (RNAThera) face significant challenges in data complexity, target identification, delivery efficiency, and personalized treatment optimization. While existing resources do not explicitly mention RNAThera, innovations in genomic data modeling—such as multi-omics integration and AutoML optimization in tools like Huawei Cloud’s AutoGenome—offer indirect solutions. Below, we analyze these challenges and propose AI-driven strategies.
Key Challenges
- Non-Euclidean Data Complexity
RNAThera relies on integrating genomic, transcriptomic, and epigenomic data, which exhibit non-Euclidean properties (e.g., gene regulatory networks, cross-layer interactions). Traditional neural networks (CNNs/RNNs) struggle to model such relationships.- Solution: AutoGenome’s Residual Fully Connected Network (RFCN) enables cross-layer feature interactions, avoiding information loss in traditional models.
- Target Identification and Biomarker Discovery
Identifying critical RNA molecules (e.g., mRNA, non-coding RNA) in high-dimensional data is hindered by noise.- Case: AutoGenome automatically identifies ribosomal genes in mouse scRNA-seq data, aiding target prioritization.
- Drug Delivery and Efficacy Prediction
RNA molecules are prone to degradation and immunogenicity, requiring genomic insights to optimize delivery systems (e.g., lipid nanoparticles).- Solution: AutoGenome’s drug sensitivity models integrate patient genomic data to refine delivery vehicle design.
- Personalized Monitoring
RNA therapy efficacy varies due to individual genomic differences, necessitating real-time tracking of transcriptional dynamics.- Bottleneck: Traditional methods fail to resolve transient states in single-cell RNA velocity.
AI-Driven Solutions
- Non-Euclidean Data Modeling with RFCN
- Innovations:
- RFCN-ResNet/DenseNet: Residual or dense connections enhance cross-layer regulatory modeling for RNA interaction networks.
- Randomized RFCN (RRFCN): Neural architecture search (NAS) auto-generates optimal structures for diverse RNA tasks.
- Case: RFCN improved breast cancer subtyping accuracy by over 5% by integrating RNA expression and proteomic data.
- Automated Target Screening and Interpretability
- AutoML Integration:
- Bayesian optimization auto-tunes hyperparameters (e.g., learning rates), reducing manual trial-and-error.
- SHAP analysis visualizes key RNA biomarkers (e.g., hydroxymethylation markers in liver cancer) for experimental validation.
- Efficiency: End-to-end modeling in five lines of code accelerates target discovery.
- Multi-Omics Integration and Dynamic Trajectories
- Approaches:
- Single-Cell RNA-seq: AutoGenome identifies cell subpopulations and infers developmental trajectories to predict RNA dynamics.
- Spatial Transcriptomics: MERFISH data models 3D tumor microenvironments to enhance RNA therapy targeting.
- Case: Predicted TGF-β pathway RNA targets in mouse kidney fibrosis studies accelerated anti-fibrotic drug development.
- Personalized Treatment and Real-Time Optimization
- Dynamic Modeling:
- RNA velocity calibration uses metabolic labels (e.g., 4sU) to track RNA expression changes over time.
- Federated learning enables secure cross-institutional data sharing for tailored RNA therapies.
- Clinical Impact: CAS teams used AutoGenome to screen liver cancer RNA methylation markers with 8% higher accuracy than published methods.
Future Challenges and Frontiers
- Data Security and Ethics
- Federated learning and blockchain protect RNA data privacy while enabling collaboration.
- Cross-Modal Integration
- Quantum computing simulates RNA-protein binding energy to optimize drug design.
- Radiogenomics merges MRI/PET imaging with RNA data to predict therapeutic responses.
- Delivery System Optimization
- Automated molecular docking screens RNA carrier materials (e.g., lipid nanoparticles).
- Reinforcement learning models in vivo distribution of delivery vehicles.
Industry Impact
RNAThera advancements rely on data modeling (resolving complexity), automation (lowering barriers), and multi-omics integration (enhancing precision). Huawei Cloud’s AutoGenome drives progress by:
- Democratizing AI: Biologists can build models without coding expertise via five-line workflows.
- Accelerating R&D: Single-cell modeling efficiency improved by 15%, shortening preclinical timelines.
- Enhancing Interpretability: SHAP outputs publication-ready results, fostering academia-industry collaboration.
Looking ahead, the fusion of spatial omics and quantum-AI will revolutionize RNAThera from molecular design to dynamic monitoring.
Data sourced from public references. For collaboration or domain inquiries, contact: chuanchuan810@gmail.com