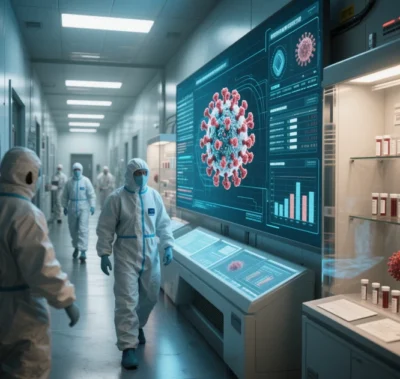
Comparative Analysis of mRNA Velocity and Traditional Single-Cell Transcriptomic Approaches
RNA Velocity is a kinetic model that predicts future transcriptional states of single cells by analyzing the ratio of unspliced to spliced mRNA. Unlike traditional single-cell methods (e.g., pseudotime trajectory analysis, differential expression analysis), it offers distinct advantages in data-driven dynamics, temporal inference, and application scenarios. Below, we compare these approaches across six dimensions:
1. Principles and Model Assumptions
Aspect | RNA Velocity | Traditional Analysis (e.g., Monocle, PAGA) |
---|---|---|
Core Principle | Uses unspliced/spliced mRNA ratios to model transient cell-state changes via differential equations. | Constructs cell trajectories based on gene expression similarity, relying on pseudotime or graph-based algorithms. |
Dynamic Assumptions | Assumes transcription, splicing, and degradation follow kinetic laws (steady-state or dynamic models). | Assumes gene expression changes follow a smooth, continuous trajectory without explicit molecular mechanisms. |
Temporal Resolution | Predicts instantaneous future states (hour-scale changes). | Relies on pseudotime to infer relative positions along a hypothetical timeline. |
Data Requirements | Requires intronic sequencing (unspliced mRNA) and high sequencing depth. | Uses exon-level expression matrices; no need for unspliced mRNA data. |
Case Study: In mouse hippocampal development, RNA Velocity identified early drivers of neurogenesis (e.g., Neurod1) via unspliced mRNA signals, while Monocle required predefined marker genes (e.g., Sox2) for trajectory inference.
2. Technical Advantages
RNA Velocity Strengths:
- Unsupervised Directionality: Predicts differentiation paths without predefined start/end points (e.g., T-cell exhaustion pathways in liver cancer).
- High-Dimensional Integration: Embeds velocity vectors into UMAP/tSNE plots for cross-validation with spatial transcriptomics.
- Transient State Detection: Captures transcriptional bursts and transitional cell states missed by traditional methods.
Traditional Methods:
- Steady-State Systems: Performs well in systems with clear endpoints (e.g., hematopoietic lineages).
- Low-Complexity Data: More robust for datasets with poor unspliced mRNA signals (e.g., low-coverage 10x Genomics data).
3. Computational Models and Challenges
Model Type | Tools | Assumptions | Limitations |
---|---|---|---|
Steady-State Model | velocyto | Constant splicing rates; assumes transcriptional equilibrium. | Fails under dynamic splicing (e.g., rapid gene regulation during inflammation). |
Dynamic Model | scVelo | Gene-specific splicing rates optimized via EM algorithms. | Computationally intensive; requires high-quality data (e.g., full-length RNA). |
Pseudotime Model | Monocle3 | Gene expression follows a single continuous trajectory. | Cannot resolve parallel paths (e.g., T-cell exhaustion vs. memory formation). |
Key Challenges:
- Splicing Kinetics Bias: Steady-state models overestimate directional consistency during rapid differentiation.
- Data Sparsity: Low unspliced mRNA abundance in 10x data increases noise (requires k-nearest neighbor smoothing).
4. Biological Interpretation
RNA Velocity Contributions:
- Driver Gene Discovery: Phase portraits identify key differentiation regulators (e.g., Neurog3 in pancreatic development).
- Temporal Resolution: Distinguishes transcriptionally active cells during G1/S transitions in cell cycle analysis.
Traditional Method Synergy:
- Pathway Enrichment: Cross-validating DEGs with velocity drivers enhances reliability (e.g., renal fibrosis studies).
- Cell Communication: Maps velocity directions to ligand-receptor interaction timelines via tools like CellChat.
5. Limitations and Emerging Solutions
Current Challenges:
- Platform Dependency: Full-length SMART-seq2 data outperforms 10x Genomics for velocity analysis.
- Model Simplifications: Ignores transcriptional noise and alternative polyadenylation.
Innovative Solutions:
- Deep Learning: veloVI integrates variational autoencoders (VAEs) to model expression-velocity uncertainty.
- Spatial Enhancements: MERFISH data infers in situ velocity via nuclear/cytoplasmic mRNA gradients (e.g., brain development).
6. Recommended Applications
Goal | Method | Rationale |
---|---|---|
Early differentiation exploration | RNA Velocity (scVelo) | Captures transient states without trajectory bias. |
Steady-state trajectory modeling | Monocle3 + differential expression | Leverages prior marker genes for coherent paths. |
Multi-omics integration | RNA Velocity + spatial embedding | Validates spatial differentiation hypotheses by mapping velocity vectors. |
Low-quality data analysis | Traditional clustering + DEGs | Avoids noise from sparse unspliced mRNA data. |
Conclusion and Future Directions
RNA Velocity revolutionizes single-cell analysis by modeling mRNA metabolism, yet its accuracy depends on data quality and model assumptions. Future advancements include:
- Multi-omics Integration: Combining ATAC-seq and proteomics to build cross-modal velocity models.
- Temporal Calibration: Using metabolic labels (e.g., 4sU) to convert velocity vectors into real-time scales.
- Noise-Robust Algorithms: Developing graph neural networks to improve signal-to-noise ratios.
Clinically, RNA Velocity predicts cancer immunotherapy response trajectories (e.g., T-cell state shifts post-PD-1 blockade), though wet-lab validation remains critical.
Data sourced from public references. For collaboration or domain inquiries, contact: chuanchuan810@gmail.com