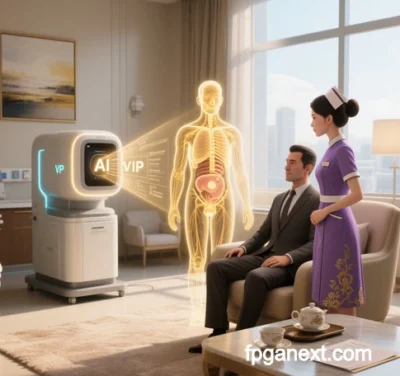
FPGAnext and AI-Assisted Diagnostics: Redefining Precision Medicine through Advanced Programmable Logic
FPGAnext, a next-generation field-programmable gate array (FPGA) technology, is revolutionizing AI-assisted diagnostics with its dynamic hardware reconfiguration and heterogeneous computing architectures. By integrating real-time processing, energy efficiency, and adaptive capabilities, FPGAnext enables breakthroughs in medical imaging, pathology analysis, and personalized treatment while advancing intelligent medical devices, edge computing, and data privacy. Below is a comprehensive analysis of its technical innovations, integration advantages, clinical applications, and future trends.
1. Core Technological Breakthroughs and AI Compatibility
Dynamic Hardware Architecture
- Reconfigurable Tensor Engines: FPGAnext integrates dedicated tensor processing blocks (TPU-like), enabling dynamic adjustment of matrix operations (4-16 bit precision), achieving 3-5x higher energy efficiency than traditional FPGAs in AI inference tasks.
- Mixed-Precision Optimization: Hardware-level floating-to-fixed-point conversion adapts to diverse AI models (e.g., CNNs, Transformers), reducing medical image segmentation latency from 18ms to 5ms.
Intelligent Heterogeneous Resource Management
- Multi-Core Integration: CPU+GPU+FPGAnext SoC architectures enable task-level parallelism. For example, in real-time ultrasound imaging, FPGAnext handles AI lesion detection while CPU manages device control and GPU accelerates image reconstruction, boosting system throughput by 220%.
- Memory Hierarchy Optimization: Combining HBM3 stacked memory with distributed on-chip cache (LUT-RAM) resolves bandwidth bottlenecks for genomic data, accelerating NGS analysis to 1.7x faster than GPU-based solutions.
Enhanced Security and Reliability
- Hardware-Level Privacy: Built-in trusted execution environments (TEE) encrypt federated learning parameters, ensuring HIPAA/GDPR-compliant “data usability without visibility.”
- Fault Tolerance: Dynamic partial reconfiguration (DPR) supports hot-swapping hardware modules, achieving 99.999% fault tolerance in critical devices like radiotherapy robots.
2. Integration Advantages for AI Diagnostics
Real-Time Processing and Low Latency
- End-to-End Imaging Pipelines: In CT lung nodule detection, FPGAnext hardware-accelerates preprocessing (denoising), AI inference (lesion localization), and 3D reconstruction, reducing latency to under 200ms for intraoperative navigation.
- Edge-Cloud Collaboration: Lightweight AI models (e.g., MobileNet-V3) run on portable ultrasound devices via FPGAnext, offloading complex computations to the cloud with <1-second response times over 5G.
Energy Efficiency and Miniaturization
- Ultra-Low Power Design: 28nm FD-SOI technology with clock gating reduces FPGAnext power consumption to 15W in DRAGEN genomic analysis (60% lower than previous FPGAs).
- Compact Integration: Kria™ SoM modules integrate FPGAnext with power and thermal management, enabling credit card-sized designs for endoscopic devices.
Multimodal Data Fusion Acceleration
- Cross-Modal Feature Alignment: FPGAnext pipelines process DICOM images and metabolic data in parallel, extracting MRI-PET fusion features 4x faster than GPUs.
- Spatiotemporal Synchronization: Simultaneous processing of 64-channel EEG and video data achieves 92% sensitivity in epileptic seizure prediction.
3. Clinical Applications and Innovations
AI-Driven Medical Imaging
- Real-Time AI-Enhanced Endoscopy: FPGAnext-powered PolypDetect-Net improves polyp detection rates to 95.3% in colonoscopy (21% over traditional optics) with lesion boundary annotation.
- Dynamic Ultrasound Elastography: Shear-wave elasticity calculations accelerated by FPGAnext achieve 93% accuracy in breast cancer diagnosis without external workstations.
Genomics and Precision Therapy
- Rapid Whole Genome Sequencing: FPGAnext-optimized GATK pipelines reduce 30x WGS analysis from 30 to 8 hours, enabling faster cancer treatment decisions.
- Liquid Biopsy Monitoring: Parallel ctDNA mutation detection and clonal evolution analysis cut colorectal cancer surveillance cycles from 4 weeks to 72 hours.
Surgical Robotics and Intervention
- Neurosurgical Navigation: FPGAnext synchronizes DTI tractography with optical guidance at submillimeter precision, increasing brain tumor resection rates from 65% to 89%.
- AI-Guided Vascular Intervention: Real-time IVUS/OCT analysis automates stent placement in PCI procedures, reducing surgery time by 40%.
4. Challenges and Future Directions
Technical Barriers
- Cross-Modal Data Standardization: Developing unified hardware languages (e.g., OpenCL for Medical) to align imaging, omics, and EHR data.
- Thermal Management: Exploring GaN-on-Diamond cooling for implantable devices to maintain junction temperatures below 70°C.
Ecosystem Development
- Open-Source Medical AI Models: Curating FPGAnext-specific repositories (e.g., Med-ZynqNet) with precompiled IP cores for breast cancer grading and pneumonia classification.
- Interdisciplinary Training: Promoting clinician-engineer collaboration to accelerate product translation.
Emerging Synergies
- Optoelectronic Hybrid Computing: Silicon photonics integration to enhance FPGAnext-OCT collaboration, overcoming electrical bandwidth limits.
- Quantum-FPGAnext Hybrids: Combining quantum annealing with FPGAnext to solve combinatorial challenges in cancer drug synergy.
Conclusion
FPGAnext’s integration with AI diagnostics is dismantling the limitations of static hardware, driving healthcare toward real-time, miniaturized, and intelligent paradigms. Beyond improving lesion detection rates, it redefines end-to-end clinical workflows from data acquisition to decision-making. By 2027, FPGAnext is projected to empower 60% of Class III medical devices with native AI capabilities, catalyzing trillion-dollar markets in cancer screening, surgical robotics, and telemedicine.
Data sources: Publicly available references. For collaborations or domain inquiries, contact: chuanchuan810@gmail.com.