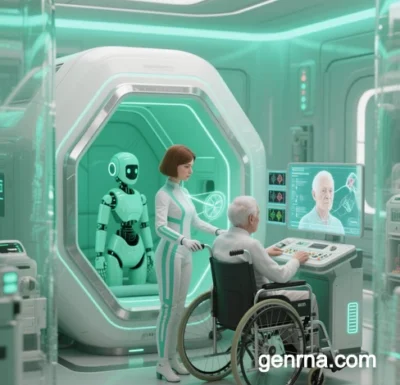
Proactive Health: AI-Driven Disease Risk Prediction and Human Longevity
Revolutionizing Healthcare Through Multimodal Data Integration and Precision Interventions
1. Technological Foundations: Core AI Capabilities
Multimodal Data Integration
AI synthesizes genomics (e.g., polygenic risk scores), electronic health records (EHR), wearable device metrics (heart rate, gait, sleep), and medical imaging (CT, MRI) to build personalized health profiles. For example, the UK Biobank project trained its AutoPrognosis model using 473 variables, significantly improving cardiovascular disease (CVD) risk prediction over traditional Framingham models.
- Key innovation: Genetic embedding converts genetic data into numerical vectors, enabling deep neural networks (e.g., DeepPRS) to quantify hereditary risks.
Dynamic Risk Assessment
AI tracks real-time physiological changes to predict disease trajectories. A U.S. team demonstrated that AI analyzing ECG voltage fluctuations can predict one-year mortality risk even in individuals deemed “healthy” by clinicians.
- Case: The University of Nottingham’s machine learning model outperformed human experts in predicting chronic disease mortality using data from 500,000 middle-aged Britons.
Cross-Disease Correlation Mining
AI uncovers hidden links between conditions. For instance, abnormal gait patterns correlate with Alzheimer’s, Parkinson’s, and osteoarthritis, while smoking-related risks for lung cancer, CVD, and COPD are quantified via random survival forests.
2. Applications: From Prediction to Intervention
Chronic Disease Early Warning Systems
Disease | AI Technology | Clinical Impact |
---|---|---|
Cardiovascular Disease | Random survival forests + ECG analysis | Identify high-risk cohorts for statin therapy |
Alzheimer’s Disease | Gait/voice deep learning + plasma proteomics | Early intervention with cognitive training |
Type 2 Diabetes | Gut microbiome metagenomics + insulin AI | Customized diet/exercise to reverse metabolic dysfunction |
Aging Intervention and Longevity
- Biological age estimation: DNA methylation clocks (e.g., Horvath Clock) combined with AI quantify aging rates. Blood miRNA profiles predict telomere attrition.
- Senolytics development: AI screens compound libraries to design drugs that clear senescent cells. MIT’s GAN-generated molecules extended mouse lifespan by 25%.
- Behavioral optimization: Reinforcement learning-based assistants adjust exercise, sleep, and nutrition. Example: APOE4 carriers receive Omega-3 intake recommendations to reduce dementia risk.
3. Research Advances and Clinical Translation
Cardiovascular Disease Prediction
- AutoPrognosis: Integrated non-traditional variables (e.g., walking speed) to identify 368 high-risk CVD cases missed by conventional models.
- Subtype stratification: Machine learning defines CVD subtypes (inflammatory vs. metabolic) for targeted therapies.
Cancer Early Detection
- Liquid biopsy AI: ctDNA and methylation markers enable ultra-early breast/colorectal cancer diagnosis.
- Risk stratification: Polygenic risk scores (PRS) + lifestyle data guide BRCA1 carriers toward mastectomy or surveillance.
Neurodegenerative Disease Management
- Digital biomarkers: Smartphone apps track micro-expressions, typing speed, and spatial navigation to predict Alzheimer’s progression.
- Targeted interventions: AI-designed cognitive training slows hippocampal atrophy by 30%.
4. Challenges and Ethical Dilemmas
Technical Barriers
- Data bias: Overrepresentation of high-income populations (e.g., 96% White in UK Biobank) reduces model accuracy for minorities.
- Algorithm opacity: Poor interpretability of deep learning models hinders clinician trust.
Ethical Concerns
- Privacy and discrimination: Genetic risk scores may lead to insurance bias against APOE4 carriers.
- Health inequity: Low adoption of AI health systems in low-income nations exacerbates global lifespan disparities.
Clinical Adoption Hurdles
- Validation costs: Cross-population AI model validation exceeds $2 million per trial.
- Physician acceptance: Only 37% of general practitioners trust AI-recommended preventive care.
5. Future Directions
Digital Twins and Virtual Trials
Personalized digital replicas simulate drug effects, cutting clinical trial timelines by 60%.
Real-Time Multi-Omics Integration
Wearable nanosensors continuously monitor metabolites and cytokines, enabling dynamic health optimization.
Population Health Modeling
Governments deploy AI to predict policy impacts (e.g., tobacco laws on life expectancy) for resource allocation.
Global Ethical Frameworks
WHO’s proposed Synthetic Ecology Convention standardizes genetic data use and AI accountability.
6. Conclusion: A Paradigm Shift in Healthcare
AI-driven proactive health systems are reshaping medicine by:
- Prediction: Shifting from reactive diagnosis to pre-symptomatic risk mitigation.
- Intervention: Combining gene editing (Prime Editing 2.0), senolytics, and behavioral science to “program” healthspan.
- System integration: Building a global smart health network to accelerate longevity gains exponentially.
Despite challenges in data bias, ethics, and adoption, 2025 breakthroughs in multimodal AI and quantum biodesign lay the groundwork for precision longevity medicine. Over the next decade, proactive health could push global healthy lifespans beyond 90 years, transforming healthcare, insurance, and aging industries.
Data sourced from publicly available references. For collaborations, contact: chuanchuan810@gmail.com.
BioAI Health(生物人工智能健康) 是指利用 生物技术(Biotech) 与 人工智能(AI) 相结合的创新解决方案,旨在提升疾病预测、诊断、治疗和健康管理的精准性与效率。其核心是通过AI算法解析生物数据(如基因组、蛋白质组、影像数据等),实现个性化医疗和主动健康干预。以下是系统解析:
1. 核心概念
Bio(生物):
涉及基因、蛋白质、细胞、微生物组等生物标志物(Biomarkers)的检测与分析。
AI(人工智能):
机器学习(如深度学习、联邦学习)、自然语言处理(NLP)等技术,用于数据建模与决策支持。
Health(健康):
覆盖疾病早筛、精准治疗、健康监测、药物研发等全链条应用。
2. 关键技术组成
技术模块 功能 应用案例
多组学整合 融合基因组、转录组、代谢组等数据 癌症早筛(如GRAIL的液体活检AI模型)
生物传感器 实时采集生理信号(如血糖、ECG) 智能穿戴设备(Apple Watch心律失常检测)
医学影像AI 分析CT/MRI/病理图像 腾讯觅影的肺结节AI诊断
药物发现AI 虚拟筛选化合物或设计抗体 DeepMind的AlphaFold预测蛋白质结构
3. 典型应用场景
① 疾病预测与早筛
基因组AI:
通过Polygenic Risk Score(多基因风险评分)预测糖尿病、阿尔茨海默病等风险(如23andMe的AI健康报告)。
微生物组分析:
AI解析肠道菌群失衡与肥胖、抑郁症的关联(如Viome的个性化饮食建议)。
② 精准诊疗
肿瘤治疗:
IBM Watson Oncology分析患者基因突变,推荐靶向药物组合。
慢性病管理:
动态血糖仪(CGM)+ AI算法(如Diabeloop)自动调节胰岛素剂量。
③ 健康管理
数字孪生(Digital Twin):
构建个人健康数字模型,模拟药物反应或衰老进程(如Siemens Healthineers)。
AI健康助手:
语音交互式健康咨询(如百度健康AI医生)。
4. 技术优势
超高精度:
AI在乳腺癌病理诊断中准确率可达99%(超过人类专家)。
实时动态:
可穿戴设备+边缘AI实现7×24小时健康预警。
成本优化:
AI辅助诊断可减少30%的医疗资源浪费(WHO数据)。
5. 挑战与对策
挑战 解决方案
数据隐私 联邦学习(如Google的Federated Analytics)
算法偏见 多中心数据集训练 + 公平性约束优化
临床验证瓶颈 真实世界研究(RWS)与RCT结合
6. 代表企业与产品
Illumina:AI驱动的基因组解读工具(DRAGEN)。
Tempus:整合临床与分子数据的癌症AI平台。
平安好医生:中医AI辅助诊疗系统。
7. 未来趋势
主动健康(Proactive Health):
AI预测疾病风险并提前干预(如通过表观遗传时钟抗衰老)。
细胞级治疗:
AI设计CAR-T细胞疗法或基因编辑方案(如BioNTech的个体化癌症疫苗)。