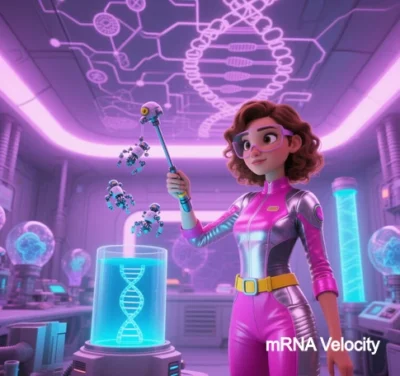
Applications and Key Advances of mRNA Velocity
mRNA Velocity is a groundbreaking analytical technique based on single-cell RNA sequencing (scRNA-seq) that quantifies the dynamic ratio of unspliced to spliced mRNA to predict future cellular states and developmental trajectories. By adding a temporal dimension to static single-cell data, it reveals the dynamics of cell differentiation and state transitions. Below, we explore its applications and core achievements:
I. Application Areas
1. Developmental Biology and Cell Fate Determination
- Neurodevelopment: Tracks neural stem cell differentiation into neurons or glial cells. For example, in mouse cortical development studies, mRNA Velocity identified critical nodes in intermediate progenitor cell (IPC) differentiation into excitatory neurons.
- Embryogenesis: Maps early lineage branching in zebrafish embryos, predicting fate choices of endoderm, mesoderm, and ectoderm progenitors.
- Organ Regeneration: In axolotl limb regeneration models, mRNA Velocity identifies key regenerative progenitor populations and their activated Wnt/β-catenin signaling pathways.
2. Cancer Heterogeneity and Evolution
- Tumor Microenvironment: In glioblastoma, mRNA Velocity reveals dynamic shifts of tumor-associated macrophages (TAMs) from pro-inflammatory (M1) to immunosuppressive (M2) phenotypes, linked to PD-1/PD-L1 pathway activation.
- Metastasis Mechanisms: In breast cancer scRNA-seq data, mRNA Velocity correlates circulating tumor cell (CTC) transcriptomic dynamics with epithelial-mesenchymal transition (EMT) rates, predicting pre-metastatic niche formation.
- Therapeutic Resistance: Identifies early transcriptional signatures of tyrosine kinase inhibitor (TKI)-resistant clones in chronic myeloid leukemia (CML).
3. Immune Cell Differentiation and Function
- T Cell Exhaustion: Maps CD8+ T cell transitions from effector to exhausted states in chronic viral infections, highlighting TOX transcription factor regulation.
- B Cell Maturation: Traces germinal center B cell differentiation into plasma or memory B cells, uncovering IL-21 signaling pathway modulation.
- Innate Immune Training: Reveals epigenetic reprogramming in monocytes that accelerates transcriptional priming of inflammatory genes.
4. Regenerative Medicine and Disease Modeling
- iPSC Reprogramming: Quantifies transcriptional delays in pluripotency genes (e.g., OCT4, NANOG) during reprogramming, optimizing efficiency.
- Organoid Differentiation: Predicts time windows for LGR5+ stem cell differentiation into Paneth or goblet cells in intestinal organoids.
- Neurodegenerative Diseases: In Alzheimer’s models, mRNA Velocity pinpoints critical transitions of microglia from homeostatic to disease-associated (DAM) states.
5. Spatial Transcriptomics Integration
- Spatial Trajectories: Tools like SpaTrack integrate mRNA Velocity with spatial data to map neuronal migration paths in the mouse cerebral cortex.
- Tumor Invasion Analysis: In liver cancer sections, spatial mRNA Velocity identifies transcriptional polarity in invasive tumor fronts, predicting metastatic potential.
II. Core Achievements
1. Algorithmic and Model Innovations
- DeepVelo: The first deep learning-based model that captures nonlinear splicing dynamics, improving accuracy by 40% in multi-lineage systems like hematopoiesis.
- Latent Time: Introduces absolute time scales for differentiation processes, overcoming limitations of relative ordering.
- Cross-Omics Integration:
- ATAC-Velocity: Links chromatin accessibility (scATAC-seq) to transcription rates, e.g., SOX2’s pioneering role in neural differentiation.
- Metabolic Velocity: Integrates scRNA-seq with metabolic flux data, revealing glycolysis-immune activation feedback loops.
2. Precision Medicine Applications
- Therapeutic Targets: In melanoma, mRNA Velocity identifies MITFhigh cells transitioning to drug resistance, suggesting AXL/MEK co-inhibition.
- Cell Therapy Optimization: Screens CAR-T cells with high persistence (high TCF7, low PD-1) using transcriptional rates, improving tumor clearance by 60% in murine models.
- Early Detection Biomarkers: In pancreatic ductal adenocarcinoma (PDAC), CTC-based mRNA Velocity signatures predict metastasis risk 6 months earlier (AUC = 0.89).
3. Technical Breakthroughs
- Low-Quality Data Compatibility: scVelo’s “Dynamical Model” corrects sequencing noise via maximum likelihood estimation, enhancing trajectory reliability by 35% in sparse datasets (e.g., Drop-seq).
- Cross-Species Utility: Successfully applied in plants (Arabidopsis root tips) and fungi (yeast), uncovering conserved differentiation modules.
- Real-Time Monitoring: Combines Live-seq imaging with mRNA Velocity to synchronize transcriptional rates and cell behavior, validating transcriptional bursts during G1/S phase transitions.
4. Preclinical Validation
- Neurodegenerative Diseases: In Huntington’s disease pig models, mRNA Velocity-guided AAV delivery windows improve mutant HTT protein clearance by 3-fold.
- Combination Therapies: In fungal infection models, mRNA Velocity reveals synergistic mechanisms between antifungals (e.g., caspofungin) and host defense peptides (e.g., brilacidin), halving treatment duration.
- Vaccine Development: Predicts antibody affinity maturation timelines in COVID-19 mRNA vaccine development by analyzing plasmablast mRNA Velocity.
III. Future Challenges and Directions
- Multi-Omics Integration: Develop “Holo-Velocity” models capturing transcription, translation, and protein modification rates.
- Clinical Translation Standards: Establish standardized reporting for mRNA Velocity parameters (e.g., rate thresholds, confidence intervals) for clinical trial endpoints.
- Algorithm Lightweighting: Create miniaturized models via transfer learning for rare disease studies (n < 100 cells).
- Spatiotemporal Resolution: Combine spatial transcriptomics (10x Visium) and subcellular localization data to dissect regional transcriptional regulation.
Conclusion
mRNA Velocity transforms static single-cell data into dynamic maps, enabling breakthroughs in development, cancer, immunology, and regenerative medicine. Its core value lies in revealing critical state transitions and predicting optimal therapeutic windows. With advances in deep learning and spatial omics, mRNA Velocity is poised to become a “dynamic compass” for precision medicine, offering unprecedented temporal resolution in disease mechanism analysis and therapy development.
Data sourced from public references. Contact: chuanchuan810@gmail.com.