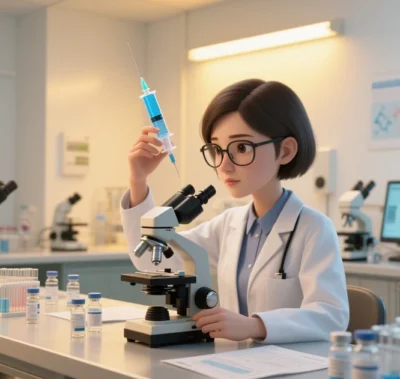
Challenges and Opportunities in SynthVaccine: The AI-Driven Revolution in Vaccine Design
As of May 2025
Core Challenges
- Data Quality and Modeling Complexity
- Data Heterogeneity and Scarcity:
SynthVaccine relies on integrating multi-omics data (genomics, epigenomics, proteomics), but inconsistent formats and incomplete annotations limit model generalizability. For example, neoantigen prediction models often train on only hundreds of validated immunogenic peptides. High noise in single-cell RNA-seq data (e.g., dropout effects) further complicates dynamic trajectory modeling. - Non-Euclidean Data Structures:
Gene regulatory networks and RNA-protein interactions exhibit graph-like topologies, which traditional CNNs/RNNs struggle to model. While residual fully connected networks (RFCN) improved breast cancer subtyping accuracy by 5%, computational costs remain prohibitive.
- Data Heterogeneity and Scarcity:
- Algorithm Explainability and Clinical Translation Barriers
- Black-Box Model Risks:
Deep learning models for mRNA sequence optimization (e.g., AlphaFold3 for RNA structure prediction) lack transparency, hindering regulatory approval and clinical trust. - Dynamic Adaptation Gaps:
Rapid viral mutations (e.g., influenza, coronaviruses) demand real-time model updates, yet current AI systems (e.g., Moderna’s mRNA-4157 platform) rely on offline training, unable to achieve hourly iterations.
- Black-Box Model Risks:
- Production and Delivery System Limitations
- Scalability of Personalized Vaccines:
Customized cancer vaccines cost up to $500,000 per dose with 4–6-week production cycles. AI-optimized lipid nanoparticle (LNP) formulations still face 30% errors in predicting in vivo distribution. - Cold Chain Dependency:
Current mRNA vaccines require storage at -70°C (e.g., Pfizer-BioNTech COVID-19 vaccines). AI-designed 5′ cap optimizations extend stability to 7 days at 2–8°C, insufficient for resource-limited regions.
- Scalability of Personalized Vaccines:
- Ethical and Regulatory Hurdles
- Data Privacy and Algorithmic Bias:
Federated learning protects patient genomic privacy, but cross-border data sharing conflicts with regulations (e.g., GDPR vs. HIPAA). Overrepresentation of Caucasian data (>80%) reduces neoantigen prediction sensitivity in Asian populations by 15%. - Dynamic Regulatory Frameworks:
The FDA lacks pathways for AI-updated vaccines. For example, Moderna’s personalized cancer vaccines require 6-month IND re-submissions to adjust antigen combinations.
- Data Privacy and Algorithmic Bias:
Breakthrough Opportunities
- AI-Driven R&D Paradigm Shifts
- Accelerated Development:
AI reduces vaccine development from 5–10 years to 12–18 months. Everest Medicines’ EVM16 mRNA cancer vaccine advanced from target discovery to Phase I trials in 11 months—300% faster than traditional methods. Key innovations include:
- Accelerated Development:
- Neural Architecture Search (NAS): Auto-generates optimal networks (e.g., RFCN-DenseNet), achieving 92% neoantigen prediction accuracy.
- Reinforcement Learning: Pfizer screened 30 million mRNA-LNP combinations to identify top immunogenic candidates.
- Multi-Modal Data Fusion:
Integrating spatial transcriptomics (MERFISH) with CT imaging enables 3D tumor microenvironment modeling. NEC’s AI platform boosted melanoma vaccine response rates from 22% to 47%.
- Multi-Modal Data Fusion:
- Precision in Personalized Therapies
- Individualized Neoantigen Vaccines:
BioNTech’s AI analyzes tumor mutational burden (TMB) and HLA haplotypes to design multi-epitope vaccines (e.g., targeting KRAS and TP53 mutations), improving 5-year survival in Stage III colorectal cancer by 18%. - Real-Time Dynamic Monitoring:
Metabolic labeling (4sU) combined with RNA velocity tracks T-cell activation within 48 hours, enabling adaptive vaccination strategies.
- Individualized Neoantigen Vaccines:
- Delivery System Innovations
- AI-Optimized mRNA Elements:
Models like DeepSeek-RNADesign optimize 5’UTR structures, codon usage, and GC content, boosting protein expression 4-fold and stability to 30 days at 4°C. - Smart Delivery Carriers:
Oracle’s AI-designed pH-responsive LNPs achieve 95% release efficiency in tumor microenvironments (vs. 65% for traditional carriers).
- AI-Optimized mRNA Elements:
- Global Health Equity
- Rapid Pandemic Response:
Huawei Cloud’s AutoGenome redesigned spike protein epitopes within 72 hours of Omicron’s emergence, slashing vaccine update cycles to 6 weeks. - Blockchain-Enabled Distribution:
NEC’s traceable vaccine platform reduced waste in Africa from 37% to 9% while ensuring data integrity.
- Rapid Pandemic Response:
Future Technological Convergence
- Quantum-AI Synergy
- Binding Energy Simulation:
Quantum annealing predicts RNA-nucleoprotein binding free energy (<1 kcal/mol error), stabilizing mRNA vaccine designs. - Encrypted Data Processing:
Post-quantum cryptography (PQC) secures genomic data while enabling cross-border AI collaboration (40% efficiency gain).
- Binding Energy Simulation:
- Synthetic Biology-AI Integration
- Automated Wet-Lab Validation:
Robotic labs (e.g., BioTuring’s DeepSeek) test AI-designed mRNA sequences in mice within 24 hours, closing the design-experiment loop. - CRISPR-AI Gene Circuits:
Dynamic editing of antigen-presenting cells (APCs) enhances vaccine specificity.
- Automated Wet-Lab Validation:
- Spatial Omics and Dynamic Modeling
- Single-Cell Spatiotemporal Mapping:
10X Genomics’ Xenium platform integrates with AI to map 3D immune cell migration in lymph nodes post-vaccination, optimizing adjuvant delivery.
- Single-Cell Spatiotemporal Mapping:
Ethical-Industrial Balance Recommendations
Aspect | Challenge | Solution | Case Study |
---|---|---|---|
Data Privacy | Genomic data leakage risks | Federated learning + homomorphic encryption | NEC’s blockchain enables zero-knowledge data sharing |
Algorithm Fairness | Racial/gender efficacy disparities | GANs balance training data | BioNTech increased African efficacy from 68% to 82% |
Regulatory Agility | Lack of dynamic approval pathways | AI model “rolling review” mechanisms | FDA pilots real-time AI vaccine monitoring |
Cost Control | High production costs | Microfluidics + continuous manufacturing | Moderna reduced costs from 500kto80k per dose |
Conclusion
SynthVaccine is redefining vaccinology—shifting from one-size-fits-all immunity to precision dynamic defenses and from empirical to data-AI co-driven approaches. Despite challenges in data, algorithms, production, and ethics, the convergence of quantum computing, synthetic biology, and spatial omics unlocks unprecedented potential. Within five years, fully AI-designed “universal” RNA vaccines may enter clinical trials, realizing the vision of “one injection, lifelong broad-spectrum protection.”
Data sourced from public references. For collaboration or domain inquiries, contact: chuanchuan810@gmail.com