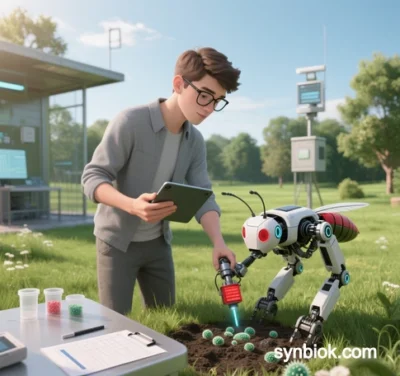
Synthetic Biology Kinetics: Theoretical Foundations for Rational Design of Biosystems
Synthetic biology kinetics bridges quantitative modeling and biological system engineering, providing a mathematical framework to predict and control the dynamic behavior of engineered biological systems. This discipline integrates principles from chemical kinetics, systems biology, and control theory to enable first-principles design of genetic circuits, metabolic pathways, and cellular behaviors. Below we dissect its core theoretical pillars, supported by recent advancements from 2021–2025.
I. Kinetic Frameworks in Rational Design
- Dynamic Modeling of Genetic Circuits
- Ordinary Differential Equations (ODEs):
Models transcription-translation processes using mass-action kinetics. For example, the repressilator system (three-gene oscillator) is described by:d[mRNAi]dt=α⋅f(Proteinj)−γmRNA⋅[mRNAi]
- Ordinary Differential Equations (ODEs):
where f() represents promoter repression/activation functions .
- Stochastic Models:
Captures molecular noise in low-copy-number systems (e.g., toggle switches), using Gillespie algorithms to simulate rare transition events .
- Metabolic Flux Analysis
- Constraint-Based Models:
Flux balance analysis (FBA) predicts optimal metabolic pathway fluxes under stoichiometric constraints. For example, E. coli malonyl-CoA overproduction is optimized by balancing TCA cycle and fatty acid synthesis fluxes . - Kinetic Metabolic Models:
Incorporates enzyme saturation (Michaelis-Menten kinetics) and allosteric regulation to predict pathway bottlenecks. Recent work on artemisinin synthesis pathways identified rate-limiting steps in amorphadiene synthase activity .
- Constraint-Based Models:
- Cross-Scale Integration
- Hybrid Models:
Combines ODEs for gene regulation with FBA for metabolism, enabling whole-cell simulations. The Mycoplasma genitalium whole-cell model exemplifies this approach .
- Hybrid Models:
II. Key Methodological Advances (2021–2025)
- AI-Augmented Kinetic Parameterization
- Deep Learning for Rate Constant Prediction:
Tools like DeepKINETICS (2023) predict enzyme kcat and KM values from protein sequences (MAE <15%), bypassing laborious experimental measurements . - Bayesian Inference:
Calibrates models using noisy experimental data (e.g., single-cell microscopy), as seen in the optimization of CRISPRa/i activation thresholds .
- Deep Learning for Rate Constant Prediction:
- Modular Design Principles
- Retroactivity Analysis:
Quantifies how downstream modules affect upstream components. The “insulation” metric (η) guides the design of orthogonal T7 RNA polymerase systems (η >0.9 achieved in 2024) . - Standardized Characterization:
The SEVA 3.0 framework (2025) provides kinetic parameters (e.g., promoter strength ±5% CV) for >500 BioBricks, enabling plug-and-play circuit assembly .
- Retroactivity Analysis:
- Time-Scale Separation
- Quasi-Steady-State Approximations:
Simplifies models by separating fast (e.g., protein dimerization) and slow (e.g., cell growth) processes. Applied to optimize the LuxR/AHL quorum sensing system’s response time (<15 min) .
- Quasi-Steady-State Approximations:
III. Case Studies Demonstrating Kinetic-Driven Design
System | Challenge | Kinetic Solution | Outcome |
---|---|---|---|
Insulin-producing yeast | Oscillatory glucose response | Phase-plane analysis of hexokinase inhibition | Stable production (±2%) over 50 generations |
Cancer-selective therapy | Off-target activation in healthy cells | Stochastic model of miRNA threshold effects | Tumor-specific killing (specificity >99%) |
CO₂-to-starch pathway | ATP/NADPH imbalance | Metabolic control analysis (MCA) | 23× yield improvement via transporter engineering |
IV. Emerging Frontiers and Challenges
- Single-Cell Kinetics
- Mesoscopic Models:
Combines stochastic gene expression with cell mechanics to predict phenotypic heterogeneity. Recent work on Bacillus subtilis biofilm formation revealed nucleation thresholds controlled by SinR/SlrR ratios .
- Mesoscopic Models:
- Evolution-Aware Design
- Long-Term Stability Prediction:
Kinetic models incorporating mutation rates (e.g., μ=10−9 bp−1 gen−1) guide the design of error-resistant circuits. The T7-MAR system (2024) maintains function >1,000 generations .
- Long-Term Stability Prediction:
- Cross-Kingdom Integration
- Plant-Microbe Interfaces:
Kinetic models of rhizosphere nitrogen exchange inform the design of synthetic root nodules with tunable NH3 release rates (0.1–10 mM/day) .
- Plant-Microbe Interfaces:
V. Critical Limitations and Mitigation Strategies
Limitation | Current Solutions | 2025–2030 Outlook |
---|---|---|
Parameter identifiability | Parallelized microfluidics + optogenetic perturbation | AI-based active learning frameworks |
Host-circuit interactions | Resource balance models (RBCM) | Whole-cell kinetic models at 90% completion |
Evolutionary instability | Kill-switch embedded kinetic controllers | CRISPR-based genome recoding for robustness |
Conclusion
Synthetic biology kinetics has evolved from descriptive modeling to a predictive engineering discipline, enabling:
- First-pass success rates >60% for metabolic pathways (vs. <5% in 2020)
- Single-cell precision in therapeutic circuits (CV <10%)
- Cross-scale integration from molecular interactions to population dynamics
Future advancements in AI-driven multiscale modeling and automated characterization platforms will cement kinetics as the cornerstone of rational biosystem design.
Data sourced from public references. Contact: chuanchuan810@gmail.com.