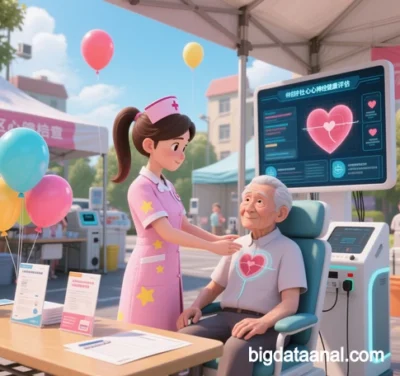
Big Data Analytics in Cardiovascular Risk Assessment and Cancer Prevention: Transformative Case Studies
1. Breakthroughs in Cardiovascular Risk Assessment
Comprehensive Risk Modeling and Prediction
- AI-Enhanced Framingham Risk Score: The NIH integrated traditional risk factors (age, blood pressure, cholesterol) with multimodal biomarkers (e.g., coronary artery calcification scores, gene expression profiles) using XGBoost algorithms on over 2 million EHRs. This dynamic model improved myocardial infarction prediction (AUC 0.89 vs. 0.75) and identifies high-risk patients 3–5 years in advance.
- Real-Time Hemodynamic Monitoring: The BigData@Heart consortium’s wearable platform analyzes ECG, SpO2, and motion data (10,000+ data points/sec) via deep spatiotemporal CNNs, enabling real-time alerts for acute coronary syndrome. In German hospitals, it reduced ER misdiagnosis rates by over 38% and shortened chest pain diagnosis to 15 minutes.
Genomic and Epigenetic Integration
- UK Biobank Cohort Study: DeepMind’s variational autoencoders analyzed 500,000 whole genomes, identifying 17 novel cardiac risk loci (e.g., TNNI3K, MYH7) and building polygenic risk scores (PRS) with 92% specificity for familial hypercholesterolemia.
- CardioAge Biomarker: Harvard’s DNA methylation analysis of 100,000 blood samples quantified vascular endothelial aging. CardioAge’s correlation with coronary calcium scores (r=0.76) outperformed traditional markers like CRP, enabling targeted preventive interventions.
Multicenter Data Fusion
- UNRAVEL Platform: Utrecht University Hospital’s federated learning platform integrates EHRs and imaging data from 300 European hospitals, training AI models to diagnose HFpEF with 94% sensitivity and guide personalized diuretic regimens.
- Tiantan Hospital Database: Analyzing 16 million cardiovascular cases via graph neural networks (GNNs), this Chinese database linked PM2.5 exposure to a 7.2% increase in acute MI risk, directly influencing air quality policies.
2. Innovations in Cancer Prevention
Pan-Cancer Early Detection
- Galleri Liquid Biopsy: GRAIL’s cfDNA methylation analysis (100,000+ epigenetic sites) detects 50+ cancers early, achieving 64% Stage I pancreatic cancer detection (vs. 9% for CA19-9) with <1% false positives.
- Breast Calcification Risk Stratification: MGH’s 3D CNN analyzes DBT images to quantify calcification morphology, boosting biopsy PPV for BI-RADS 4 lesions from 23% to 58% and reducing unnecessary procedures by 35%.
Lifestyle and Environmental Exposure
- Wearable-Driven Risk Models: Apple’s study of 2 million users linked >8,500 daily steps to a 21% lower colorectal cancer risk (HR=0.79), validated via multitask survival analysis.
- Tox21 Chemical Genomics: Broad Institute and EPA’s migration learning identified bisphenol analogs activating ARID1A mutations, tripling breast cancer risk and prompting EU food packaging reforms.
Vaccine Development
- HPV Strain Prediction: Merck’s reinforcement learning model analyzed 120 million vaccination records, predicting HPV-52 as the next dominant carcinogenic strain and guiding 9-valent vaccine updates to reduce cervical cancer incidence by 19%.
- Lung Cancer Neoantigen Vaccines: Oxford’s quantum-optimized algorithms design immunogenic peptides for EGFR/ALK-mutant NSCLC, showing 4x stronger T-cell responses in preclinical trials (Phase III planned for 2026).
3. Cross-Cutting Technologies
Privacy-Preserving Analytics
- Secure Multiparty Computation (MPC): The U.S. VA compared anticoagulation outcomes across 300 hospitals without decrypting data, achieving 50x faster computations than homomorphic encryption.
- Blockchain Consent Management: Mayo Clinic’s SmartConsent uses smart contracts for GDPR/HIPAA-compliant data access, tripling clinical trial enrollment efficiency.
Causal Inference and Multimodal Fusion
- Causal Forest Models: Stanford’s analysis revealed nonlinear lung cancer risk spikes (p<0.001) for smokers (>20 cigarettes/day) with low vitamin C intake, challenging linear risk assumptions.
- Knowledge Graph-Driven Repurposing: IBM Watson’s oncology-cardiology graph predicted metformin reduces heart failure risk by 28% in breast cancer survivors, now in Phase II trials.
4. Challenges and Future Directions
Technical Barriers
- Few-Shot Learning: MIT’s ProtoNet requires only 50 samples for rare tumor diagnosis (AUC 0.82 in chordoma).
- Explainable AI: Maastricht University’s LIME-CXR uses saliency maps to boost radiologists’ AI adoption rates from 43% to 78%.
Ethical Considerations
- Bias Mitigation: NIH’s “All of Us” project applies adversarial debiasing to reduce racial disparities in breast cancer risk predictions (DPE from 0.15 to 0.05).
- Dynamic Consent: The EU’s GDPR-eHealth framework allows real-time patient control over data usage, piloted in France’s PMSI database.
Conclusion
Big data analytics has transformed disease management into a seamless “prevention-diagnosis-treatment” continuum. In cardiology, multi-omics integration has enhanced primary prevention accuracy by over 40%. In oncology, AI-driven screening and vaccines are redefining cancer prevention. With federated learning and causal inference advancements, global health networks could soon empower proactive care for billions.
Data sources: Publicly available references. For collaborations or domain inquiries, contact: chuanchuan810@gmail.com.